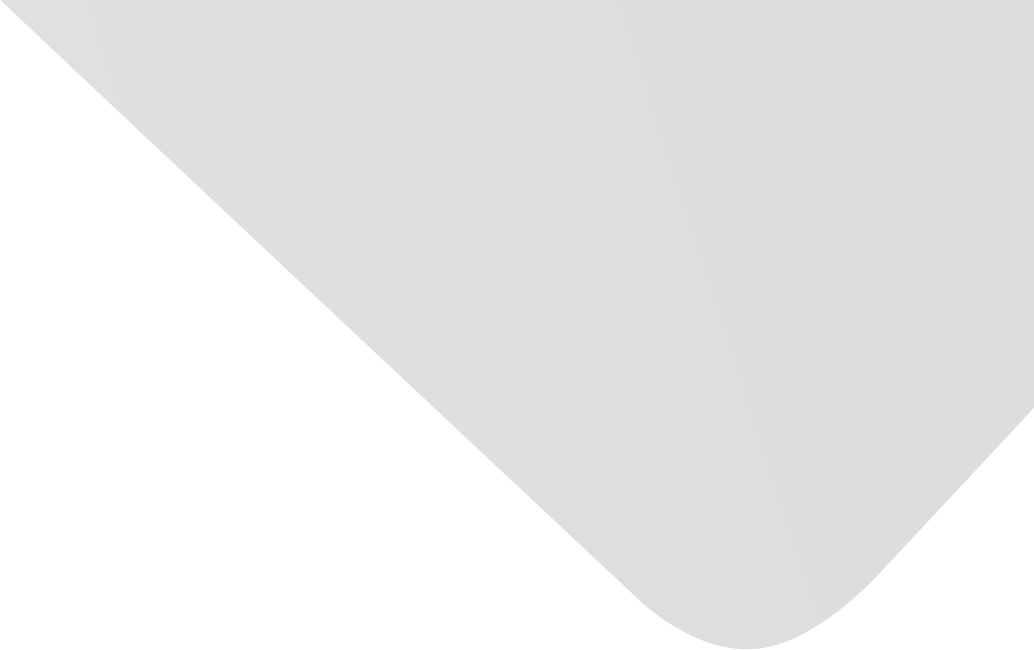
Adaptive Anomaly Detection Framework Model Objects in Cyberspace
المؤلفون المشاركون
Aldhyani, Theyazn H. H
Al-Yaari, Mohammed
Alkahtani, Hasan
المصدر
Applied Bionics and Biomechanics
العدد
المجلد 2020، العدد 2020 (31 ديسمبر/كانون الأول 2020)، ص ص. 1-14، 14ص.
الناشر
Hindawi Publishing Corporation
تاريخ النشر
2020-12-10
دولة النشر
مصر
عدد الصفحات
14
التخصصات الرئيسية
الملخص EN
Telecommunication has registered strong and rapid growth in the past decade.
Accordingly, the monitoring of computers and networks is too complicated for network administrators.
Hence, network security represents one of the biggest serious challenges that can be faced by network security communities.
Taking into consideration the fact that e-banking, e-commerce, and business data will be shared on the computer network, these data may face a threat from intrusion.
The purpose of this research is to propose a methodology that will lead to a high level and sustainable protection against cyberattacks.
In particular, an adaptive anomaly detection framework model was developed using deep and machine learning algorithms to manage automatically-configured application-level firewalls.
The standard network datasets were used to evaluate the proposed model which is designed for improving the cybersecurity system.
The deep learning based on Long-Short Term Memory Recurrent Neural Network (LSTM-RNN) and machine learning algorithms namely Support Vector Machine (SVM), K-Nearest Neighbor (K-NN) algorithms were implemented to classify the Denial-of-Service attack (DoS) and Distributed Denial-of-Service (DDoS) attacks.
The information gain method was applied to select the relevant features from the network dataset.
These network features were significant to improve the classification algorithm.
The system was used to classify DoS and DDoS attacks in four stand datasets namely KDD cup 199, NSL-KDD, ISCX, and ICI-ID2017.
The empirical results indicate that the deep learning based on the LSTM-RNN algorithm has obtained the highest accuracy.
The proposed system based on the LSTM-RNN algorithm produced the highest testing accuracy rate of 99.51% and 99.91% with respect to KDD Cup’99, NSL-KDD, ISCX, and ICI-Id2017 datasets, respectively.
A comparative result analysis between the machine learning algorithms, namely SVM and KNN, and the deep learning algorithms based on the LSTM-RNN model is presented.
Finally, it is concluded that the LSTM-RNN model is efficient and effective to improve the cybersecurity system for detecting anomaly-based cybersecurity.
نمط استشهاد جمعية علماء النفس الأمريكية (APA)
Alkahtani, Hasan& Aldhyani, Theyazn H. H& Al-Yaari, Mohammed. 2020. Adaptive Anomaly Detection Framework Model Objects in Cyberspace. Applied Bionics and Biomechanics،Vol. 2020, no. 2020, pp.1-14.
https://search.emarefa.net/detail/BIM-1120137
نمط استشهاد الجمعية الأمريكية للغات الحديثة (MLA)
Alkahtani, Hasan…[et al.]. Adaptive Anomaly Detection Framework Model Objects in Cyberspace. Applied Bionics and Biomechanics No. 2020 (2020), pp.1-14.
https://search.emarefa.net/detail/BIM-1120137
نمط استشهاد الجمعية الطبية الأمريكية (AMA)
Alkahtani, Hasan& Aldhyani, Theyazn H. H& Al-Yaari, Mohammed. Adaptive Anomaly Detection Framework Model Objects in Cyberspace. Applied Bionics and Biomechanics. 2020. Vol. 2020, no. 2020, pp.1-14.
https://search.emarefa.net/detail/BIM-1120137
نوع البيانات
مقالات
لغة النص
الإنجليزية
الملاحظات
Includes bibliographical references
رقم السجل
BIM-1120137
قاعدة معامل التأثير والاستشهادات المرجعية العربي "ارسيف Arcif"
أضخم قاعدة بيانات عربية للاستشهادات المرجعية للمجلات العلمية المحكمة الصادرة في العالم العربي
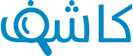
تقوم هذه الخدمة بالتحقق من التشابه أو الانتحال في الأبحاث والمقالات العلمية والأطروحات الجامعية والكتب والأبحاث باللغة العربية، وتحديد درجة التشابه أو أصالة الأعمال البحثية وحماية ملكيتها الفكرية. تعرف اكثر
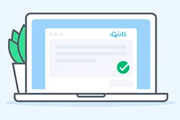