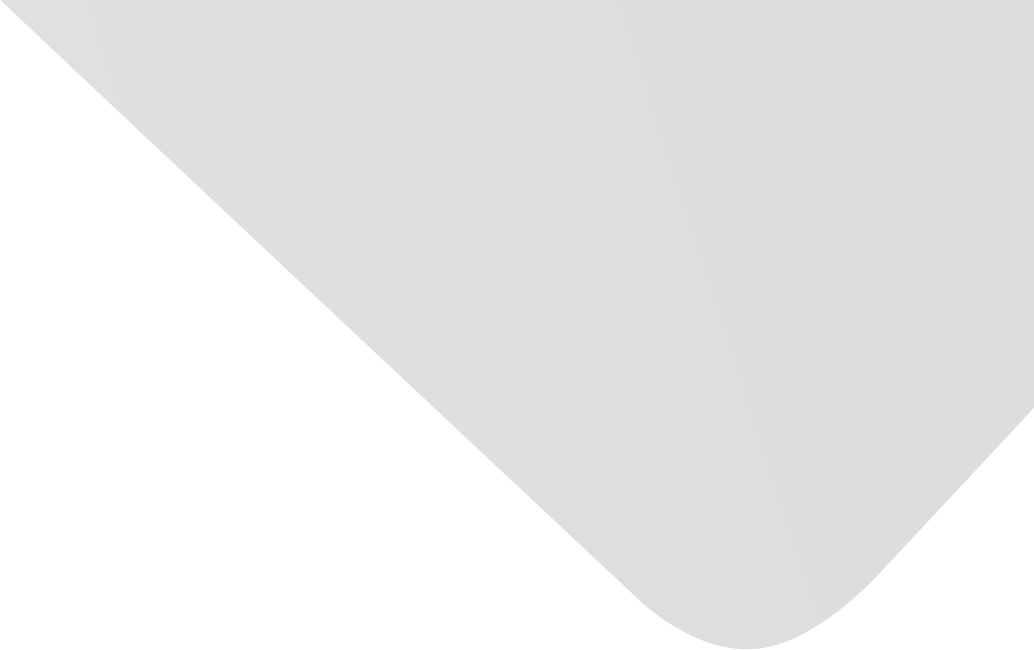
Prediction of “Aggregation-Prone” Peptides with Hybrid Classification Approach
Joint Authors
Wang, Jia-Nan
Zhang, Wen-yi
Jia, Longjia
Liu, Bo
Yin, Minghao
Zhao, Xiaowei
Source
Mathematical Problems in Engineering
Issue
Vol. 2015, Issue 2015 (31 Dec. 2015), pp.1-9, 9 p.
Publisher
Hindawi Publishing Corporation
Publication Date
2015-03-05
Country of Publication
Egypt
No. of Pages
9
Main Subjects
Abstract EN
Protein aggregation is a biological phenomenon caused by misfolding proteins aggregation and is associated with a wide variety of diseases, such as Alzheimer’s, Parkinson’s, and prion diseases.
Many studies indicate that protein aggregation is mediated by short “aggregation-prone” peptide segments.
Thus, the prediction of aggregation-prone sites plays a crucial role in the research of drug targets.
Compared with the labor-intensive and time-consuming experiment approaches, the computational prediction of aggregation-prone sites is much desirable due to their convenience and high efficiency.
In this study, we introduce two computational approaches Aggre_Easy and Aggre_Balance for predicting aggregation residues from the sequence information; here, the protein samples are represented by the composition of k-spaced amino acid pairs (CKSAAP).
And we use the hybrid classification approach to predict aggregation-prone residues, which integrates the naïve Bayes classification to reduce the number of features, and two undersampling approaches EasyEnsemble and BalanceCascade to deal with samples imbalance problem.
The Aggre_Easy achieves a promising performance with a sensitivity of 79.47%, a specificity of 80.70% and a MCC of 0.42; the sensitivity, specificity, and MCC of Aggre_Balance reach 70.32%, 80.70% and 0.42.
Experimental results show that the performance of Aggre_Easy and Aggre_Balance predictor is better than several other state-of-the-art predictors.
A user-friendly web server is built for prediction of aggregation-prone which is freely accessible to public at the website.
American Psychological Association (APA)
Liu, Bo& Zhang, Wen-yi& Jia, Longjia& Wang, Jia-Nan& Zhao, Xiaowei& Yin, Minghao. 2015. Prediction of “Aggregation-Prone” Peptides with Hybrid Classification Approach. Mathematical Problems in Engineering،Vol. 2015, no. 2015, pp.1-9.
https://search.emarefa.net/detail/BIM-1074907
Modern Language Association (MLA)
Liu, Bo…[et al.]. Prediction of “Aggregation-Prone” Peptides with Hybrid Classification Approach. Mathematical Problems in Engineering No. 2015 (2015), pp.1-9.
https://search.emarefa.net/detail/BIM-1074907
American Medical Association (AMA)
Liu, Bo& Zhang, Wen-yi& Jia, Longjia& Wang, Jia-Nan& Zhao, Xiaowei& Yin, Minghao. Prediction of “Aggregation-Prone” Peptides with Hybrid Classification Approach. Mathematical Problems in Engineering. 2015. Vol. 2015, no. 2015, pp.1-9.
https://search.emarefa.net/detail/BIM-1074907
Data Type
Journal Articles
Language
English
Notes
Includes bibliographical references
Record ID
BIM-1074907