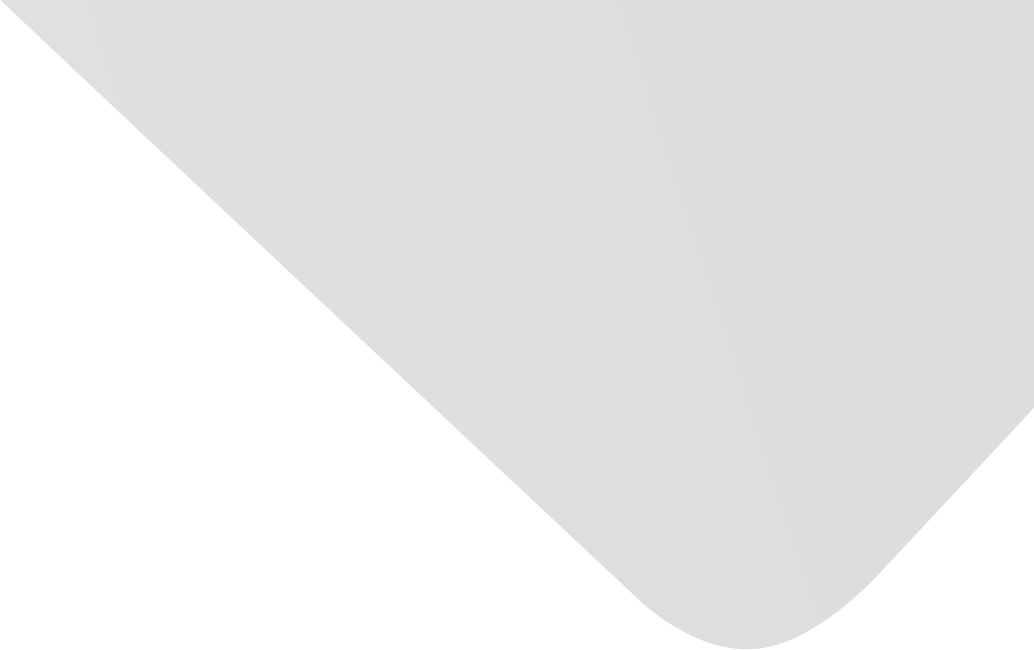
Assessing the Applicability of Random Forest, Stochastic Gradient Boosted Model, and Extreme Learning Machine Methods to the Quantitative Precipitation Estimation of the Radar Data: A Case Study to Gwangdeoksan Radar, South Korea, in 2018
المؤلفون المشاركون
Ro, Yonghun
Shin, Ju-Young
Cha, Joo-Wan
Kim, Kyu-Rang
Ha, Jong-Chul
المصدر
العدد
المجلد 2019، العدد 2019 (31 ديسمبر/كانون الأول 2019)، ص ص. 1-17، 17ص.
الناشر
Hindawi Publishing Corporation
تاريخ النشر
2019-10-07
دولة النشر
مصر
عدد الصفحات
17
التخصصات الرئيسية
الملخص EN
Machine learning algorithms should be tested for use in quantitative precipitation estimation models of rain radar data in South Korea because such an application can provide a more accurate estimate of rainfall than the conventional ZR relationship-based model.
The applicability of random forest, stochastic gradient boosted model, and extreme learning machine methods to quantitative precipitation estimation models was investigated using case studies with polarization radar data from Gwangdeoksan radar station.
Various combinations of input variable sets were tested, and results showed that machine learning algorithms can be applied to build the quantitative precipitation estimation model of the polarization radar data in South Korea.
The machine learning-based quantitative precipitation estimation models led to better performances than ZR relationship-based models, particularly for heavy rainfall events.
The extreme learning machine is considered the best of the algorithms used based on evaluation criteria.
نمط استشهاد جمعية علماء النفس الأمريكية (APA)
Shin, Ju-Young& Ro, Yonghun& Cha, Joo-Wan& Kim, Kyu-Rang& Ha, Jong-Chul. 2019. Assessing the Applicability of Random Forest, Stochastic Gradient Boosted Model, and Extreme Learning Machine Methods to the Quantitative Precipitation Estimation of the Radar Data: A Case Study to Gwangdeoksan Radar, South Korea, in 2018. Advances in Meteorology،Vol. 2019, no. 2019, pp.1-17.
https://search.emarefa.net/detail/BIM-1118729
نمط استشهاد الجمعية الأمريكية للغات الحديثة (MLA)
Shin, Ju-Young…[et al.]. Assessing the Applicability of Random Forest, Stochastic Gradient Boosted Model, and Extreme Learning Machine Methods to the Quantitative Precipitation Estimation of the Radar Data: A Case Study to Gwangdeoksan Radar, South Korea, in 2018. Advances in Meteorology No. 2019 (2019), pp.1-17.
https://search.emarefa.net/detail/BIM-1118729
نمط استشهاد الجمعية الطبية الأمريكية (AMA)
Shin, Ju-Young& Ro, Yonghun& Cha, Joo-Wan& Kim, Kyu-Rang& Ha, Jong-Chul. Assessing the Applicability of Random Forest, Stochastic Gradient Boosted Model, and Extreme Learning Machine Methods to the Quantitative Precipitation Estimation of the Radar Data: A Case Study to Gwangdeoksan Radar, South Korea, in 2018. Advances in Meteorology. 2019. Vol. 2019, no. 2019, pp.1-17.
https://search.emarefa.net/detail/BIM-1118729
نوع البيانات
مقالات
لغة النص
الإنجليزية
الملاحظات
Includes bibliographical references
رقم السجل
BIM-1118729
قاعدة معامل التأثير والاستشهادات المرجعية العربي "ارسيف Arcif"
أضخم قاعدة بيانات عربية للاستشهادات المرجعية للمجلات العلمية المحكمة الصادرة في العالم العربي
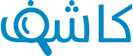
تقوم هذه الخدمة بالتحقق من التشابه أو الانتحال في الأبحاث والمقالات العلمية والأطروحات الجامعية والكتب والأبحاث باللغة العربية، وتحديد درجة التشابه أو أصالة الأعمال البحثية وحماية ملكيتها الفكرية. تعرف اكثر
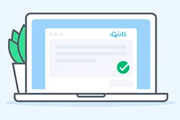