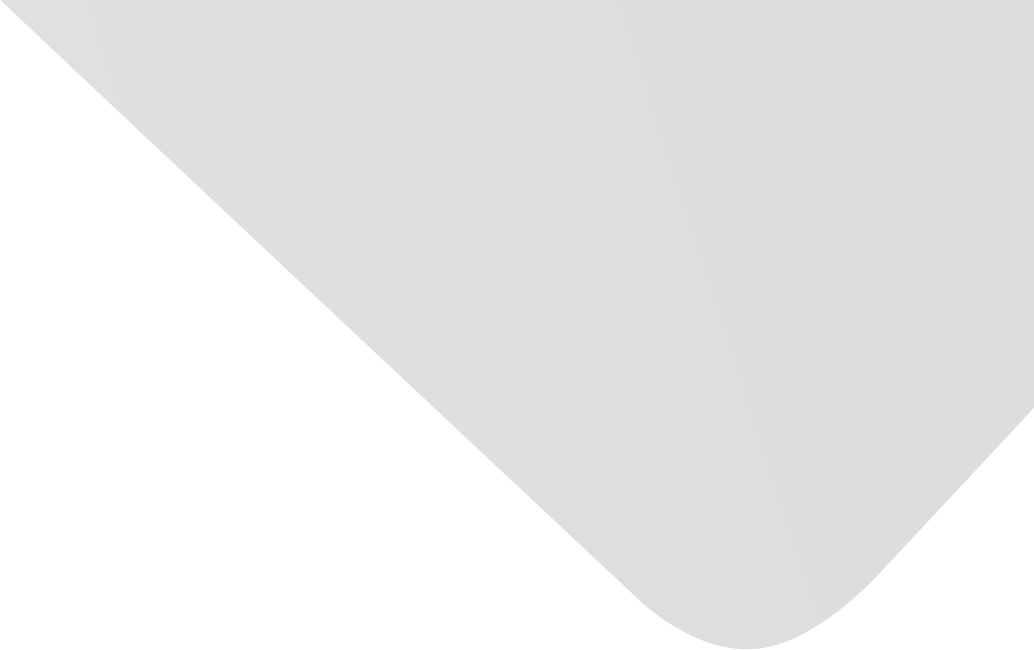
ℓp-Norm Multikernel Learning Approach for Stock Market Price Forecasting
Joint Authors
Wu, Kun
Liao, Bifeng
Shao, Xigao
Source
Computational Intelligence and Neuroscience
Issue
Vol. 2012, Issue 2012 (31 Dec. 2012), pp.1-10, 10 p.
Publisher
Hindawi Publishing Corporation
Publication Date
2012-12-29
Country of Publication
Egypt
No. of Pages
10
Main Subjects
Abstract EN
Linear multiple kernel learning model has been used for predicting financial time series.
However, ℓ1-norm multiple support vector regression is rarely observed to outperform trivial baselines in practical applications.
To allow for robust kernel mixtures that generalize well, we adopt ℓp-norm multiple kernel support vector regression (1≤p<∞) as a stock price prediction model.
The optimization problem is decomposed into smaller subproblems, and the interleaved optimization strategy is employed to solve the regression model.
The model is evaluated on forecasting the daily stock closing prices of Shanghai Stock Index in China.
Experimental results show that our proposed model performs better than ℓ1-norm multiple support vector regression model.
American Psychological Association (APA)
Shao, Xigao& Wu, Kun& Liao, Bifeng. 2012. ℓp-Norm Multikernel Learning Approach for Stock Market Price Forecasting. Computational Intelligence and Neuroscience،Vol. 2012, no. 2012, pp.1-10.
https://search.emarefa.net/detail/BIM-484081
Modern Language Association (MLA)
Shao, Xigao…[et al.]. ℓp-Norm Multikernel Learning Approach for Stock Market Price Forecasting. Computational Intelligence and Neuroscience No. 2012 (2012), pp.1-10.
https://search.emarefa.net/detail/BIM-484081
American Medical Association (AMA)
Shao, Xigao& Wu, Kun& Liao, Bifeng. ℓp-Norm Multikernel Learning Approach for Stock Market Price Forecasting. Computational Intelligence and Neuroscience. 2012. Vol. 2012, no. 2012, pp.1-10.
https://search.emarefa.net/detail/BIM-484081
Data Type
Journal Articles
Language
English
Notes
Includes bibliographical references
Record ID
BIM-484081