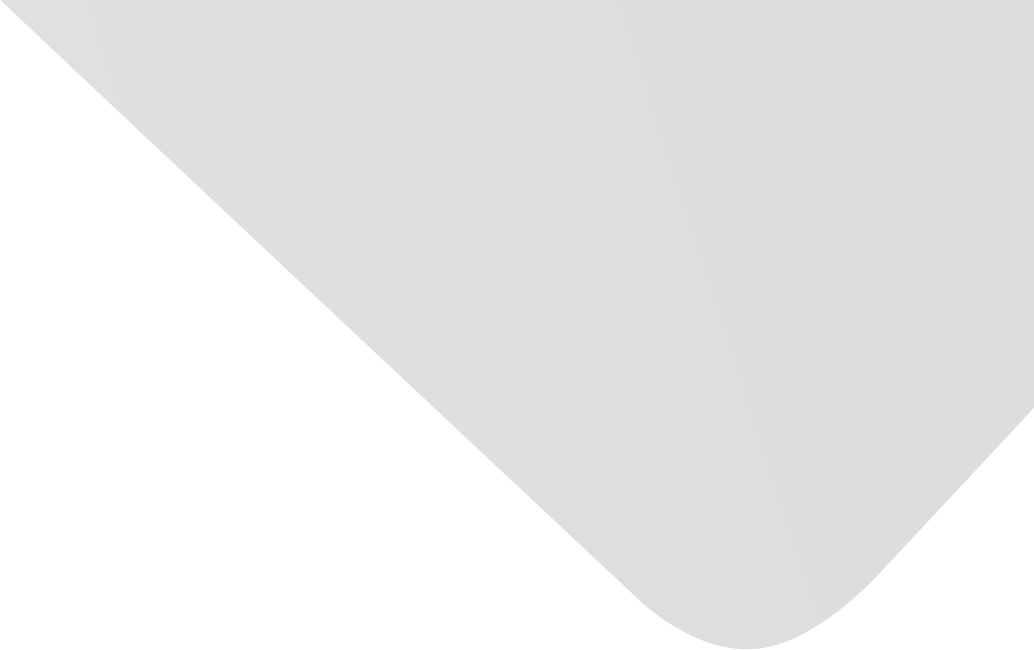
The Hybrid KICA-GDA-LSSVM Method Research on Rolling Bearing Fault Feature Extraction and Classification
المؤلفون المشاركون
Li, Jiyong
Li, Shunming
Chen, Xiaohong
Wang, Lili
المصدر
العدد
المجلد 2015، العدد 2015 (31 ديسمبر/كانون الأول 2015)، ص ص. 1-9، 9ص.
الناشر
Hindawi Publishing Corporation
تاريخ النشر
2015-02-02
دولة النشر
مصر
عدد الصفحات
9
التخصصات الرئيسية
الملخص EN
Rolling element bearings are widely used in high-speed rotating machinery; thus proper monitoring and fault diagnosis procedure to avoid major machine failures is necessary.
As feature extraction and classification based on vibration signals are important in condition monitoring technique, and superfluous features may degrade the classification performance, it is needed to extract independent features, so LSSVM (least square support vector machine) based on hybrid KICA-GDA (kernel independent component analysis-generalized discriminate analysis) is presented in this study.
A new method named sensitive subband feature set design (SSFD) based on wavelet packet is also presented; using proposed variance differential spectrum method, the sensitive subbands are selected.
Firstly, independent features are obtained by KICA; the feature redundancy is reduced.
Secondly, feature dimension is reduced by GDA.
Finally, the projected feature is classified by LSSVM.
The whole paper aims to classify the feature vectors extracted from the time series and magnitude of spectral analysis and to discriminate the state of the rolling element bearings by virtue of multiclass LSSVM.
Experimental results from two different fault-seeded bearing tests show good performance of the proposed method.
نمط استشهاد جمعية علماء النفس الأمريكية (APA)
Li, Jiyong& Li, Shunming& Chen, Xiaohong& Wang, Lili. 2015. The Hybrid KICA-GDA-LSSVM Method Research on Rolling Bearing Fault Feature Extraction and Classification. Shock and Vibration،Vol. 2015, no. 2015, pp.1-9.
https://search.emarefa.net/detail/BIM-1078186
نمط استشهاد الجمعية الأمريكية للغات الحديثة (MLA)
Li, Jiyong…[et al.]. The Hybrid KICA-GDA-LSSVM Method Research on Rolling Bearing Fault Feature Extraction and Classification. Shock and Vibration No. 2015 (2015), pp.1-9.
https://search.emarefa.net/detail/BIM-1078186
نمط استشهاد الجمعية الطبية الأمريكية (AMA)
Li, Jiyong& Li, Shunming& Chen, Xiaohong& Wang, Lili. The Hybrid KICA-GDA-LSSVM Method Research on Rolling Bearing Fault Feature Extraction and Classification. Shock and Vibration. 2015. Vol. 2015, no. 2015, pp.1-9.
https://search.emarefa.net/detail/BIM-1078186
نوع البيانات
مقالات
لغة النص
الإنجليزية
الملاحظات
Includes bibliographical references
رقم السجل
BIM-1078186
قاعدة معامل التأثير والاستشهادات المرجعية العربي "ارسيف Arcif"
أضخم قاعدة بيانات عربية للاستشهادات المرجعية للمجلات العلمية المحكمة الصادرة في العالم العربي
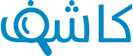
تقوم هذه الخدمة بالتحقق من التشابه أو الانتحال في الأبحاث والمقالات العلمية والأطروحات الجامعية والكتب والأبحاث باللغة العربية، وتحديد درجة التشابه أو أصالة الأعمال البحثية وحماية ملكيتها الفكرية. تعرف اكثر
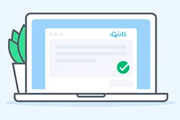