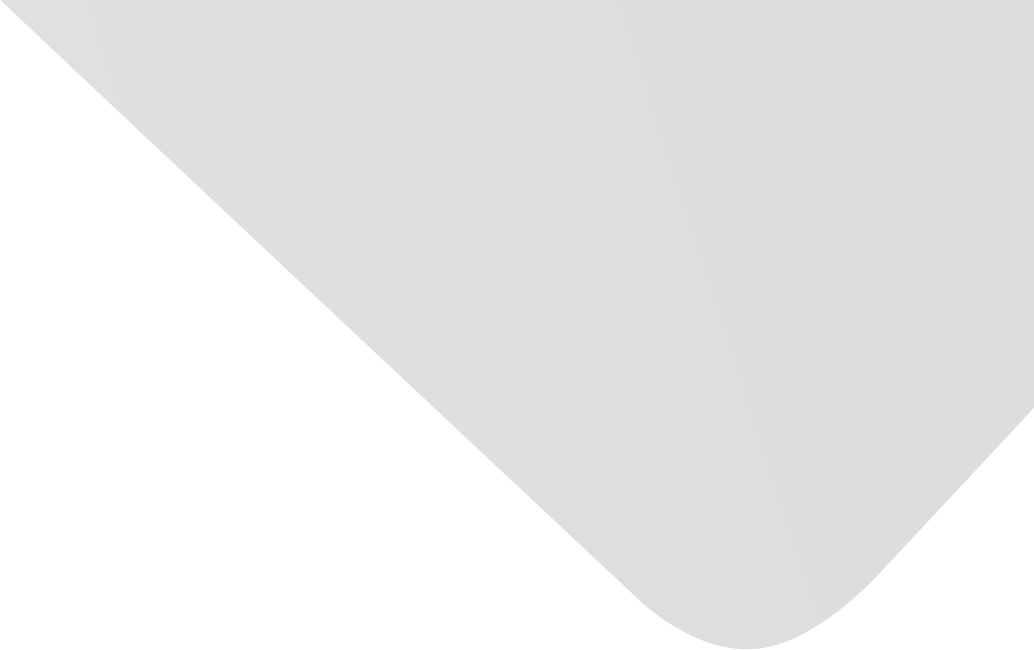
An Effective ABC-SVM Approach for Surface Roughness Prediction in Manufacturing Processes
المؤلفون المشاركون
Lu, Juan
Liao, Xiaoping
Li, Steven
Ouyang, Haibin
Chen, Kai
Huang, Bing
المصدر
العدد
المجلد 2019، العدد 2019 (31 ديسمبر/كانون الأول 2019)، ص ص. 1-13، 13ص.
الناشر
Hindawi Publishing Corporation
تاريخ النشر
2019-06-13
دولة النشر
مصر
عدد الصفحات
13
التخصصات الرئيسية
الملخص EN
It is difficult to accurately predict the response of some stochastic and complicated manufacturing processes.
Data-driven learning methods which can mine unseen relationship between influence parameters and outputs are regarded as an effective solution.
In this study, support vector machine (SVM) is applied to develop prediction models for machining processes.
Kernel function and loss function are Gaussian radial basis function and ε -insensitive loss function, respectively.
To improve the prediction accuracy and reduce parameter adjustment time of SVM model, artificial bee colony algorithm (ABC) is employed to optimize internal parameters of SVM model.
Further, to evaluate the optimization performance of ABC in parameters determination of SVM, this study compares the prediction performance of SVM models optimized by well-known evolutionary and swarm-based algorithms (differential evolution (DE), genetic algorithm (GA), particle swarm optimization (PSO), and ABC) and analyzes ability of these optimization algorithms from their optimization mechanism and convergence speed based on experimental datasets of turning and milling.
Experimental results indicate that the selected four evaluation indicators values that reflect prediction accuracy and adjustment time for ABC-SVM are better than DE-SVM, GA-SVM, and PSO-SVM except three indicator values of DE-SVM for AISI 1045 steel under the case that training set is enough to develop the prediction model.
ABC algorithm has less control parameters, faster convergence speed, and stronger searching ability than DE, GA, and PSO algorithms for optimizing the internal parameters of SVM model.
These results shed light on choosing a satisfactory optimization algorithm of SVM for manufacturing processes.
نمط استشهاد جمعية علماء النفس الأمريكية (APA)
Lu, Juan& Liao, Xiaoping& Li, Steven& Ouyang, Haibin& Chen, Kai& Huang, Bing. 2019. An Effective ABC-SVM Approach for Surface Roughness Prediction in Manufacturing Processes. Complexity،Vol. 2019, no. 2019, pp.1-13.
https://search.emarefa.net/detail/BIM-1131314
نمط استشهاد الجمعية الأمريكية للغات الحديثة (MLA)
Lu, Juan…[et al.]. An Effective ABC-SVM Approach for Surface Roughness Prediction in Manufacturing Processes. Complexity No. 2019 (2019), pp.1-13.
https://search.emarefa.net/detail/BIM-1131314
نمط استشهاد الجمعية الطبية الأمريكية (AMA)
Lu, Juan& Liao, Xiaoping& Li, Steven& Ouyang, Haibin& Chen, Kai& Huang, Bing. An Effective ABC-SVM Approach for Surface Roughness Prediction in Manufacturing Processes. Complexity. 2019. Vol. 2019, no. 2019, pp.1-13.
https://search.emarefa.net/detail/BIM-1131314
نوع البيانات
مقالات
لغة النص
الإنجليزية
الملاحظات
Includes bibliographical references
رقم السجل
BIM-1131314
قاعدة معامل التأثير والاستشهادات المرجعية العربي "ارسيف Arcif"
أضخم قاعدة بيانات عربية للاستشهادات المرجعية للمجلات العلمية المحكمة الصادرة في العالم العربي
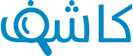
تقوم هذه الخدمة بالتحقق من التشابه أو الانتحال في الأبحاث والمقالات العلمية والأطروحات الجامعية والكتب والأبحاث باللغة العربية، وتحديد درجة التشابه أو أصالة الأعمال البحثية وحماية ملكيتها الفكرية. تعرف اكثر
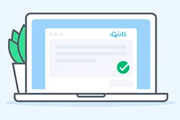