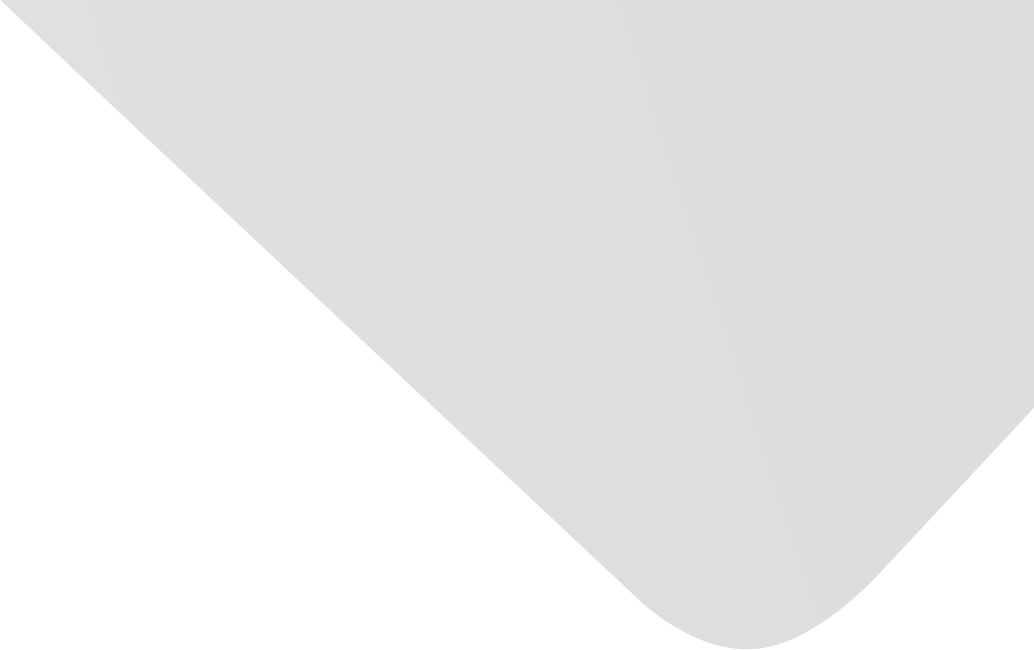
Strength Investigation of the Silt-Based Cemented Paste Backfill Using Lab Experiments and Deep Neural Network
المؤلفون المشاركون
Wang, Xinmin
Xiao, Chongchun
Wang, Yihan
Chen, Qiusong
Bin, Feng
Wei, Wei
المصدر
Advances in Materials Science and Engineering
العدد
المجلد 2020، العدد 2020 (31 ديسمبر/كانون الأول 2020)، ص ص. 1-12، 12ص.
الناشر
Hindawi Publishing Corporation
تاريخ النشر
2020-12-24
دولة النشر
مصر
عدد الصفحات
12
الملخص EN
The cemented paste backfill (CPB) technology has been successfully used for the recycling of mine tailings all around the world.
However, its application in coal mines is limited due to the lack of mine tailings that can work as aggregates.
In this work, the feasibility of using silts from the Yellow River silts (YRS) as aggregates in CPB was investigated.
Cementitious materials were selected to be the ordinary Portland cement (OPC), OPC + coal gangue (CG), and OPC + coal fly ash (CFA).
A large number of lab experiments were conducted to investigate the unconfined compressive strength (UCS) of CPB samples.
After the discussion of the experimental results, a dataset was prepared after data collection and processing.
Deep neural network (DNN) was employed to predict the UCS of CPB from its influencing variables, namely, the proportion of OPC, CG, CFA, and YS, the solids content, and the curing time.
The results show the following: (i) The solid content, cement content (cement/sand ratio), and curing time present positive correlation with UCS.
The CG can be used as a kind of OPC substitute, while adding CFA increases the UCS of CPB significantly.
(ii) The optimum training set size was 80% and the number of runs was 36 to obtain the converged results.
(iii) GA was efficient at the DNN architecture tuning with the optimum DNN architecture being found at the 17th iteration.
(iv) The optimum DNN had an excellent performance on the UCS prediction of silt-based CPB (correlation coefficient was 0.97 on the training set and 0.99 on the testing set).
(v) The curing time, the CFA proportion, and the solids content were the most significant input variables for the silt-based CPB and all of them were positively correlated with the UCS.
نمط استشهاد جمعية علماء النفس الأمريكية (APA)
Xiao, Chongchun& Wang, Xinmin& Chen, Qiusong& Bin, Feng& Wang, Yihan& Wei, Wei. 2020. Strength Investigation of the Silt-Based Cemented Paste Backfill Using Lab Experiments and Deep Neural Network. Advances in Materials Science and Engineering،Vol. 2020, no. 2020, pp.1-12.
https://search.emarefa.net/detail/BIM-1128907
نمط استشهاد الجمعية الأمريكية للغات الحديثة (MLA)
Xiao, Chongchun…[et al.]. Strength Investigation of the Silt-Based Cemented Paste Backfill Using Lab Experiments and Deep Neural Network. Advances in Materials Science and Engineering No. 2020 (2020), pp.1-12.
https://search.emarefa.net/detail/BIM-1128907
نمط استشهاد الجمعية الطبية الأمريكية (AMA)
Xiao, Chongchun& Wang, Xinmin& Chen, Qiusong& Bin, Feng& Wang, Yihan& Wei, Wei. Strength Investigation of the Silt-Based Cemented Paste Backfill Using Lab Experiments and Deep Neural Network. Advances in Materials Science and Engineering. 2020. Vol. 2020, no. 2020, pp.1-12.
https://search.emarefa.net/detail/BIM-1128907
نوع البيانات
مقالات
لغة النص
الإنجليزية
الملاحظات
Includes bibliographical references
رقم السجل
BIM-1128907
قاعدة معامل التأثير والاستشهادات المرجعية العربي "ارسيف Arcif"
أضخم قاعدة بيانات عربية للاستشهادات المرجعية للمجلات العلمية المحكمة الصادرة في العالم العربي
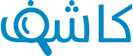
تقوم هذه الخدمة بالتحقق من التشابه أو الانتحال في الأبحاث والمقالات العلمية والأطروحات الجامعية والكتب والأبحاث باللغة العربية، وتحديد درجة التشابه أو أصالة الأعمال البحثية وحماية ملكيتها الفكرية. تعرف اكثر
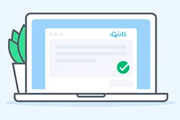