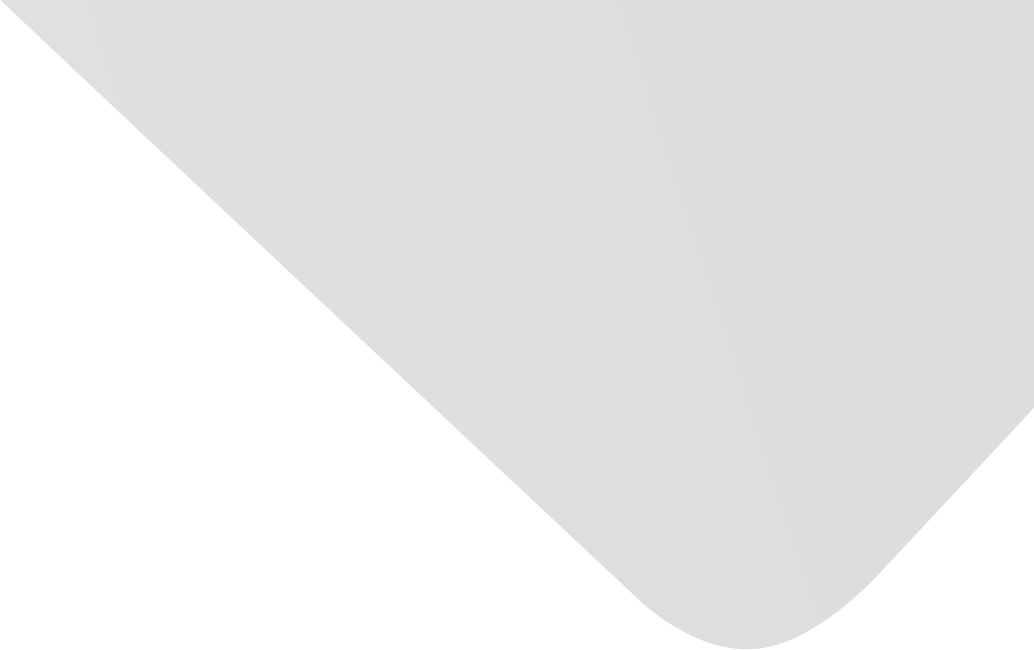
Application of Machine Learning Techniques for Clinical Predictive Modeling: A Cross-Sectional Study on Nonalcoholic Fatty Liver Disease in China
المؤلفون المشاركون
Ma, Han
Li, Youming
Yu, Chaohui
Shen, Zhe
Li, You-ming
المصدر
العدد
المجلد 2018، العدد 2018 (31 ديسمبر/كانون الأول 2018)، ص ص. 1-9، 9ص.
الناشر
Hindawi Publishing Corporation
تاريخ النشر
2018-10-03
دولة النشر
مصر
عدد الصفحات
9
التخصصات الرئيسية
الملخص EN
Background.
Nonalcoholic fatty liver disease (NAFLD) is one of the most common chronic liver diseases.
Machine learning techniques were introduced to evaluate the optimal predictive clinical model of NAFLD.
Methods.
A cross-sectional study was performed with subjects who attended a health examination at the First Affiliated Hospital, Zhejiang University.
Questionnaires, laboratory tests, physical examinations, and liver ultrasonography were employed.
Machine learning techniques were then implemented using the open source software Weka.
The tasks included feature selection and classification.
Feature selection techniques built a screening model by removing the redundant features.
Classification was used to build a prediction model, which was evaluated by the F-measure.
11 state-of-the-art machine learning techniques were investigated.
Results.
Among the 10,508 enrolled subjects, 2,522 (24%) met the diagnostic criteria of NAFLD.
By leveraging a set of statistical testing techniques, BMI, triglycerides, gamma-glutamyl transpeptidase (γGT), the serum alanine aminotransferase (ALT), and uric acid were the top 5 features contributing to NAFLD.
A 10-fold cross-validation was used in the classification.
According to the results, the Bayesian network model demonstrated the best performance from among the 11 different techniques.
It achieved accuracy, specificity, sensitivity, and F-measure scores of up to 83%, 0.878, 0.675, and 0.655, respectively.
Compared with logistic regression, the Bayesian network model improves the F-measure score by 9.17%.
Conclusion.
Novel machine learning techniques may have screening and predictive value for NAFLD.
نمط استشهاد جمعية علماء النفس الأمريكية (APA)
Ma, Han& Li, Youming& Shen, Zhe& Yu, Chaohui& Li, You-ming. 2018. Application of Machine Learning Techniques for Clinical Predictive Modeling: A Cross-Sectional Study on Nonalcoholic Fatty Liver Disease in China. BioMed Research International،Vol. 2018, no. 2018, pp.1-9.
https://search.emarefa.net/detail/BIM-1126519
نمط استشهاد الجمعية الأمريكية للغات الحديثة (MLA)
Ma, Han…[et al.]. Application of Machine Learning Techniques for Clinical Predictive Modeling: A Cross-Sectional Study on Nonalcoholic Fatty Liver Disease in China. BioMed Research International No. 2018 (2018), pp.1-9.
https://search.emarefa.net/detail/BIM-1126519
نمط استشهاد الجمعية الطبية الأمريكية (AMA)
Ma, Han& Li, Youming& Shen, Zhe& Yu, Chaohui& Li, You-ming. Application of Machine Learning Techniques for Clinical Predictive Modeling: A Cross-Sectional Study on Nonalcoholic Fatty Liver Disease in China. BioMed Research International. 2018. Vol. 2018, no. 2018, pp.1-9.
https://search.emarefa.net/detail/BIM-1126519
نوع البيانات
مقالات
لغة النص
الإنجليزية
الملاحظات
Includes bibliographical references
رقم السجل
BIM-1126519
قاعدة معامل التأثير والاستشهادات المرجعية العربي "ارسيف Arcif"
أضخم قاعدة بيانات عربية للاستشهادات المرجعية للمجلات العلمية المحكمة الصادرة في العالم العربي
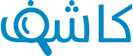
تقوم هذه الخدمة بالتحقق من التشابه أو الانتحال في الأبحاث والمقالات العلمية والأطروحات الجامعية والكتب والأبحاث باللغة العربية، وتحديد درجة التشابه أو أصالة الأعمال البحثية وحماية ملكيتها الفكرية. تعرف اكثر
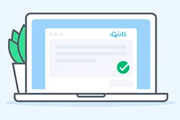