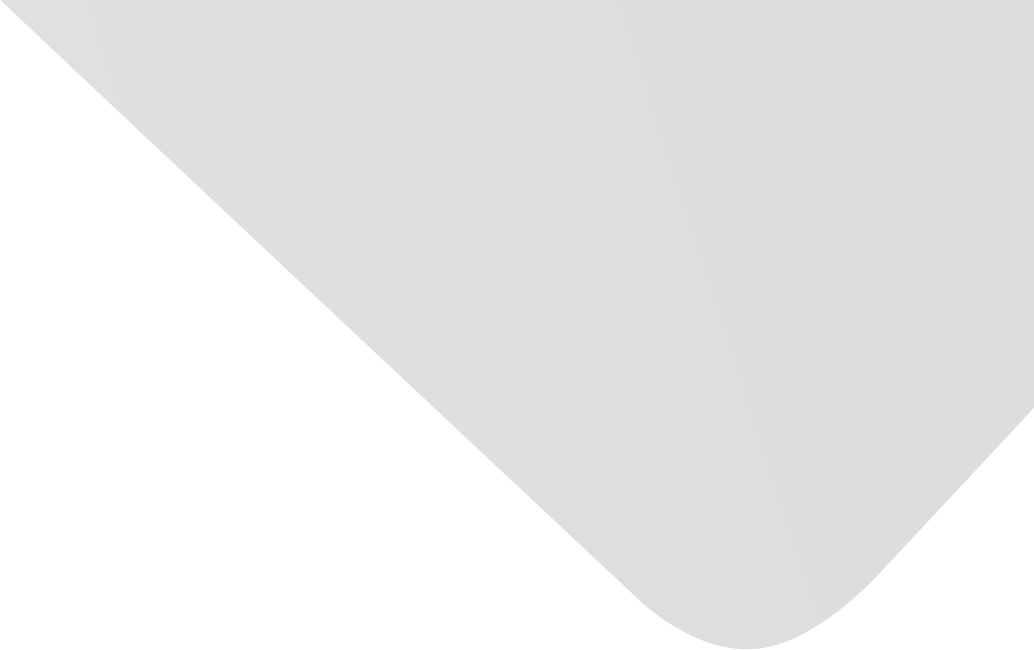
Machine Learning Readmission Risk Modeling: A Pediatric Case Study
المؤلفون المشاركون
Ríos, Sebastián A.
Graña, Manuel
Wolff, Patricio
Yarza, Maria Begoña
المصدر
العدد
المجلد 2019، العدد 2019 (31 ديسمبر/كانون الأول 2019)، ص ص. 1-9، 9ص.
الناشر
Hindawi Publishing Corporation
تاريخ النشر
2019-04-15
دولة النشر
مصر
عدد الصفحات
9
التخصصات الرئيسية
الملخص EN
Background.
Hospital readmission prediction in pediatric hospitals has received little attention.
Studies have focused on the readmission frequency analysis stratified by disease and demographic/geographic characteristics but there are no predictive modeling approaches, which may be useful to identify preventable readmissions that constitute a major portion of the cost attributed to readmissions.
Objective.
To assess the all-cause readmission predictive performance achieved by machine learning techniques in the emergency department of a pediatric hospital in Santiago, Chile.
Materials.
An all-cause admissions dataset has been collected along six consecutive years in a pediatric hospital in Santiago, Chile.
The variables collected are the same used for the determination of the child’s treatment administrative cost.
Methods.
Retrospective predictive analysis of 30-day readmission was formulated as a binary classification problem.
We report classification results achieved with various model building approaches after data curation and preprocessing for correction of class imbalance.
We compute repeated cross-validation (RCV) with decreasing number of folders to assess performance and sensitivity to effect of imbalance in the test set and training set size.
Results.
Increase in recall due to SMOTE class imbalance correction is large and statistically significant.
The Naive Bayes (NB) approach achieves the best AUC (0.65); however the shallow multilayer perceptron has the best PPV and f-score (5.6 and 10.2, resp.).
The NB and support vector machines (SVM) give comparable results if we consider AUC, PPV, and f-score ranking for all RCV experiments.
High recall of deep multilayer perceptron is due to high false positive ratio.
There is no detectable effect of the number of folds in the RCV on the predictive performance of the algorithms.
Conclusions.
We recommend the use of Naive Bayes (NB) with Gaussian distribution model as the most robust modeling approach for pediatric readmission prediction, achieving the best results across all training dataset sizes.
The results show that the approach could be applied to detect preventable readmissions.
نمط استشهاد جمعية علماء النفس الأمريكية (APA)
Wolff, Patricio& Graña, Manuel& Ríos, Sebastián A.& Yarza, Maria Begoña. 2019. Machine Learning Readmission Risk Modeling: A Pediatric Case Study. BioMed Research International،Vol. 2019, no. 2019, pp.1-9.
https://search.emarefa.net/detail/BIM-1127960
نمط استشهاد الجمعية الأمريكية للغات الحديثة (MLA)
Wolff, Patricio…[et al.]. Machine Learning Readmission Risk Modeling: A Pediatric Case Study. BioMed Research International No. 2019 (2019), pp.1-9.
https://search.emarefa.net/detail/BIM-1127960
نمط استشهاد الجمعية الطبية الأمريكية (AMA)
Wolff, Patricio& Graña, Manuel& Ríos, Sebastián A.& Yarza, Maria Begoña. Machine Learning Readmission Risk Modeling: A Pediatric Case Study. BioMed Research International. 2019. Vol. 2019, no. 2019, pp.1-9.
https://search.emarefa.net/detail/BIM-1127960
نوع البيانات
مقالات
لغة النص
الإنجليزية
الملاحظات
Includes bibliographical references
رقم السجل
BIM-1127960
قاعدة معامل التأثير والاستشهادات المرجعية العربي "ارسيف Arcif"
أضخم قاعدة بيانات عربية للاستشهادات المرجعية للمجلات العلمية المحكمة الصادرة في العالم العربي
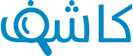
تقوم هذه الخدمة بالتحقق من التشابه أو الانتحال في الأبحاث والمقالات العلمية والأطروحات الجامعية والكتب والأبحاث باللغة العربية، وتحديد درجة التشابه أو أصالة الأعمال البحثية وحماية ملكيتها الفكرية. تعرف اكثر
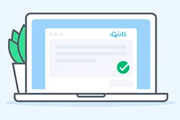