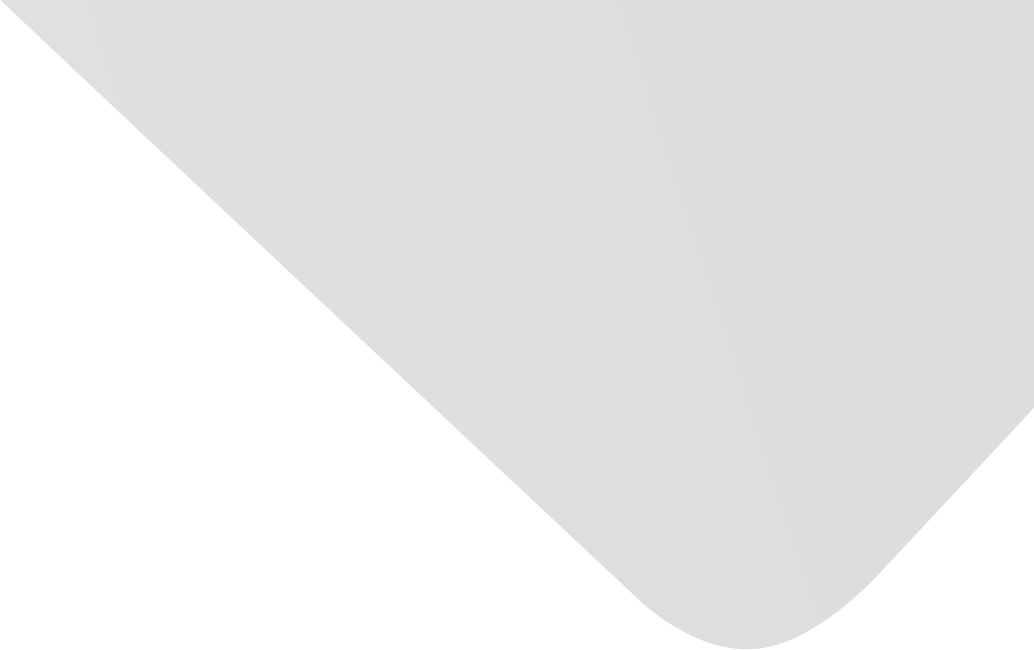
Drug-Target Interaction Prediction via Dual Laplacian Graph Regularized Matrix Completion
المؤلفون المشاركون
Wang, Minhui
Tang, Chang
Chen, Jiajia
المصدر
العدد
المجلد 2018، العدد 2018 (31 ديسمبر/كانون الأول 2018)، ص ص. 1-12، 12ص.
الناشر
Hindawi Publishing Corporation
تاريخ النشر
2018-12-02
دولة النشر
مصر
عدد الصفحات
12
التخصصات الرئيسية
الملخص EN
Drug-target interactions play an important role for biomedical drug discovery and development.
However, it is expensive and time-consuming to accomplish this task by experimental determination.
Therefore, developing computational techniques for drug-target interaction prediction is urgent and has practical significance.
In this work, we propose an effective computational model of dual Laplacian graph regularized matrix completion, referred to as DLGRMC briefly, to infer the unknown drug-target interactions.
Specifically, DLGRMC transforms the task of drug-target interaction prediction into a matrix completion problem, in which the potential interactions between drugs and targets can be obtained based on the prediction scores after the matrix completion procedure.
In DLGRMC, the drug pairwise chemical structure similarities and the target pairwise genomic sequence similarities are fully exploited to serve the matrix completion by using a dual Laplacian graph regularization term; i.e., drugs with similar chemical structure are more likely to have interactions with similar targets and targets with similar genomic sequence similarity are more likely to have interactions with similar drugs.
In addition, during the matrix completion process, an indicator matrix with binary values which indicates the indices of the observed drug-target interactions is deployed to preserve the experimental confirmed interactions.
Furthermore, we develop an alternative iterative strategy to solve the constrained matrix completion problem based on Augmented Lagrange Multiplier algorithm.
We evaluate DLGRMC on five benchmark datasets and the results show that DLGRMC outperforms several state-of-the-art approaches in terms of 10-fold cross validation based AUPR values and PR curves.
In addition, case studies also demonstrate that DLGRMC can successfully predict most of the experimental validated drug-target interactions.
نمط استشهاد جمعية علماء النفس الأمريكية (APA)
Wang, Minhui& Tang, Chang& Chen, Jiajia. 2018. Drug-Target Interaction Prediction via Dual Laplacian Graph Regularized Matrix Completion. BioMed Research International،Vol. 2018, no. 2018, pp.1-12.
https://search.emarefa.net/detail/BIM-1124309
نمط استشهاد الجمعية الأمريكية للغات الحديثة (MLA)
Wang, Minhui…[et al.]. Drug-Target Interaction Prediction via Dual Laplacian Graph Regularized Matrix Completion. BioMed Research International No. 2018 (2018), pp.1-12.
https://search.emarefa.net/detail/BIM-1124309
نمط استشهاد الجمعية الطبية الأمريكية (AMA)
Wang, Minhui& Tang, Chang& Chen, Jiajia. Drug-Target Interaction Prediction via Dual Laplacian Graph Regularized Matrix Completion. BioMed Research International. 2018. Vol. 2018, no. 2018, pp.1-12.
https://search.emarefa.net/detail/BIM-1124309
نوع البيانات
مقالات
لغة النص
الإنجليزية
الملاحظات
Includes bibliographical references
رقم السجل
BIM-1124309
قاعدة معامل التأثير والاستشهادات المرجعية العربي "ارسيف Arcif"
أضخم قاعدة بيانات عربية للاستشهادات المرجعية للمجلات العلمية المحكمة الصادرة في العالم العربي
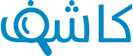
تقوم هذه الخدمة بالتحقق من التشابه أو الانتحال في الأبحاث والمقالات العلمية والأطروحات الجامعية والكتب والأبحاث باللغة العربية، وتحديد درجة التشابه أو أصالة الأعمال البحثية وحماية ملكيتها الفكرية. تعرف اكثر
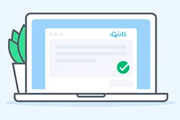