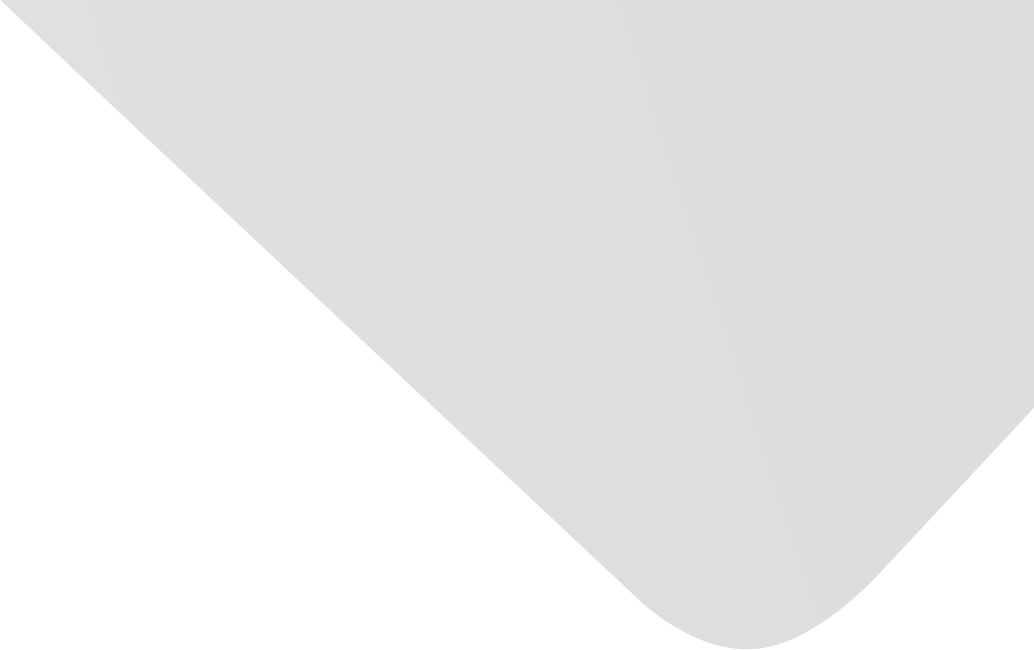
Sinc-Windowing and Multiple Correlation Coefficients Improve SSVEP Recognition Based on Canonical Correlation Analysis
المؤلفون المشاركون
Mondini, Valeria
Mangia, Anna Lisa
Cappello, Angelo
Talevi, Luca
المصدر
Computational Intelligence and Neuroscience
العدد
المجلد 2018، العدد 2018 (31 ديسمبر/كانون الأول 2018)، ص ص. 1-11، 11ص.
الناشر
Hindawi Publishing Corporation
تاريخ النشر
2018-04-12
دولة النشر
مصر
عدد الصفحات
11
التخصصات الرئيسية
الملخص EN
Canonical Correlation Analysis (CCA) is an increasingly used approach in the field of Steady-State Visually Evoked Potential (SSVEP) recognition.
The efficacy of the method has been widely proven, and several variations have been proposed.
However, most CCA variations tend to complicate the method, usually requiring additional user training or increasing computational load.
Taking simple procedures and low computational costs may be, however, a relevant aspect, especially in view of low-cost and high-portability devices.
In addition, it would be desirable that the proposed variations are as general and modular as possible to facilitate the translation of results to different algorithms and setups.
In this work, we evaluated the impact of two simple, modular variations of the classical CCA method.
The variations involved (i) the number of canonical correlations used for classification and (ii) the inclusion of a prefiltering step by means of sinc-windowing.
We tested ten volunteers in a 4-class SSVEP setup.
Both variations significantly improved classification accuracy when they were used separately or in conjunction and led to accuracy increments up to 7-8% on average and peak of 25–30%.
Additionally, variations had no (variation (i)) or minimal (variation (ii)) impact on the number of algorithm steps required for each classification.
Given the modular nature of the proposed variations and their positive impact on classification accuracy, they might be easily included in the design of CCA-based algorithms that are even different from ours.
نمط استشهاد جمعية علماء النفس الأمريكية (APA)
Mondini, Valeria& Mangia, Anna Lisa& Talevi, Luca& Cappello, Angelo. 2018. Sinc-Windowing and Multiple Correlation Coefficients Improve SSVEP Recognition Based on Canonical Correlation Analysis. Computational Intelligence and Neuroscience،Vol. 2018, no. 2018, pp.1-11.
https://search.emarefa.net/detail/BIM-1130716
نمط استشهاد الجمعية الأمريكية للغات الحديثة (MLA)
Mondini, Valeria…[et al.]. Sinc-Windowing and Multiple Correlation Coefficients Improve SSVEP Recognition Based on Canonical Correlation Analysis. Computational Intelligence and Neuroscience No. 2018 (2018), pp.1-11.
https://search.emarefa.net/detail/BIM-1130716
نمط استشهاد الجمعية الطبية الأمريكية (AMA)
Mondini, Valeria& Mangia, Anna Lisa& Talevi, Luca& Cappello, Angelo. Sinc-Windowing and Multiple Correlation Coefficients Improve SSVEP Recognition Based on Canonical Correlation Analysis. Computational Intelligence and Neuroscience. 2018. Vol. 2018, no. 2018, pp.1-11.
https://search.emarefa.net/detail/BIM-1130716
نوع البيانات
مقالات
لغة النص
الإنجليزية
الملاحظات
Includes bibliographical references
رقم السجل
BIM-1130716
قاعدة معامل التأثير والاستشهادات المرجعية العربي "ارسيف Arcif"
أضخم قاعدة بيانات عربية للاستشهادات المرجعية للمجلات العلمية المحكمة الصادرة في العالم العربي
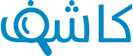
تقوم هذه الخدمة بالتحقق من التشابه أو الانتحال في الأبحاث والمقالات العلمية والأطروحات الجامعية والكتب والأبحاث باللغة العربية، وتحديد درجة التشابه أو أصالة الأعمال البحثية وحماية ملكيتها الفكرية. تعرف اكثر
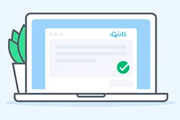