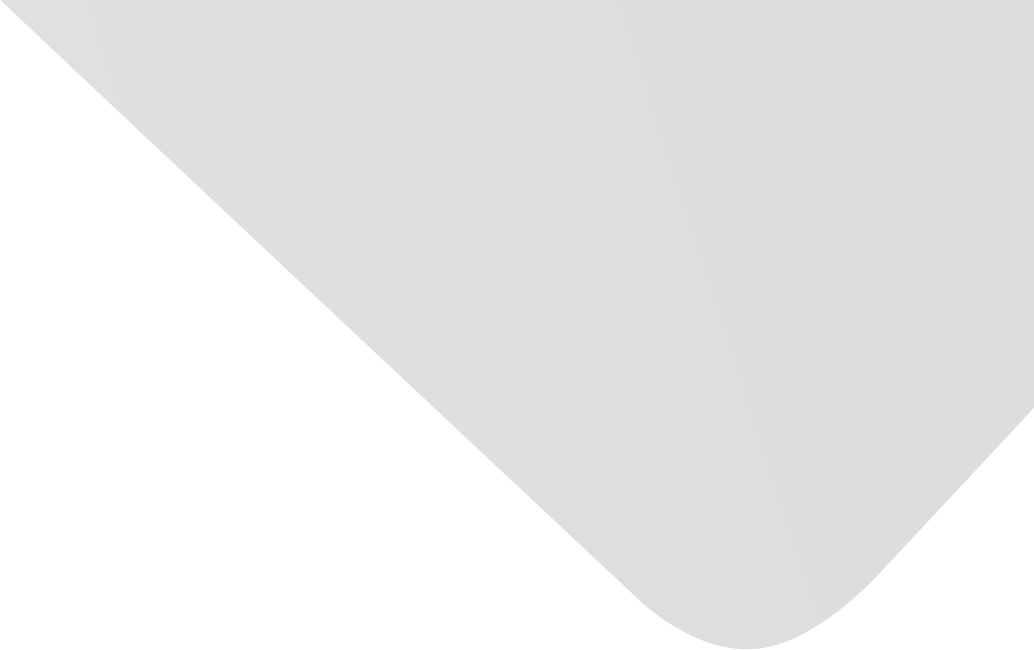
An Incremental Version of L-MVU for the Feature Extraction of MI-EEG
المؤلفون المشاركون
Li, Mingai
Xi, Hongwei
Zhu, Xiaoqing
المصدر
Computational Intelligence and Neuroscience
العدد
المجلد 2019، العدد 2019 (31 ديسمبر/كانون الأول 2019)، ص ص. 1-19، 19ص.
الناشر
Hindawi Publishing Corporation
تاريخ النشر
2019-05-02
دولة النشر
مصر
عدد الصفحات
19
التخصصات الرئيسية
الملخص EN
Due to the nonlinear and high-dimensional characteristics of motor imagery electroencephalography (MI-EEG), it can be challenging to get high online accuracy.
As a nonlinear dimension reduction method, landmark maximum variance unfolding (L-MVU) can completely retain the nonlinear features of MI-EEG.
However, L-MVU still requires considerable computation costs for out-of-sample data.
An incremental version of L-MVU (denoted as IL-MVU) is proposed in this paper.
The low-dimensional representation of the training data is generated by L-MVU.
For each out-of-sample data, its nearest neighbors will be found in the high-dimensional training samples and the corresponding reconstruction weight matrix be calculated to generate its low-dimensional representation as well.
IL-MVU is further combined with the dual-tree complex wavelet transform (DTCWT), which develops a hybrid feature extraction method (named as IL-MD).
IL-MVU is applied to extract the nonlinear features of the specific subband signals, which are reconstructed by DTCWT and have the obvious event-related synchronization/event-related desynchronization phenomenon.
The average energy features of α and β waves are calculated simultaneously.
The two types of features are fused and are evaluated by a linear discriminant analysis classifier.
Based on the two public datasets with 12 subjects, extensive experiments were conducted.
The average recognition accuracies of 10-fold cross-validation are 92.50% on Dataset 3b and 88.13% on Dataset 2b, and they gain at least 1.43% and 3.45% improvement, respectively, compared to existing methods.
The experimental results show that IL-MD can extract more accurate features with relatively lower consumption cost, and it also has better feature visualization and self-adaptive characteristics to subjects.
The t-test results and Kappa values suggest the proposed feature extraction method reaches statistical significance and has high consistency in classification.
نمط استشهاد جمعية علماء النفس الأمريكية (APA)
Li, Mingai& Xi, Hongwei& Zhu, Xiaoqing. 2019. An Incremental Version of L-MVU for the Feature Extraction of MI-EEG. Computational Intelligence and Neuroscience،Vol. 2019, no. 2019, pp.1-19.
https://search.emarefa.net/detail/BIM-1129452
نمط استشهاد الجمعية الأمريكية للغات الحديثة (MLA)
Li, Mingai…[et al.]. An Incremental Version of L-MVU for the Feature Extraction of MI-EEG. Computational Intelligence and Neuroscience No. 2019 (2019), pp.1-19.
https://search.emarefa.net/detail/BIM-1129452
نمط استشهاد الجمعية الطبية الأمريكية (AMA)
Li, Mingai& Xi, Hongwei& Zhu, Xiaoqing. An Incremental Version of L-MVU for the Feature Extraction of MI-EEG. Computational Intelligence and Neuroscience. 2019. Vol. 2019, no. 2019, pp.1-19.
https://search.emarefa.net/detail/BIM-1129452
نوع البيانات
مقالات
لغة النص
الإنجليزية
الملاحظات
Includes bibliographical references
رقم السجل
BIM-1129452
قاعدة معامل التأثير والاستشهادات المرجعية العربي "ارسيف Arcif"
أضخم قاعدة بيانات عربية للاستشهادات المرجعية للمجلات العلمية المحكمة الصادرة في العالم العربي
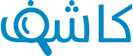
تقوم هذه الخدمة بالتحقق من التشابه أو الانتحال في الأبحاث والمقالات العلمية والأطروحات الجامعية والكتب والأبحاث باللغة العربية، وتحديد درجة التشابه أو أصالة الأعمال البحثية وحماية ملكيتها الفكرية. تعرف اكثر
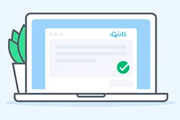