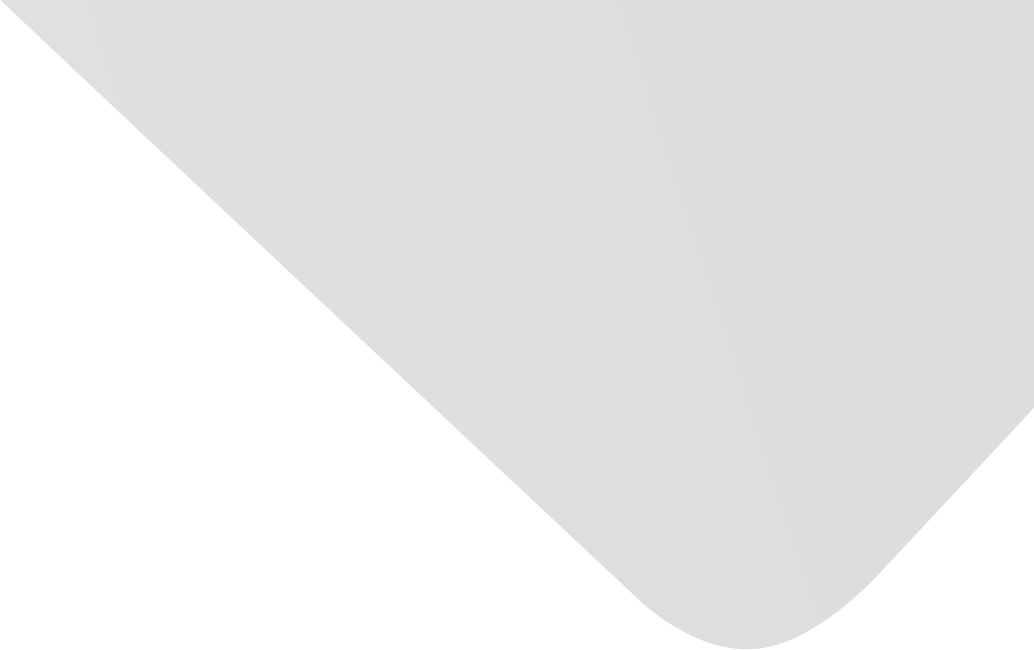
An Improved Convolutional Neural Network Algorithm and Its Application in Multilabel Image Labeling
المؤلفون المشاركون
Cao, Jianfang
Chen, Lichao
Wu, Chenyan
Cui, Hongyan
Feng, Guoqing
المصدر
Computational Intelligence and Neuroscience
العدد
المجلد 2019، العدد 2019 (31 ديسمبر/كانون الأول 2019)، ص ص. 1-12، 12ص.
الناشر
Hindawi Publishing Corporation
تاريخ النشر
2019-07-04
دولة النشر
مصر
عدد الصفحات
12
التخصصات الرئيسية
الملخص EN
In today’s society, image resources are everywhere, and the number of available images can be overwhelming.
Determining how to rapidly and effectively query, retrieve, and organize image information has become a popular research topic, and automatic image annotation is the key to text-based image retrieval.
If the semantic images with annotations are not balanced among the training samples, the low-frequency labeling accuracy can be poor.
In this study, a dual-channel convolution neural network (DCCNN) was designed to improve the accuracy of automatic labeling.
The model integrates two convolutional neural network (CNN) channels with different structures.
One channel is used for training based on the low-frequency samples and increases the proportion of low-frequency samples in the model, and the other is used for training based on all training sets.
In the labeling process, the outputs of the two channels are fused to obtain a labeling decision.
We verified the proposed model on the Caltech-256, Pascal VOC 2007, and Pascal VOC 2012 standard datasets.
On the Pascal VOC 2012 dataset, the proposed DCCNN model achieves an overall labeling accuracy of up to 93.4% after 100 training iterations: 8.9% higher than the CNN and 15% higher than the traditional method.
A similar accuracy can be achieved by the CNN only after 2,500 training iterations.
On the 50,000-image dataset from Caltech-256 and Pascal VOC 2012, the performance of the DCCNN is relatively stable; it achieves an average labeling accuracy above 93%.
In contrast, the CNN reaches an accuracy of only 91% even after extended training.
Furthermore, the proposed DCCNN achieves a labeling accuracy for low-frequency words approximately 10% higher than that of the CNN, which further verifies the reliability of the proposed model in this study.
نمط استشهاد جمعية علماء النفس الأمريكية (APA)
Cao, Jianfang& Wu, Chenyan& Chen, Lichao& Cui, Hongyan& Feng, Guoqing. 2019. An Improved Convolutional Neural Network Algorithm and Its Application in Multilabel Image Labeling. Computational Intelligence and Neuroscience،Vol. 2019, no. 2019, pp.1-12.
https://search.emarefa.net/detail/BIM-1129371
نمط استشهاد الجمعية الأمريكية للغات الحديثة (MLA)
Cao, Jianfang…[et al.]. An Improved Convolutional Neural Network Algorithm and Its Application in Multilabel Image Labeling. Computational Intelligence and Neuroscience No. 2019 (2019), pp.1-12.
https://search.emarefa.net/detail/BIM-1129371
نمط استشهاد الجمعية الطبية الأمريكية (AMA)
Cao, Jianfang& Wu, Chenyan& Chen, Lichao& Cui, Hongyan& Feng, Guoqing. An Improved Convolutional Neural Network Algorithm and Its Application in Multilabel Image Labeling. Computational Intelligence and Neuroscience. 2019. Vol. 2019, no. 2019, pp.1-12.
https://search.emarefa.net/detail/BIM-1129371
نوع البيانات
مقالات
لغة النص
الإنجليزية
الملاحظات
Includes bibliographical references
رقم السجل
BIM-1129371
قاعدة معامل التأثير والاستشهادات المرجعية العربي "ارسيف Arcif"
أضخم قاعدة بيانات عربية للاستشهادات المرجعية للمجلات العلمية المحكمة الصادرة في العالم العربي
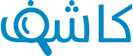
تقوم هذه الخدمة بالتحقق من التشابه أو الانتحال في الأبحاث والمقالات العلمية والأطروحات الجامعية والكتب والأبحاث باللغة العربية، وتحديد درجة التشابه أو أصالة الأعمال البحثية وحماية ملكيتها الفكرية. تعرف اكثر
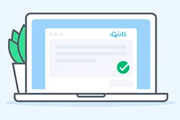