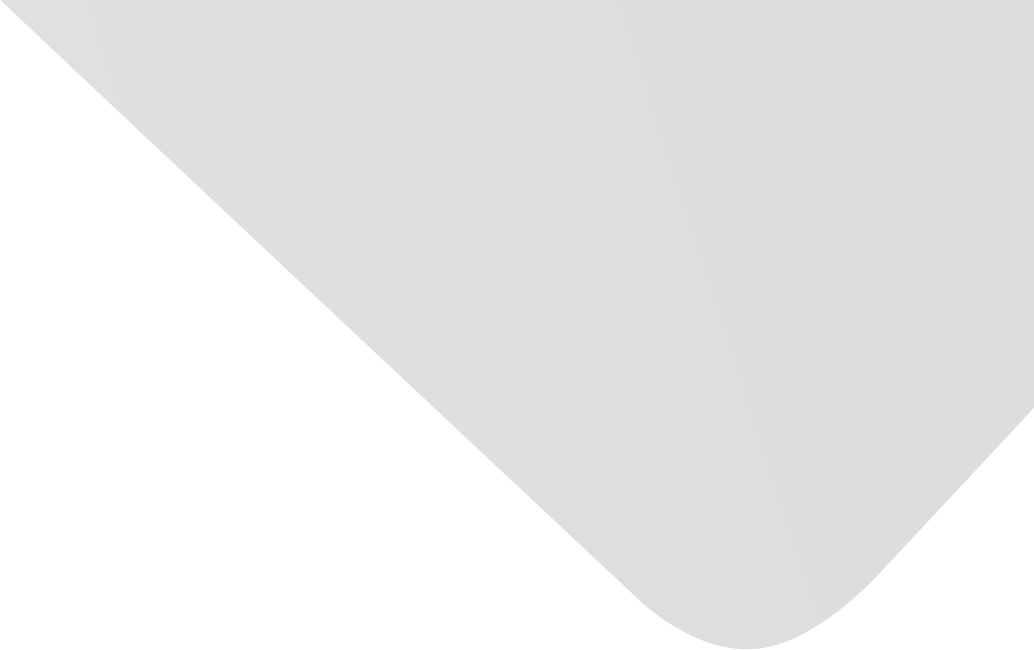
Context Attention Heterogeneous Network Embedding
المؤلفون المشاركون
Zhuo, Wei
Zhan, Qianyi
Liu, Yuan
Xie, Zhenping
Lu, Jing
المصدر
Computational Intelligence and Neuroscience
العدد
المجلد 2019، العدد 2019 (31 ديسمبر/كانون الأول 2019)، ص ص. 1-15، 15ص.
الناشر
Hindawi Publishing Corporation
تاريخ النشر
2019-08-21
دولة النشر
مصر
عدد الصفحات
15
التخصصات الرئيسية
الملخص EN
Network embedding (NE), which maps nodes into a low-dimensional latent Euclidean space to represent effective features of each node in the network, has obtained considerable attention in recent years.
Many popular NE methods, such as DeepWalk, Node2vec, and LINE, are capable of handling homogeneous networks.
However, nodes are always fully accompanied by heterogeneous information (e.g., text descriptions, node properties, and hashtags) in the real-world network, which remains a great challenge to jointly project the topological structure and different types of information into the fixed-dimensional embedding space due to heterogeneity.
Besides, in the unweighted network, how to quantify the strength of edges (tightness of connections between nodes) accurately is also a difficulty faced by existing methods.
To bridge the gap, in this paper, we propose CAHNE (context attention heterogeneous network embedding), a novel network embedding method, to accurately determine the learning result.
Specifically, we propose the concept of node importance to measure the strength of edges, which can better preserve the context relations of a node in unweighted networks.
Moreover, text information is a widely ubiquitous feature in real-world networks, e.g., online social networks and citation networks.
On account of the sophisticated interactions between the network structure and text features of nodes, CAHNE learns context embeddings for nodes by introducing the context node sequence, and the attention mechanism is also integrated into our model to better reflect the impact of context nodes on the current node.
To corroborate the efficacy of CAHNE, we apply our method and various baseline methods on several real-world datasets.
The experimental results show that CAHNE achieves higher quality compared to a number of state-of-the-art network embedding methods on the tasks of network reconstruction, link prediction, node classification, and visualization.
نمط استشهاد جمعية علماء النفس الأمريكية (APA)
Zhuo, Wei& Zhan, Qianyi& Liu, Yuan& Xie, Zhenping& Lu, Jing. 2019. Context Attention Heterogeneous Network Embedding. Computational Intelligence and Neuroscience،Vol. 2019, no. 2019, pp.1-15.
https://search.emarefa.net/detail/BIM-1129592
نمط استشهاد الجمعية الأمريكية للغات الحديثة (MLA)
Zhuo, Wei…[et al.]. Context Attention Heterogeneous Network Embedding. Computational Intelligence and Neuroscience No. 2019 (2019), pp.1-15.
https://search.emarefa.net/detail/BIM-1129592
نمط استشهاد الجمعية الطبية الأمريكية (AMA)
Zhuo, Wei& Zhan, Qianyi& Liu, Yuan& Xie, Zhenping& Lu, Jing. Context Attention Heterogeneous Network Embedding. Computational Intelligence and Neuroscience. 2019. Vol. 2019, no. 2019, pp.1-15.
https://search.emarefa.net/detail/BIM-1129592
نوع البيانات
مقالات
لغة النص
الإنجليزية
الملاحظات
Includes bibliographical references
رقم السجل
BIM-1129592
قاعدة معامل التأثير والاستشهادات المرجعية العربي "ارسيف Arcif"
أضخم قاعدة بيانات عربية للاستشهادات المرجعية للمجلات العلمية المحكمة الصادرة في العالم العربي
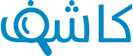
تقوم هذه الخدمة بالتحقق من التشابه أو الانتحال في الأبحاث والمقالات العلمية والأطروحات الجامعية والكتب والأبحاث باللغة العربية، وتحديد درجة التشابه أو أصالة الأعمال البحثية وحماية ملكيتها الفكرية. تعرف اكثر
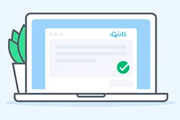