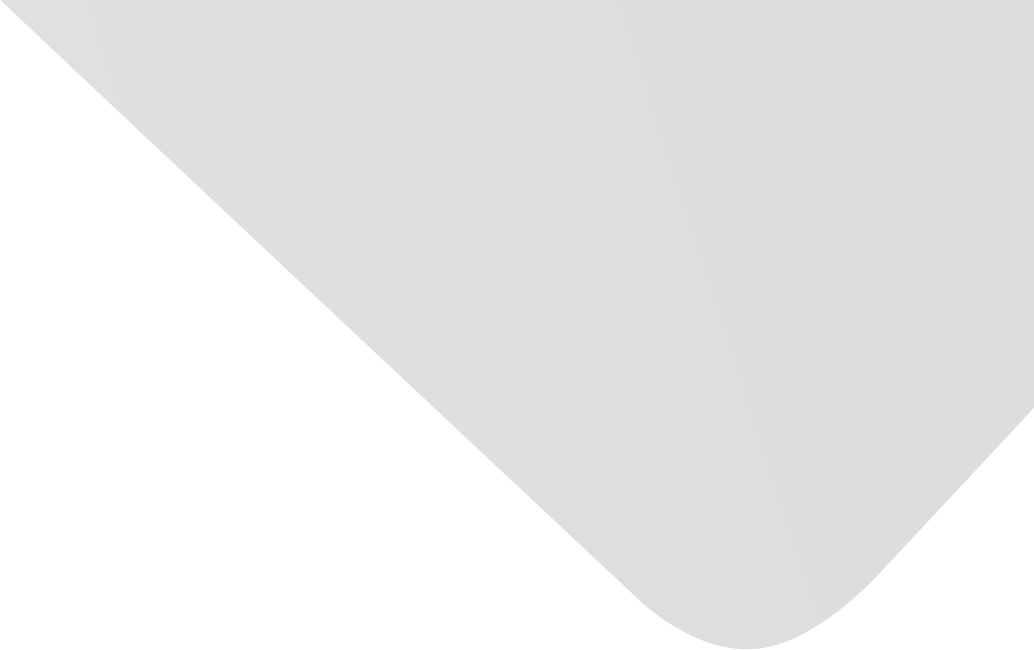
Real-Time Road Crack Mapping Using an Optimized Convolutional Neural Network
المؤلفون المشاركون
Zargarzadeh, Hassan
Naddaf-Sh, M-Mahdi
Hosseini, SeyedSaeid
Zhang, Jing
Brake, Nicholas A.
المصدر
العدد
المجلد 2019، العدد 2019 (31 ديسمبر/كانون الأول 2019)، ص ص. 1-17، 17ص.
الناشر
Hindawi Publishing Corporation
تاريخ النشر
2019-09-29
دولة النشر
مصر
عدد الصفحات
17
التخصصات الرئيسية
الملخص EN
Pavement surveying and distress mapping is completed by roadway authorities to quantify the topical and structural damage levels for strategic preventative or rehabilitative action.
The failure to time the preventative or rehabilitative action and control distress propagation can lead to severe structural and financial loss of the asset requiring complete reconstruction.
Continuous and computer-aided surveying measures not only can eliminate human error when analyzing, identifying, defining, and mapping pavement surface distresses, but also can provide a database of road damage patterns and their locations.
The database can be used for timely road repairs to gain the maximum durability of the asphalt and the minimum cost of maintenance.
This paper introduces an autonomous surveying scheme to collect, analyze, and map the image-based distress data in real time.
A descriptive approach is considered for identifying cracks from collected images using a convolutional neural network (CNN) that classifies several types of cracks.
Typically, CNN-based schemes require a relatively large processing power to detect desired objects in images in real time.
However, the portability objective of this work requires to utilize low-weight processing units.
To that end, the CNN training was optimized by the Bayesian optimization algorithm (BOA) to achieve the maximum accuracy and minimum processing time with minimum neural network layers.
First, a database consisting of a diverse population of crack distress types such as longitudinal, transverse, and alligator cracks, photographed at multiple angles, was prepared.
Then, the database was used to train a CNN whose hyperparameters were optimized using BOA.
Finally, a heuristic algorithm is introduced to process the CNN’s output and produce the crack map.
The performance of the classifier and mapping algorithm is examined against still images and videos captured by a drone from cracked pavement.
In both instances, the proposed CNN was able to classify the cracks with 97% accuracy.
The mapping algorithm is able to map a diverse population of surface cracks patterns in real time at the speed of 11.1 km per hour.
نمط استشهاد جمعية علماء النفس الأمريكية (APA)
Naddaf-Sh, M-Mahdi& Hosseini, SeyedSaeid& Zhang, Jing& Brake, Nicholas A.& Zargarzadeh, Hassan. 2019. Real-Time Road Crack Mapping Using an Optimized Convolutional Neural Network. Complexity،Vol. 2019, no. 2019, pp.1-17.
https://search.emarefa.net/detail/BIM-1131240
نمط استشهاد الجمعية الأمريكية للغات الحديثة (MLA)
Naddaf-Sh, M-Mahdi…[et al.]. Real-Time Road Crack Mapping Using an Optimized Convolutional Neural Network. Complexity No. 2019 (2019), pp.1-17.
https://search.emarefa.net/detail/BIM-1131240
نمط استشهاد الجمعية الطبية الأمريكية (AMA)
Naddaf-Sh, M-Mahdi& Hosseini, SeyedSaeid& Zhang, Jing& Brake, Nicholas A.& Zargarzadeh, Hassan. Real-Time Road Crack Mapping Using an Optimized Convolutional Neural Network. Complexity. 2019. Vol. 2019, no. 2019, pp.1-17.
https://search.emarefa.net/detail/BIM-1131240
نوع البيانات
مقالات
لغة النص
الإنجليزية
الملاحظات
Includes bibliographical references
رقم السجل
BIM-1131240
قاعدة معامل التأثير والاستشهادات المرجعية العربي "ارسيف Arcif"
أضخم قاعدة بيانات عربية للاستشهادات المرجعية للمجلات العلمية المحكمة الصادرة في العالم العربي
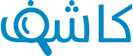
تقوم هذه الخدمة بالتحقق من التشابه أو الانتحال في الأبحاث والمقالات العلمية والأطروحات الجامعية والكتب والأبحاث باللغة العربية، وتحديد درجة التشابه أو أصالة الأعمال البحثية وحماية ملكيتها الفكرية. تعرف اكثر
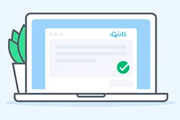