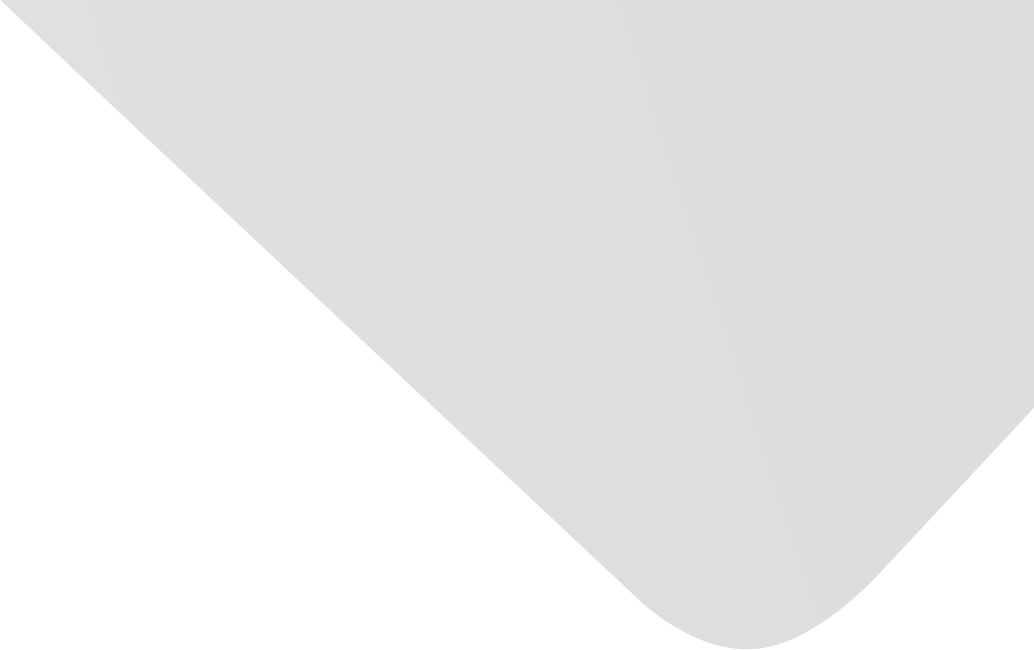
Deep Learning Application for Predicting Soil Organic Matter Content by VIS-NIR Spectroscopy
المؤلفون المشاركون
Xu, Zhe
Zhao, Xiaomin
Guo, Xi
Guo, Jiaxin
المصدر
Computational Intelligence and Neuroscience
العدد
المجلد 2019، العدد 2019 (31 ديسمبر/كانون الأول 2019)، ص ص. 1-11، 11ص.
الناشر
Hindawi Publishing Corporation
تاريخ النشر
2019-11-29
دولة النشر
مصر
عدد الصفحات
11
التخصصات الرئيسية
الملخص EN
Deep learning is characterized by its strong ability of data feature extraction.
This method can provide unique advantages when applying it to visible and near-infrared spectroscopy for predicting soil organic matter (SOM) content in those cases where the SOM content is negatively correlated with the spectral reflectance of soil.
This study relied on the SOM content data of 248 red soil samples and their spectral reflectance data of 400–2450 nm in Fengxin County, Jiangxi Province (China) to meet three objectives.
First, a multilayer perceptron and two convolutional neural networks (LeNet5 and DenseNet10) were used to predict the SOM content based on spectral variation and variable selection, and the outcomes were compared with that from the traditional back-propagation neural network (BPN).
Second, the four methods were applied to full-spectrum modeling to test the difference to selected feature variables.
Finally, the potential of direct modeling was evaluated using spectral reflectance data without any spectral variation.
The results of prediction accuracy showed that deep learning performed better at predicting the SOM content than did the traditional BPN.
Based on full-spectrum data, deep learning was able to obtain more feature information, thus achieving better and more stable results (i.e., similar average accuracy and far lower standard deviation) than those obtained through variable selection.
DenseNet achieved the best prediction result, with a coefficient of determination (R2) = 0.892 ± 0.004 and a ratio of performance to deviation (RPD) = 3.053 ± 0.056 in validation.
Based on DenseNet, the application of spectral reflectance data (without spectral variation) produced robust results for application-level purposes (validation R2 = 0.853 ± 0.007 and validation RPD = 2.639 ± 0.056).
In conclusion, deep learning provides an effective approach to predict the SOM content by visible and near-infrared spectroscopy and DenseNet is a promising method for reducing the amount of data preprocessing.
نمط استشهاد جمعية علماء النفس الأمريكية (APA)
Xu, Zhe& Zhao, Xiaomin& Guo, Xi& Guo, Jiaxin. 2019. Deep Learning Application for Predicting Soil Organic Matter Content by VIS-NIR Spectroscopy. Computational Intelligence and Neuroscience،Vol. 2019, no. 2019, pp.1-11.
https://search.emarefa.net/detail/BIM-1129415
نمط استشهاد الجمعية الأمريكية للغات الحديثة (MLA)
Xu, Zhe…[et al.]. Deep Learning Application for Predicting Soil Organic Matter Content by VIS-NIR Spectroscopy. Computational Intelligence and Neuroscience No. 2019 (2019), pp.1-11.
https://search.emarefa.net/detail/BIM-1129415
نمط استشهاد الجمعية الطبية الأمريكية (AMA)
Xu, Zhe& Zhao, Xiaomin& Guo, Xi& Guo, Jiaxin. Deep Learning Application for Predicting Soil Organic Matter Content by VIS-NIR Spectroscopy. Computational Intelligence and Neuroscience. 2019. Vol. 2019, no. 2019, pp.1-11.
https://search.emarefa.net/detail/BIM-1129415
نوع البيانات
مقالات
لغة النص
الإنجليزية
الملاحظات
Includes bibliographical references
رقم السجل
BIM-1129415
قاعدة معامل التأثير والاستشهادات المرجعية العربي "ارسيف Arcif"
أضخم قاعدة بيانات عربية للاستشهادات المرجعية للمجلات العلمية المحكمة الصادرة في العالم العربي

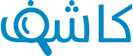
تقوم هذه الخدمة بالتحقق من التشابه أو الانتحال في الأبحاث والمقالات العلمية والأطروحات الجامعية والكتب والأبحاث باللغة العربية، وتحديد درجة التشابه أو أصالة الأعمال البحثية وحماية ملكيتها الفكرية. تعرف اكثر
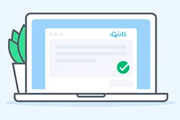