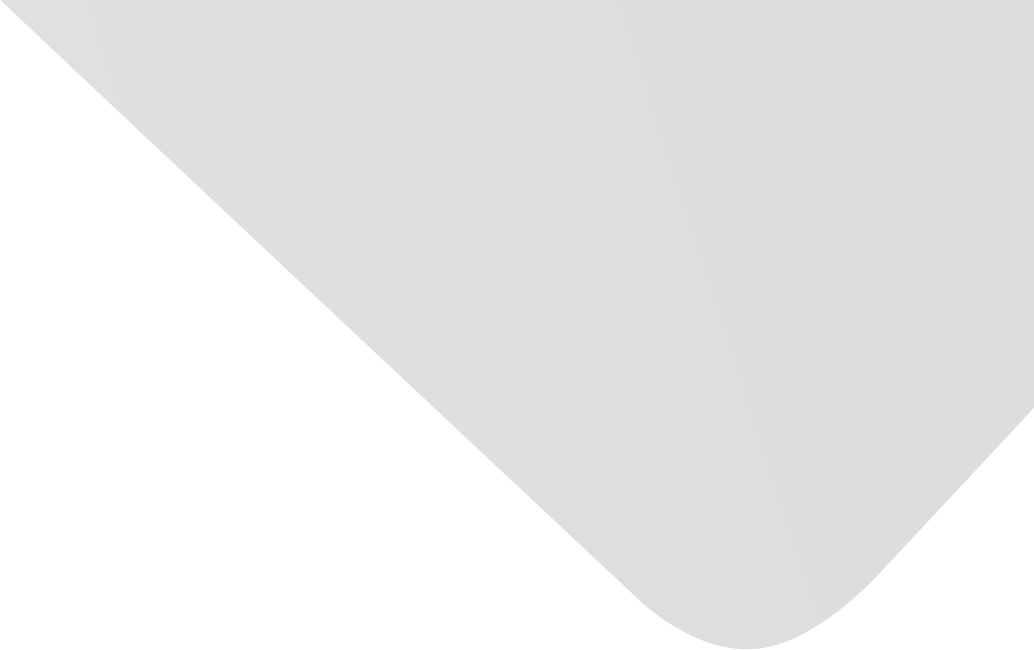
Instance-Wise Denoising Autoencoder for High Dimensional Data
المؤلفون المشاركون
المصدر
Mathematical Problems in Engineering
العدد
المجلد 2016، العدد 2016 (31 ديسمبر/كانون الأول 2016)، ص ص. 1-13، 13ص.
الناشر
Hindawi Publishing Corporation
تاريخ النشر
2016-10-31
دولة النشر
مصر
عدد الصفحات
13
التخصصات الرئيسية
الملخص EN
Denoising Autoencoder (DAE) is one of the most popular fashions that has reported significant success in recent neural network research.
To be specific, DAE randomly corrupts some features of the data to zero as to utilize the cooccurrence information while avoiding overfitting.
However, existing DAE approaches do not fare well on sparse and high dimensional data.
In this paper, we present a Denoising Autoencoder labeled here as Instance-Wise Denoising Autoencoder (IDA), which is designed to work with high dimensional and sparse data by utilizing the instance-wise cooccurrence relation instead of the feature-wise one.
IDA works ahead based on the following corruption rule: if an instance vector of nonzero feature is selected, it is forced to become a zero vector.
To avoid serious information loss in the event that too many instances are discarded, an ensemble of multiple independent autoencoders built on different corrupted versions of the data is considered.
Extensive experimental results on high dimensional and sparse text data show the superiority of IDA in efficiency and effectiveness.
IDA is also experimented on the heterogenous transfer learning setting and cross-modal retrieval to study its generality on heterogeneous feature representation.
نمط استشهاد جمعية علماء النفس الأمريكية (APA)
Chen, Lin& Deng, Wan-Yu. 2016. Instance-Wise Denoising Autoencoder for High Dimensional Data. Mathematical Problems in Engineering،Vol. 2016, no. 2016, pp.1-13.
https://search.emarefa.net/detail/BIM-1112188
نمط استشهاد الجمعية الأمريكية للغات الحديثة (MLA)
Chen, Lin& Deng, Wan-Yu. Instance-Wise Denoising Autoencoder for High Dimensional Data. Mathematical Problems in Engineering No. 2016 (2016), pp.1-13.
https://search.emarefa.net/detail/BIM-1112188
نمط استشهاد الجمعية الطبية الأمريكية (AMA)
Chen, Lin& Deng, Wan-Yu. Instance-Wise Denoising Autoencoder for High Dimensional Data. Mathematical Problems in Engineering. 2016. Vol. 2016, no. 2016, pp.1-13.
https://search.emarefa.net/detail/BIM-1112188
نوع البيانات
مقالات
لغة النص
الإنجليزية
الملاحظات
Includes bibliographical references
رقم السجل
BIM-1112188
قاعدة معامل التأثير والاستشهادات المرجعية العربي "ارسيف Arcif"
أضخم قاعدة بيانات عربية للاستشهادات المرجعية للمجلات العلمية المحكمة الصادرة في العالم العربي
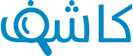
تقوم هذه الخدمة بالتحقق من التشابه أو الانتحال في الأبحاث والمقالات العلمية والأطروحات الجامعية والكتب والأبحاث باللغة العربية، وتحديد درجة التشابه أو أصالة الأعمال البحثية وحماية ملكيتها الفكرية. تعرف اكثر
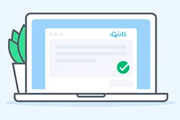