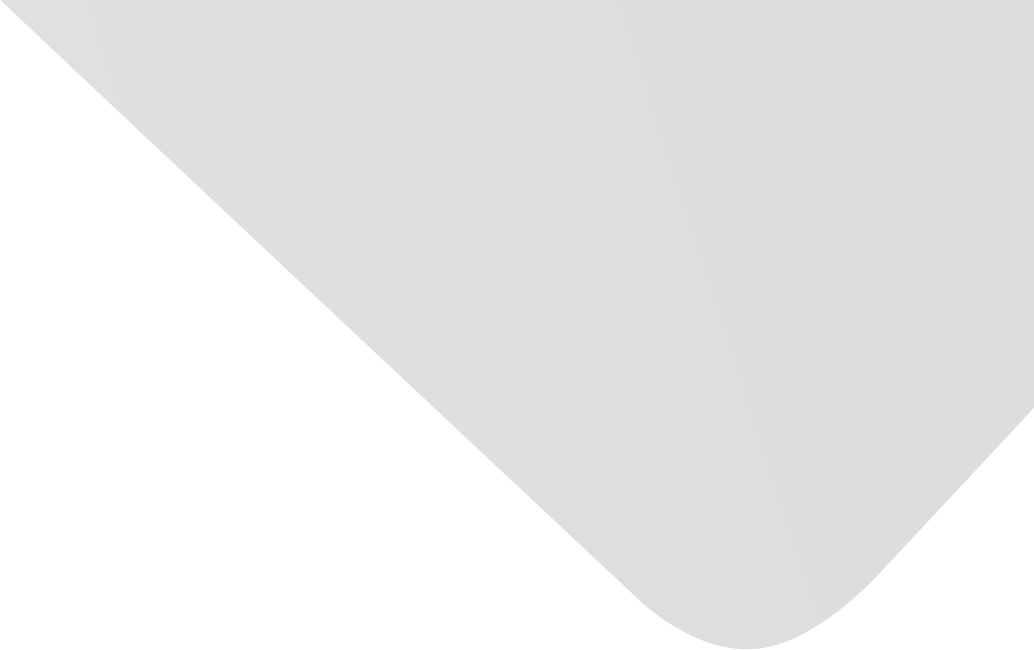
Forecasting CDS Term Structure Based on Nelson–Siegel Model and Machine Learning
Joint Authors
Kim, Won Joong
Jung, Gunho
Choi, Sun-Yong
Source
Issue
Vol. 2020, Issue 2020 (31 Dec. 2020), pp.1-23, 23 p.
Publisher
Hindawi Publishing Corporation
Publication Date
2020-07-14
Country of Publication
Egypt
No. of Pages
23
Main Subjects
Abstract EN
In this study, we analyze the term structure of credit default swaps (CDSs) and predict future term structures using the Nelson–Siegel model, recurrent neural network (RNN), support vector regression (SVR), long short-term memory (LSTM), and group method of data handling (GMDH) using CDS term structure data from 2008 to 2019.
Furthermore, we evaluate the change in the forecasting performance of the models through a subperiod analysis.
According to the empirical results, we confirm that the Nelson–Siegel model can be used to predict not only the interest rate term structure but also the CDS term structure.
Additionally, we demonstrate that machine-learning models, namely, SVR, RNN, LSTM, and GMDH, outperform the model-driven methods (in this case, the Nelson–Siegel model).
Among the machine learning approaches, GMDH demonstrates the best performance in forecasting the CDS term structure.
According to the subperiod analysis, the performance of all models was inconsistent with the data period.
All the models were less predictable in highly volatile data periods than in less volatile periods.
This study will enable traders and policymakers to invest efficiently and make policy decisions based on the current and future risk factors of a company or country.
American Psychological Association (APA)
Kim, Won Joong& Jung, Gunho& Choi, Sun-Yong. 2020. Forecasting CDS Term Structure Based on Nelson–Siegel Model and Machine Learning. Complexity،Vol. 2020, no. 2020, pp.1-23.
https://search.emarefa.net/detail/BIM-1141062
Modern Language Association (MLA)
Kim, Won Joong…[et al.]. Forecasting CDS Term Structure Based on Nelson–Siegel Model and Machine Learning. Complexity No. 2020 (2020), pp.1-23.
https://search.emarefa.net/detail/BIM-1141062
American Medical Association (AMA)
Kim, Won Joong& Jung, Gunho& Choi, Sun-Yong. Forecasting CDS Term Structure Based on Nelson–Siegel Model and Machine Learning. Complexity. 2020. Vol. 2020, no. 2020, pp.1-23.
https://search.emarefa.net/detail/BIM-1141062
Data Type
Journal Articles
Language
English
Notes
Includes bibliographical references
Record ID
BIM-1141062