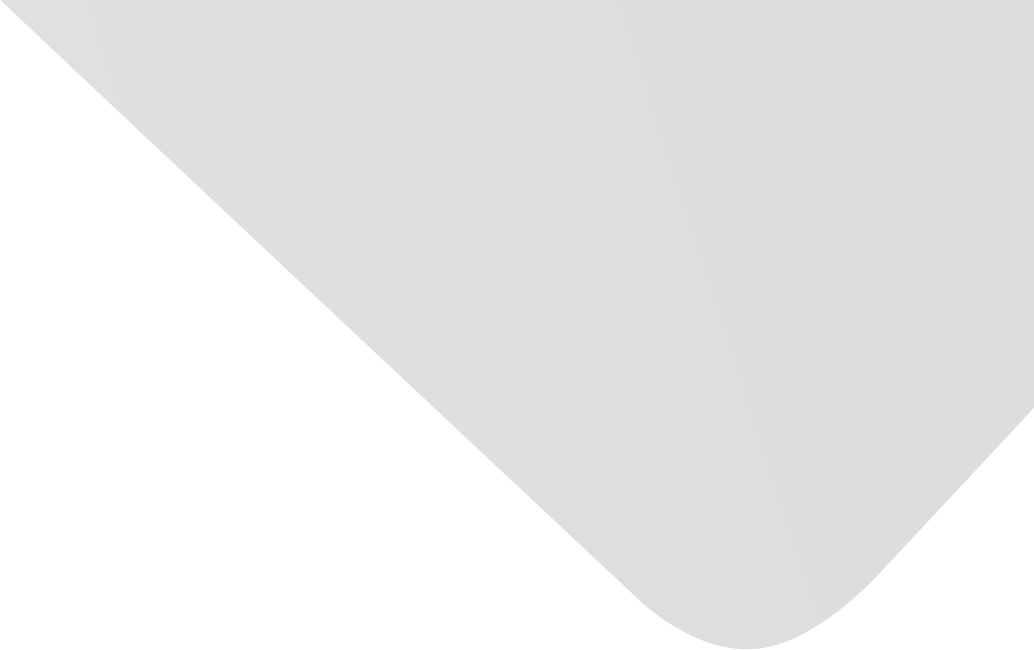
Downscaling and Projection of Multi-CMIP5 Precipitation Using Machine Learning Methods in the Upper Han River Basin
المؤلفون المشاركون
Chen, Nengcheng
Chen, Zeqiang
Xu, Ren
Chen, Yumin
المصدر
العدد
المجلد 2020، العدد 2020 (31 ديسمبر/كانون الأول 2020)، ص ص. 1-17، 17ص.
الناشر
Hindawi Publishing Corporation
تاريخ النشر
2020-03-09
دولة النشر
مصر
عدد الصفحات
17
التخصصات الرئيسية
الملخص EN
Downscaling considerably alleviates the drawbacks of regional climate simulation by general circulation models (GCMs).
However, little information is available regarding the downscaling using machine learning methods, specifically at hydrological basin scale.
This study developed multiple machine learning (ML) downscaling models, based on a Bayesian model average (BMA), to downscale the precipitation simulation of 8 Coupled Model Intercomparison Project Phase 5 (CMIP5) models using model output statistics (MOS) for the years 1961–2005 in the upper Han River basin.
A series of statistical metrics, including Pearson’s correlation coefficient (PCC), root mean squared error (RMSE), and relative bias (Rbias), were used for evaluation and comparative analyses.
Moreover, the BMA and the best ML downscaling model were used to downscale precipitation in the 21st century under Representative Concentration Pathway 4.5 (RCP4.5) and RCP8.5 scenarios.
The results show the following: (1) The performance of the BMA ensemble simulation is clearly better than that of the individual models and the simple mean model ensemble (MME).
The PCC reaches 0.74, and the RMSE is reduced by 28%–60% for all the GCMs and 33% compared to the MME.
(2) The downscaled models greatly improved station simulation performance.
Support vector machine for regression (SVR) was superior to multilayer perceptron (MLP) and random forest (RF).
The downscaling results based on the BMA ensemble simulation and SVR models were regarded as the best performing overall (PCC, RMSE, and Rbias were 0.82, 35.07, mm and −5.45%, respectively).
(3) Based on BMA and SVR models, the projected precipitations show a weak increasing trend on the whole under RCP4.5 and RCP8.5.
Specifically, the average rainfall during the mid- (2040–2069) and late (2070–2099) 21st century increased by 3.23% and 1.02%, respectively, compared to the base year (1971–2000) under RCP4.5, while they increased by 4.25% and 8.30% under RCP8.5.
Additionally, the magnitude of changes during winter and spring was higher than that during summer and autumn.
Furthermore, future work is recommended to study the improvement of downscaling models and the effect of local climate.
نمط استشهاد جمعية علماء النفس الأمريكية (APA)
Xu, Ren& Chen, Nengcheng& Chen, Yumin& Chen, Zeqiang. 2020. Downscaling and Projection of Multi-CMIP5 Precipitation Using Machine Learning Methods in the Upper Han River Basin. Advances in Meteorology،Vol. 2020, no. 2020, pp.1-17.
https://search.emarefa.net/detail/BIM-1127043
نمط استشهاد الجمعية الأمريكية للغات الحديثة (MLA)
Xu, Ren…[et al.]. Downscaling and Projection of Multi-CMIP5 Precipitation Using Machine Learning Methods in the Upper Han River Basin. Advances in Meteorology No. 2020 (2020), pp.1-17.
https://search.emarefa.net/detail/BIM-1127043
نمط استشهاد الجمعية الطبية الأمريكية (AMA)
Xu, Ren& Chen, Nengcheng& Chen, Yumin& Chen, Zeqiang. Downscaling and Projection of Multi-CMIP5 Precipitation Using Machine Learning Methods in the Upper Han River Basin. Advances in Meteorology. 2020. Vol. 2020, no. 2020, pp.1-17.
https://search.emarefa.net/detail/BIM-1127043
نوع البيانات
مقالات
لغة النص
الإنجليزية
الملاحظات
Includes bibliographical references
رقم السجل
BIM-1127043
قاعدة معامل التأثير والاستشهادات المرجعية العربي "ارسيف Arcif"
أضخم قاعدة بيانات عربية للاستشهادات المرجعية للمجلات العلمية المحكمة الصادرة في العالم العربي
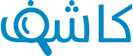
تقوم هذه الخدمة بالتحقق من التشابه أو الانتحال في الأبحاث والمقالات العلمية والأطروحات الجامعية والكتب والأبحاث باللغة العربية، وتحديد درجة التشابه أو أصالة الأعمال البحثية وحماية ملكيتها الفكرية. تعرف اكثر
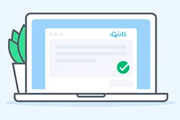