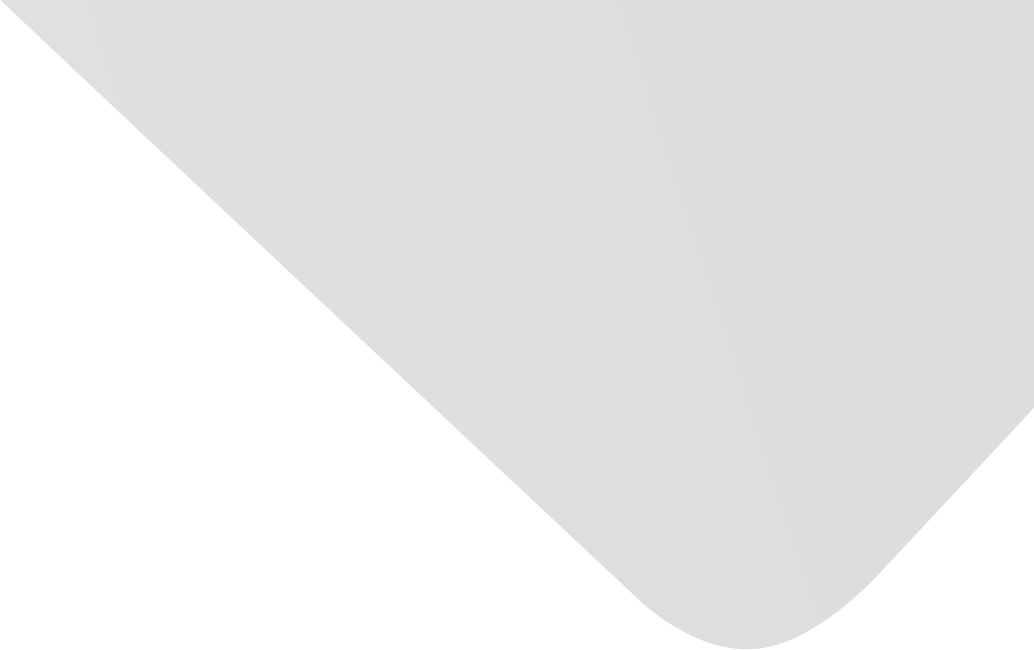
Online Knowledge-Based Model for Big Data Topic Extraction
المؤلفون المشاركون
Khan, Muhammad Taimoor
Durrani, Mehr
Aziz, Furqan
Khalid, Shehzad
المصدر
Computational Intelligence and Neuroscience
العدد
المجلد 2016، العدد 2016 (31 ديسمبر/كانون الأول 2015)، ص ص. 1-10، 10ص.
الناشر
Hindawi Publishing Corporation
تاريخ النشر
2016-04-19
دولة النشر
مصر
عدد الصفحات
10
التخصصات الرئيسية
الملخص EN
Lifelong machine learning (LML) models learn with experience maintaining a knowledge-base, without user intervention.
Unlike traditional single-domain models they can easily scale up to explore big data.
The existing LML models have high data dependency, consume more resources, and do not support streaming data.
This paper proposes online LML model (OAMC) to support streaming data with reduced data dependency.
With engineering the knowledge-base and introducing new knowledge features the learning pattern of the model is improved for data arriving in pieces.
OAMC improves accuracy as topic coherence by 7% for streaming data while reducing the processing cost to half.
نمط استشهاد جمعية علماء النفس الأمريكية (APA)
Khan, Muhammad Taimoor& Durrani, Mehr& Khalid, Shehzad& Aziz, Furqan. 2016. Online Knowledge-Based Model for Big Data Topic Extraction. Computational Intelligence and Neuroscience،Vol. 2016, no. 2016, pp.1-10.
https://search.emarefa.net/detail/BIM-1099719
نمط استشهاد الجمعية الأمريكية للغات الحديثة (MLA)
Khan, Muhammad Taimoor…[et al.]. Online Knowledge-Based Model for Big Data Topic Extraction. Computational Intelligence and Neuroscience Vol. 2016, no. 2016 (2015), pp.1-10.
https://search.emarefa.net/detail/BIM-1099719
نمط استشهاد الجمعية الطبية الأمريكية (AMA)
Khan, Muhammad Taimoor& Durrani, Mehr& Khalid, Shehzad& Aziz, Furqan. Online Knowledge-Based Model for Big Data Topic Extraction. Computational Intelligence and Neuroscience. 2016. Vol. 2016, no. 2016, pp.1-10.
https://search.emarefa.net/detail/BIM-1099719
نوع البيانات
مقالات
لغة النص
الإنجليزية
الملاحظات
Includes bibliographical references
رقم السجل
BIM-1099719
قاعدة معامل التأثير والاستشهادات المرجعية العربي "ارسيف Arcif"
أضخم قاعدة بيانات عربية للاستشهادات المرجعية للمجلات العلمية المحكمة الصادرة في العالم العربي
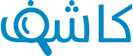
تقوم هذه الخدمة بالتحقق من التشابه أو الانتحال في الأبحاث والمقالات العلمية والأطروحات الجامعية والكتب والأبحاث باللغة العربية، وتحديد درجة التشابه أو أصالة الأعمال البحثية وحماية ملكيتها الفكرية. تعرف اكثر
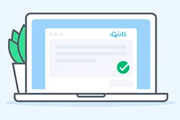