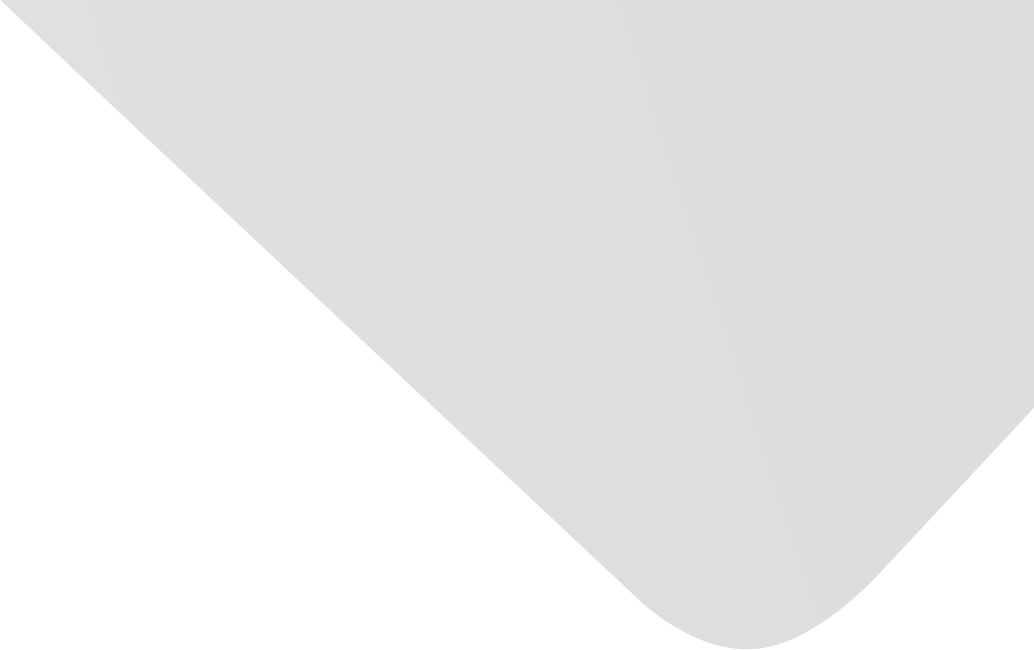
اكتشاف البيانات الشاذة في تدفقات انترنت الأشياء : دراسة استقصائية وتوجهات مستقبلية
Other Title(s)
Anomaly detection in internet of things streams survey and future trends
Joint Authors
الدوه جي، صلاح
الحمود، سهيل
أسامة إبراهيم
Source
مجلة جامعة دمشق للعلوم الهندسية : مجلة علمية محكمة دورية
Publisher
Publication Date
2022-12-31
Country of Publication
Syria
No. of Pages
12
Main Subjects
Arabic Abstract
إن النمو السريع لانتشار أجهزة إنترنت الأشياء أصبح يحدث فارقا كبيرا في حياتنا اليومية، و يساعدنا على اتخاذ قرارات حاسمة في كثير من قطاعات الحياة.
حقيقة إن طبيعة هذه البيئة تفرض اعتبارات معينة، فعلى عكس الشبكات في الماضي فقد كانت مكونة من عدد صغير من آلات الأغراض العامة، فإن شبكات إنترنت الأشياء تتكون بشكل متزايد من عدد كبير من الأجهزة المتخصصة المصممة للقيام بمهمة واحدة، و مما لا يمكن تجاهله أن المهمة المتخصصة والطبيعة المقيدة لهذه الأجهزة في كثير من الأحيان يجعل من الصعب تأمينها، و لكن من السهل تحليلها خارجيا، و هنا لا يمكننا إغفال مسألة الموارد الحسابية و التخزينية المحدودة في أنظمة إنترنت الأشياء، و التي تضع قيودا على تثبيت برامج الأمن التقليدية ؛ لذلك فإن الأنظمة التقليدية للكشف عن التسلل (IDS) أصبحت غير فعالة في هذه البيئة؛ بسبب طبيعتها غير المتجانسة، والازدياد التدريجي لنقاط الضعف؛ لذلك كان لابد من إعادة دراسة هذا المجال ضمن قيود هذه البيئة، كون موارد المعالجة المحدودة لا تسمح باستخدام آليات الأمان القياسية على العقد، و تقديم منهجيات تراعي هذه القيود وتحل هذه المسألة ضمن سياق البيانات الضخمة.
نقدم في هذه الدراسة الاستقصائية مراجعة حول مختلف التقنيات والمنهجيات المستخدمة في اكتشاف البيانات الشاذة في تدفقات البيانات الضخمة بشكل عام وتدفقات انترنت الأشياء بشكل خاص بالاعتماد على طرائق التعلم الآلي مع دراسة مقارنة لتحديد الخوارزمية الأفضل للعمل على تطويرها لاحقا لتكون مناسبة للعمل في بيئات الشبكات محدودة الموارد، و بناء نموذج مفاهيمي لاكتشاف الشذوذ في بيئة انترنت الأشياء معتمد على الخوارزمية الأفضل ضمن سياق هذه البيئة ومفهوم البيانات الوصفية، و الخوارزميات وبنى المعطيات الاحتمالية.
بنتيجة الدراسة المقارنة وجدنا أن خوارزمية الغابة العشوائية Random Forest هي الخوارزمية الأفضل في بيئات البيانات الضخمة بشكل عام، وبيئة انترنت الأشياء بشكل خاص، و قدمنا مقاربة اكتشاف هجينة متطورة ذاتيا وديناميكية من حيث دقة الكشف.
English Abstract
The rapid growth of Internet of Things (IoT) devices is making a huge difference in our daily lives and helps us make critical decisions in many sectors of life.
Indeed, the nature of this environment dictates certain considerations.
Unlike networks in the past that were made up of a small number of general-purpose machines, IoT networks are increasingly made up of a large number of specialized devices designed to do a single task.
The Specialized task and Constrained nature of these devices often makes them difficult to secure, but it is easy to analyze them externally, and here we cannot overlook the issue of limited computational and storage resources in IoT systems, which place restrictions on the installation of traditional security software; Therefore, traditional intrusion detection systems (IDS) have become ineffective in this environment; Because of its heterogeneous nature and the gradual increase in vulnerabilities, so it was necessary to re-study this field within the constraints of this environment, Because of limited processing resources which do not allow the use of standard security mechanisms on nodes, and provide methodologies that take into account these limitations and solve this issue within the context of big data.
In this survey, we present a review of the various techniques and methodologies used in Detecting anomalies in Big Data streams in general and Internet of Things streams in particular, based on machine learning methods with a comparative study to determine the best algorithm to work on to develop it later to be suitable for working in Constrained networks environments Resources, and building a conceptual model for anomaly detection in the Internet of Things environment based on the best algorithm within the context of this environment and the concept of Metadata, probabilistic data structures and algorithms.
As a result of the comparative study, we found that the Random Forest algorithm is the best algorithm in the big data environments in general, and the Internet of Things environment in particular, and we presented a self- evolving and dynamic hybrid detection approach in terms of detection accuracy.
Data Type
Conference Papers
Record ID
BIM-1529930
American Psychological Association (APA)
أسامة إبراهيم والدوه جي، صلاح والحمود، سهيل. 2022-12-31. اكتشاف البيانات الشاذة في تدفقات انترنت الأشياء : دراسة استقصائية وتوجهات مستقبلية. مؤتمر الهندسة المعلوماتية (1 : 2022 : دمشق، سوريا) :1) 2022 : دمشق، سوريا). . مج. 38، ع. 4 (s+conf) (2022)، ص ص. 125-136.دمشق، سوريا : جامعة دمشق،.
https://search.emarefa.net/detail/BIM-1529930
Modern Language Association (MLA)
أسامة إبراهيم....[و آخرون]. اكتشاف البيانات الشاذة في تدفقات انترنت الأشياء : دراسة استقصائية وتوجهات مستقبلية. . دمشق، سوريا : جامعة دمشق،. 2022-12-31.
https://search.emarefa.net/detail/BIM-1529930
American Medical Association (AMA)
أسامة إبراهيم والدوه جي، صلاح والحمود، سهيل. اكتشاف البيانات الشاذة في تدفقات انترنت الأشياء : دراسة استقصائية وتوجهات مستقبلية. . مؤتمر الهندسة المعلوماتية (1 : 2022 : دمشق، سوريا) :1) 2022 : دمشق، سوريا).
https://search.emarefa.net/detail/BIM-1529930