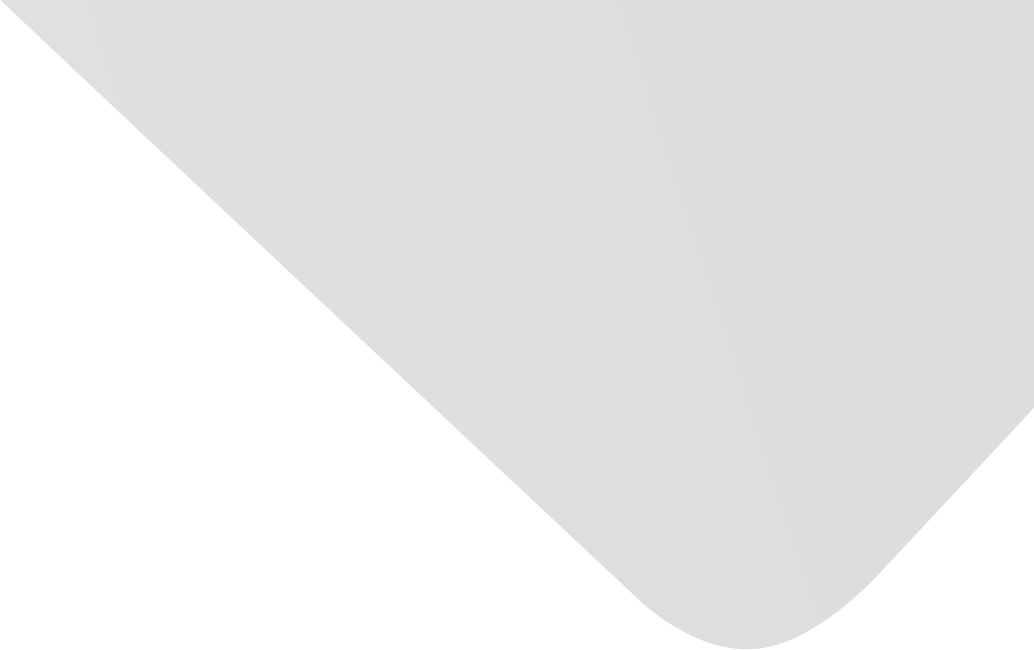
Boosting Accuracy of Classical Machine Learning Antispam Classifiers in Real Scenarios by Applying Rough Set Theory
المؤلفون المشاركون
Fdez-Riverola, Florentino
Pérez-Díaz, N.
Ruano-Ordás, D.
Méndez, J. R.
المصدر
العدد
المجلد 2016، العدد 2016 (31 ديسمبر/كانون الأول 2016)، ص ص. 1-10، 10ص.
الناشر
Hindawi Publishing Corporation
تاريخ النشر
2016-06-29
دولة النشر
مصر
عدد الصفحات
10
التخصصات الرئيسية
الملخص EN
Nowadays, spam deliveries represent a major problem to benefit from the wide range of Internet-based communication forms.
Despite the existence of different well-known intelligent techniques for fighting spam, only some specific implementations of Naïve Bayes algorithm are finally used in real environments for performance reasons.
As long as some of these algorithms suffer from a large number of false positive errors, in this work we propose a rough set postprocessing approach able to significantly improve their accuracy.
In order to demonstrate the advantages of the proposed method, we carried out a straightforward study based on a publicly available standard corpus (SpamAssassin), which compares the performance of previously successful well-known antispam classifiers (i.e., Support Vector Machines, AdaBoost, Flexible Bayes, and Naïve Bayes) with and without the application of our developed technique.
Results clearly evidence the suitability of our rough set postprocessing approach for increasing the accuracy of previous successful antispam classifiers when working in real scenarios.
نمط استشهاد جمعية علماء النفس الأمريكية (APA)
Pérez-Díaz, N.& Ruano-Ordás, D.& Fdez-Riverola, Florentino& Méndez, J. R.. 2016. Boosting Accuracy of Classical Machine Learning Antispam Classifiers in Real Scenarios by Applying Rough Set Theory. Scientific Programming،Vol. 2016, no. 2016, pp.1-10.
https://search.emarefa.net/detail/BIM-1118304
نمط استشهاد الجمعية الأمريكية للغات الحديثة (MLA)
Pérez-Díaz, N.…[et al.]. Boosting Accuracy of Classical Machine Learning Antispam Classifiers in Real Scenarios by Applying Rough Set Theory. Scientific Programming No. 2016 (2016), pp.1-10.
https://search.emarefa.net/detail/BIM-1118304
نمط استشهاد الجمعية الطبية الأمريكية (AMA)
Pérez-Díaz, N.& Ruano-Ordás, D.& Fdez-Riverola, Florentino& Méndez, J. R.. Boosting Accuracy of Classical Machine Learning Antispam Classifiers in Real Scenarios by Applying Rough Set Theory. Scientific Programming. 2016. Vol. 2016, no. 2016, pp.1-10.
https://search.emarefa.net/detail/BIM-1118304
نوع البيانات
مقالات
لغة النص
الإنجليزية
الملاحظات
Includes bibliographical references
رقم السجل
BIM-1118304
قاعدة معامل التأثير والاستشهادات المرجعية العربي "ارسيف Arcif"
أضخم قاعدة بيانات عربية للاستشهادات المرجعية للمجلات العلمية المحكمة الصادرة في العالم العربي
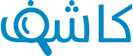
تقوم هذه الخدمة بالتحقق من التشابه أو الانتحال في الأبحاث والمقالات العلمية والأطروحات الجامعية والكتب والأبحاث باللغة العربية، وتحديد درجة التشابه أو أصالة الأعمال البحثية وحماية ملكيتها الفكرية. تعرف اكثر
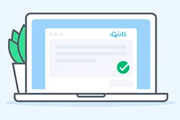