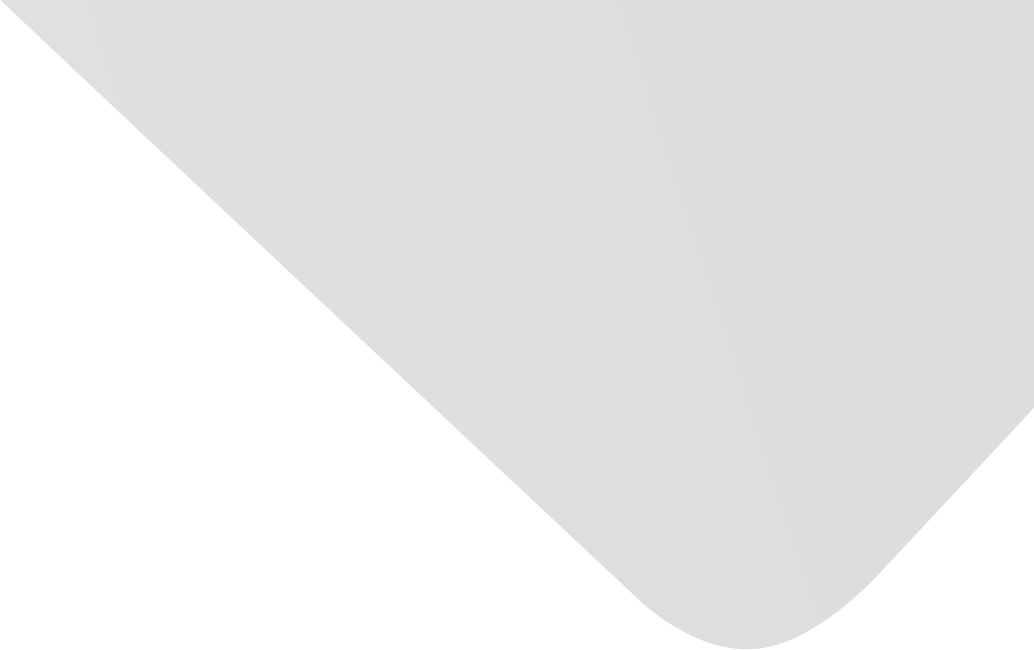
Medical Image Classification Based on Deep Features Extracted by Deep Model and Statistic Feature Fusion with Multilayer Perceptron
المؤلفون المشاركون
المصدر
Computational Intelligence and Neuroscience
العدد
المجلد 2018، العدد 2018 (31 ديسمبر/كانون الأول 2018)، ص ص. 1-13، 13ص.
الناشر
Hindawi Publishing Corporation
تاريخ النشر
2018-09-12
دولة النشر
مصر
عدد الصفحات
13
التخصصات الرئيسية
الملخص EN
Medical image classification is a key technique of Computer-Aided Diagnosis (CAD) systems.
Traditional methods rely mainly on the shape, color, and/or texture features as well as their combinations, most of which are problem-specific and have shown to be complementary in medical images, which leads to a system that lacks the ability to make representations of high-level problem domain concepts and that has poor model generalization ability.
Recent deep learning methods provide an effective way to construct an end-to-end model that can compute final classification labels with the raw pixels of medical images.
However, due to the high resolution of the medical images and the small dataset size, deep learning models suffer from high computational costs and limitations in the model layers and channels.
To solve these problems, in this paper, we propose a deep learning model that integrates Coding Network with Multilayer Perceptron (CNMP), which combines high-level features that are extracted from a deep convolutional neural network and some selected traditional features.
The construction of the proposed model includes the following steps.
First, we train a deep convolutional neural network as a coding network in a supervised manner, and the result is that it can code the raw pixels of medical images into feature vectors that represent high-level concepts for classification.
Second, we extract a set of selected traditional features based on background knowledge of medical images.
Finally, we design an efficient model that is based on neural networks to fuse the different feature groups obtained in the first and second step.
We evaluate the proposed approach on two benchmark medical image datasets: HIS2828 and ISIC2017.
We achieve an overall classification accuracy of 90.1% and 90.2%, respectively, which are higher than the current successful methods.
نمط استشهاد جمعية علماء النفس الأمريكية (APA)
Lai, ZhiFei& Deng, HuiFang. 2018. Medical Image Classification Based on Deep Features Extracted by Deep Model and Statistic Feature Fusion with Multilayer Perceptron. Computational Intelligence and Neuroscience،Vol. 2018, no. 2018, pp.1-13.
https://search.emarefa.net/detail/BIM-1130625
نمط استشهاد الجمعية الأمريكية للغات الحديثة (MLA)
Lai, ZhiFei& Deng, HuiFang. Medical Image Classification Based on Deep Features Extracted by Deep Model and Statistic Feature Fusion with Multilayer Perceptron. Computational Intelligence and Neuroscience No. 2018 (2018), pp.1-13.
https://search.emarefa.net/detail/BIM-1130625
نمط استشهاد الجمعية الطبية الأمريكية (AMA)
Lai, ZhiFei& Deng, HuiFang. Medical Image Classification Based on Deep Features Extracted by Deep Model and Statistic Feature Fusion with Multilayer Perceptron. Computational Intelligence and Neuroscience. 2018. Vol. 2018, no. 2018, pp.1-13.
https://search.emarefa.net/detail/BIM-1130625
نوع البيانات
مقالات
لغة النص
الإنجليزية
الملاحظات
Includes bibliographical references
رقم السجل
BIM-1130625
قاعدة معامل التأثير والاستشهادات المرجعية العربي "ارسيف Arcif"
أضخم قاعدة بيانات عربية للاستشهادات المرجعية للمجلات العلمية المحكمة الصادرة في العالم العربي
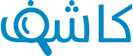
تقوم هذه الخدمة بالتحقق من التشابه أو الانتحال في الأبحاث والمقالات العلمية والأطروحات الجامعية والكتب والأبحاث باللغة العربية، وتحديد درجة التشابه أو أصالة الأعمال البحثية وحماية ملكيتها الفكرية. تعرف اكثر
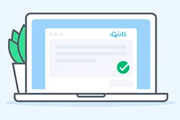