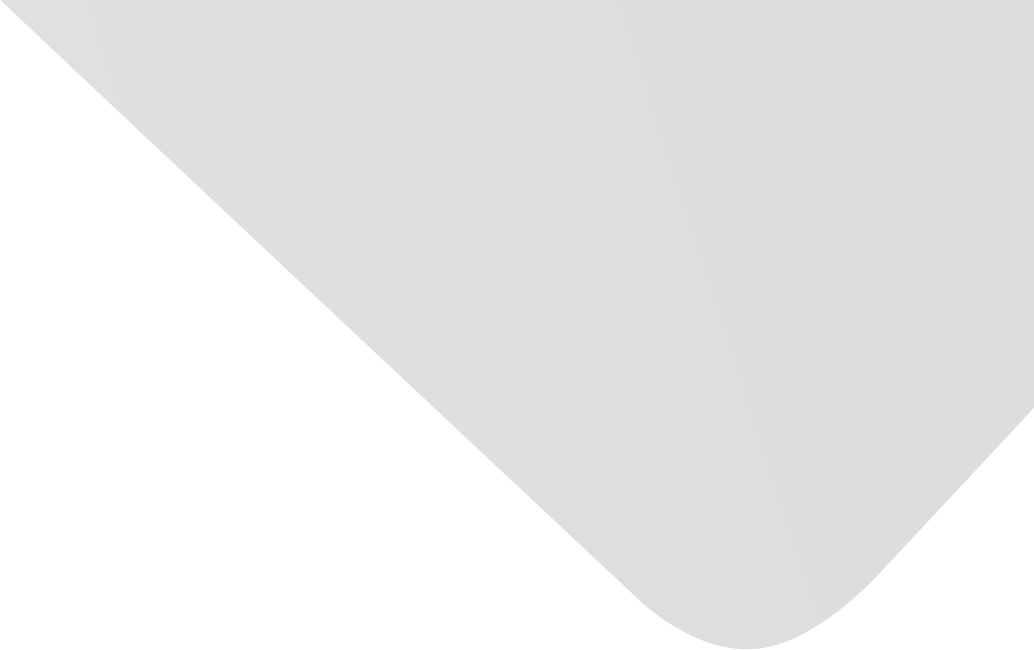
التنبؤ لبيانات تلوث الهواء باستخدام الطريقة الهجينة RNN-Wavelet بالاعتماد على نموذج MLR
Joint Authors
ختام وليد قادر
حنون، أسامة بشير شكر
Source
المجلة العراقية للعلوم الإحصائية
Issue
Vol. 18, Issue 34 (31 Dec. 2021), pp.40-55, 16 p.
Publisher
University of Mosul College of Computer Science and Mathematics
Publication Date
2021-12-31
Country of Publication
Iraq
No. of Pages
16
Main Subjects
Information Technology and Computer Science
Topics
Abstract AR
ان دراسة الجسيمات المعلقة ((PM10) Particular matter) و التكهن بها ضروري للتقليل و السيطرة على الأضرار البيئية و صحة الإنسان.
هنالك العديد من مصادر التلوث او ما يسمى بالملوثات والتي ربما تؤثر على متغير PM10.
كل هذه المتغيرات تصنف بياناتها كغير خطية.
أخذ بيانات الدراسة من محطة مناخية في ماليزيا.
و تم استخدام نماذج الانحدار الخطي المتعدد Multiple linear regression (MLR) كطريقة إحصائية خطية للتنبؤ بمتغير PM10 من خلال تأثره بمتغيرات الأرصاد الجوية المقابلة، لذلك فقد يعكس نتائج غير دقيقة عند استخدامه مع مجموعات البيانات غير الخطية.
و لتحسين نتائج التنبؤ تم استخدام الشبكات العصبية المعاودةRecurrent neural network (RNN) من خلال توفيقه مع نموذج MLR ضمن الطريقة الهجينة MLR-RNN.
و كذلك تم استخدام تحليل المويجات WaveIet في ترشيح النتائج المستحصلة من طريقة MLR-RNN للحصول على تحسين أكثر للنتائج التنبؤ من خلال ما يسمى الطريقة الهجينة RNN-Wavelet و اعتمادا على نموذج MLR.
ان أفضل نتائج التنبؤ بشكل عام كانت باستخدام الطريقة الهجينة RNN-Wavelet.
أن أفضل نتائج التنبؤ بشكل عام كانت باستخدام الطريقة المقترحة الهجينة RNN-Wavelet.
كما عكست النتائج افضلية تنبؤات الطرق الهجينة مقارنة مع الطريقة التقليدية المتمثلة بنموذج MLR.
و كاستنتاج في هذه الدراسة فمن الممكن استخدام التحليل المويجي بعد تهجينه مع الشبكات العصبية المعاودة اعتمادا على نموذج الانحدار الخطي المتعدد كأسلوب فعال للحصول على أفضل نتائج التنبؤ مع البيانات غير الخطية متعددة المتغيرات عندما يكون PM10 كمتغير معتمد.
Abstract EN
Particular matter (PM10) studying and forecasting is necessary to control and reduce the damage of environment and human health.
There are many pollutants as sources of air pollution may effect on PM10 variable.
This type of dataset can be classified as nonlinear.
Studied datasets have been taken from climate station in Malaysia.
Multiple linear regression (MLR) is used as linear statistical method for PM10 forecasting through its influencing by corresponding climate variables, therefore it may reflect inaccurate results when used with nonlinear datasets.
To improve the results of forecasting, recurrent neural network (RNN) has been suggested to be used after combining with MLR in hybrid in this study.
Wavelet analysis is proposed filtering the result of MLR-RNN method for more improving of forecasting results through RNN-Wavelet hybrid method based on MLR model.
In general, the best results of forecasting were for using RNN-Wavelet method.
.
In addition, the results of hybrid methods were outperformed comparing to MLR model as traditional method.
As conclusion in this study, Wavelet analysis can be used after hybridizing with RNN based on MLR as active approach to obtain better forecasting results with nonlinear datasets in which PM10 is to dependent variable.
American Psychological Association (APA)
ختام وليد قادر وحنون، أسامة بشير شكر. 2021. التنبؤ لبيانات تلوث الهواء باستخدام الطريقة الهجينة RNN-Wavelet بالاعتماد على نموذج MLR. المجلة العراقية للعلوم الإحصائية،مج. 18، ع. 34، ص ص. 40-55.
https://search.emarefa.net/detail/BIM-1339857
Modern Language Association (MLA)
ختام وليد قادر وحنون، أسامة بشير شكر. التنبؤ لبيانات تلوث الهواء باستخدام الطريقة الهجينة RNN-Wavelet بالاعتماد على نموذج MLR. المجلة العراقية للعلوم الإحصائية مج. 18، ع. 34 (2021)، ص ص. 40-55.
https://search.emarefa.net/detail/BIM-1339857
American Medical Association (AMA)
ختام وليد قادر وحنون، أسامة بشير شكر. التنبؤ لبيانات تلوث الهواء باستخدام الطريقة الهجينة RNN-Wavelet بالاعتماد على نموذج MLR. المجلة العراقية للعلوم الإحصائية. 2021. مج. 18، ع. 34، ص ص. 40-55.
https://search.emarefa.net/detail/BIM-1339857
Data Type
Journal Articles
Language
Arabic
Notes
يتضمن مراجع ببليوجرافية : ص. 54-55
Record ID
BIM-1339857