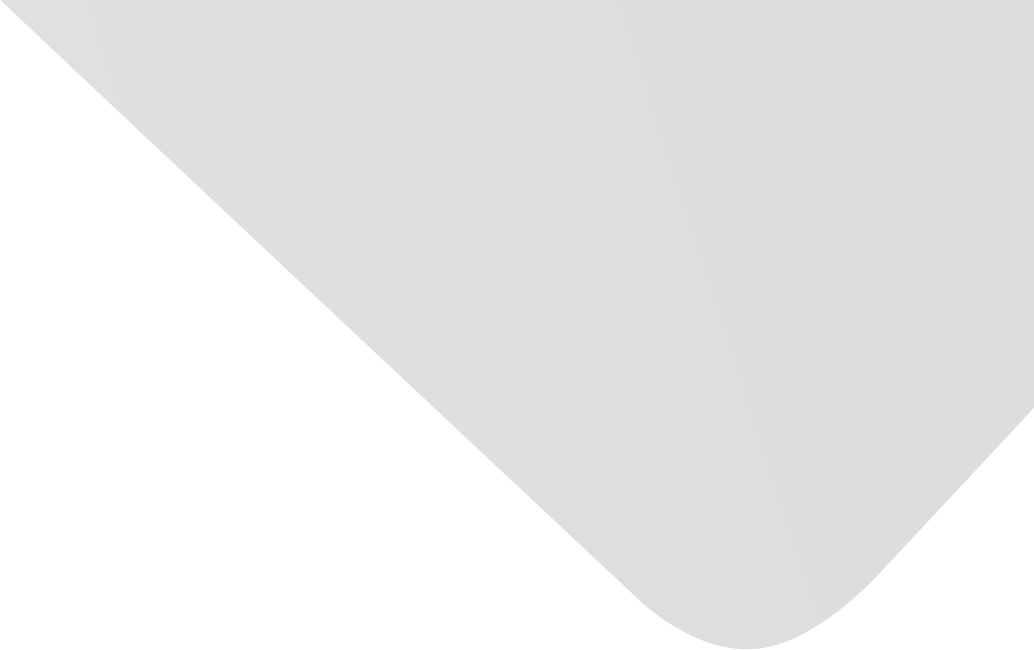
Deep Learning Approach for Building Detection Using LiDAR–Orthophoto Fusion
Joint Authors
Pradhan, Biswajeet
Mohd Shafri, Helmi Zulhaidi
Sameen, Maher Ibrahim
Nahhas, Faten Hamed
Mansor, Shattri
Source
Issue
Vol. 2018, Issue 2018 (31 Dec. 2018), pp.1-12, 12 p.
Publisher
Hindawi Publishing Corporation
Publication Date
2018-08-05
Country of Publication
Egypt
No. of Pages
12
Main Subjects
Abstract EN
This paper reports on a building detection approach based on deep learning (DL) using the fusion of Light Detection and Ranging (LiDAR) data and orthophotos.
The proposed method utilized object-based analysis to create objects, a feature-level fusion, an autoencoder-based dimensionality reduction to transform low-level features into compressed features, and a convolutional neural network (CNN) to transform compressed features into high-level features, which were used to classify objects into buildings and background.
The proposed architecture was optimized for the grid search method, and its sensitivity to hyperparameters was analyzed and discussed.
The proposed model was evaluated on two datasets selected from an urban area with different building types.
Results show that the dimensionality reduction by the autoencoder approach from 21 features to 10 features can improve detection accuracy from 86.06% to 86.19% in the working area and from 77.92% to 78.26% in the testing area.
The sensitivity analysis also shows that the selection of the hyperparameter values of the model significantly affects detection accuracy.
The best hyperparameters of the model are 128 filters in the CNN model, the Adamax optimizer, 10 units in the fully connected layer of the CNN model, a batch size of 8, and a dropout of 0.2.
These hyperparameters are critical to improving the generalization capacity of the model.
Furthermore, comparison experiments with the support vector machine (SVM) show that the proposed model with or without dimensionality reduction outperforms the SVM models in the working area.
However, the SVM model achieves better accuracy in the testing area than the proposed model without dimensionality reduction.
This study generally shows that the use of an autoencoder in DL models can improve the accuracy of building recognition in fused LiDAR–orthophoto data.
American Psychological Association (APA)
Nahhas, Faten Hamed& Mohd Shafri, Helmi Zulhaidi& Sameen, Maher Ibrahim& Pradhan, Biswajeet& Mansor, Shattri. 2018. Deep Learning Approach for Building Detection Using LiDAR–Orthophoto Fusion. Journal of Sensors،Vol. 2018, no. 2018, pp.1-12.
https://search.emarefa.net/detail/BIM-1201959
Modern Language Association (MLA)
Nahhas, Faten Hamed…[et al.]. Deep Learning Approach for Building Detection Using LiDAR–Orthophoto Fusion. Journal of Sensors No. 2018 (2018), pp.1-12.
https://search.emarefa.net/detail/BIM-1201959
American Medical Association (AMA)
Nahhas, Faten Hamed& Mohd Shafri, Helmi Zulhaidi& Sameen, Maher Ibrahim& Pradhan, Biswajeet& Mansor, Shattri. Deep Learning Approach for Building Detection Using LiDAR–Orthophoto Fusion. Journal of Sensors. 2018. Vol. 2018, no. 2018, pp.1-12.
https://search.emarefa.net/detail/BIM-1201959
Data Type
Journal Articles
Language
English
Notes
Includes bibliographical references
Record ID
BIM-1201959