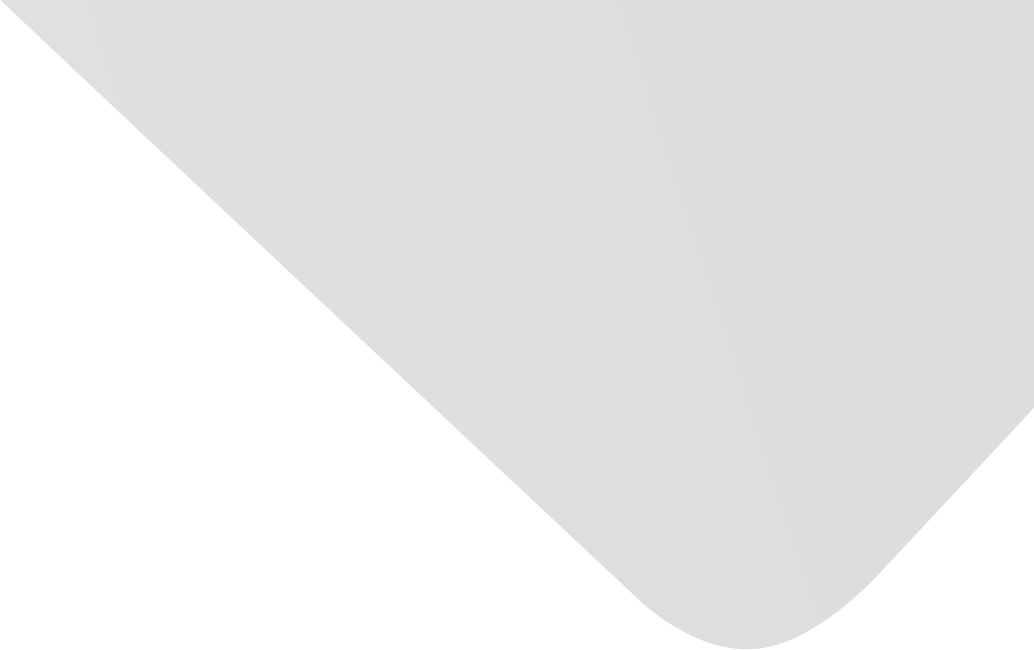
A Novel Computer-Aided Diagnosis Scheme on Small Annotated Set: G2C-CAD
المؤلفون المشاركون
Qadeer, Nouman
Zheng, Guangyuan
Han, Guanghui
Ma, Linjuan
Zhang, Fuquan
Zhao, Yanfeng
Zhao, Xinming
Zhou, Chunwu
المصدر
العدد
المجلد 2019، العدد 2019 (31 ديسمبر/كانون الأول 2019)، ص ص. 1-14، 14ص.
الناشر
Hindawi Publishing Corporation
تاريخ النشر
2019-04-15
دولة النشر
مصر
عدد الصفحات
14
التخصصات الرئيسية
الملخص EN
Purpose.
Computer-aided diagnosis (CAD) can aid in improving diagnostic level; however, the main problem currently faced by CAD is that it cannot obtain sufficient labeled samples.
To solve this problem, in this study, we adopt a generative adversarial network (GAN) approach and design a semisupervised learning algorithm, named G2C-CAD.
Methods.
From the National Cancer Institute (NCI) Lung Image Database Consortium (LIDC) dataset, we extracted four types of pulmonary nodule sign images closely related to lung cancer: noncentral calcification, lobulation, spiculation, and nonsolid/ground-glass opacity (GGO) texture, obtaining a total of 3,196 samples.
In addition, we randomly selected 2,000 non-lesion image blocks as negative samples.
We split the data 90% for training and 10% for testing.
We designed a DCGAN generative adversarial framework and trained it on the small sample set.
We also trained our designed CNN-based fuzzy Co-forest on the labeled small sample set and obtained a preliminary classifier.
Then, coupled with the simulated unlabeled samples generated by the trained DCGAN, we conducted iterative semisupervised learning, which continually improved the classification performance of the fuzzy Co-forest until the termination condition was reached.
Finally, we tested the fuzzy Co-forest and compared its performance with that of a C4.5 random decision forest and the G2C-CAD system without the fuzzy scheme, using ROC and confusion matrix for evaluation.
Results.
Four different types of lung cancer-related signs were used in the classification experiment: noncentral calcification, lobulation, spiculation, and nonsolid/ground-glass opacity (GGO) texture, along with negative image samples.
For these five classes, the G2C-CAD system obtained AUCs of 0.946, 0.912, 0.908, 0.887, and 0.939, respectively.
The average accuracy of G2C-CAD exceeded that of the C4.5 random decision tree by 14%.
G2C-CAD also obtained promising test results on the LISS signs dataset; its AUCs for GGO, lobulation, spiculation, pleural indentation, and negative image samples were 0.972, 0.964, 0.941, 0.967, and 0.953, respectively.
Conclusion.
The experimental results show that G2C-CAD is an appropriate method for addressing the problem of insufficient labeled samples in the medical image analysis field.
Moreover, our system can be used to establish a training sample library for CAD classification diagnosis, which is important for future medical image analysis.
نمط استشهاد جمعية علماء النفس الأمريكية (APA)
Zheng, Guangyuan& Han, Guanghui& Qadeer, Nouman& Ma, Linjuan& Zhang, Fuquan& Zhao, Yanfeng…[et al.]. 2019. A Novel Computer-Aided Diagnosis Scheme on Small Annotated Set: G2C-CAD. BioMed Research International،Vol. 2019, no. 2019, pp.1-14.
https://search.emarefa.net/detail/BIM-1126768
نمط استشهاد الجمعية الأمريكية للغات الحديثة (MLA)
Zheng, Guangyuan…[et al.]. A Novel Computer-Aided Diagnosis Scheme on Small Annotated Set: G2C-CAD. BioMed Research International No. 2019 (2019), pp.1-14.
https://search.emarefa.net/detail/BIM-1126768
نمط استشهاد الجمعية الطبية الأمريكية (AMA)
Zheng, Guangyuan& Han, Guanghui& Qadeer, Nouman& Ma, Linjuan& Zhang, Fuquan& Zhao, Yanfeng…[et al.]. A Novel Computer-Aided Diagnosis Scheme on Small Annotated Set: G2C-CAD. BioMed Research International. 2019. Vol. 2019, no. 2019, pp.1-14.
https://search.emarefa.net/detail/BIM-1126768
نوع البيانات
مقالات
لغة النص
الإنجليزية
الملاحظات
Includes bibliographical references
رقم السجل
BIM-1126768
قاعدة معامل التأثير والاستشهادات المرجعية العربي "ارسيف Arcif"
أضخم قاعدة بيانات عربية للاستشهادات المرجعية للمجلات العلمية المحكمة الصادرة في العالم العربي
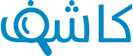
تقوم هذه الخدمة بالتحقق من التشابه أو الانتحال في الأبحاث والمقالات العلمية والأطروحات الجامعية والكتب والأبحاث باللغة العربية، وتحديد درجة التشابه أو أصالة الأعمال البحثية وحماية ملكيتها الفكرية. تعرف اكثر
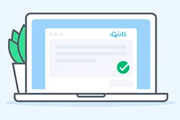