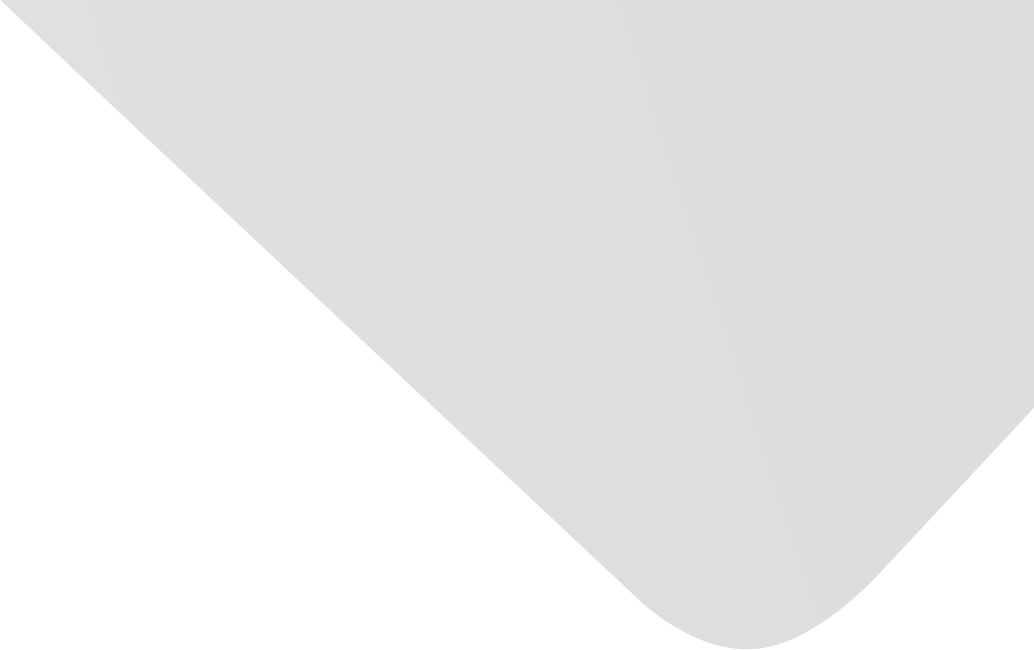
Improving Model Performance on the Stratification of Breast Cancer Patients by Integrating Multiscale Genomic Features
المؤلفون المشاركون
Wen, Zhining
Li, Menglong
Hao, Yingyi
He, Li
Zhou, Yifan
Zhao, Yiru
Jing, Runyu
المصدر
العدد
المجلد 2020، العدد 2020 (31 ديسمبر/كانون الأول 2020)، ص ص. 1-12، 12ص.
الناشر
Hindawi Publishing Corporation
تاريخ النشر
2020-08-26
دولة النشر
مصر
عدد الصفحات
12
التخصصات الرئيسية
الملخص EN
In clinical cancer research, it is a hot topic on how to accurately stratify patients based on genomic data.
With the development of next-generation sequencing technology, more and more types of genomic features, such as mRNA expression level, can be used to distinguish cancer patients.
Previous studies commonly stratified patients by using a single type of genomic features, which can only reflect one aspect of the cancer.
In fact, multiscale genomic features will provide more information and may be helpful for clinical prediction.
In addition, most of the conventional machine learning algorithms use a handcrafted gene set as features to construct models, which is generally selected by a statistical method with an arbitrary cut-off, e.g., p value < 0.05.
The genes in the gene set are not necessarily related to the cancer and will make the model unreliable.
Therefore, in our study, we thoroughly investigated the performance of different machine learning methods on stratifying breast cancer patients with a single type of genomic features.
Then, we proposed a strategy, which can take into account the degree of correlation between genes and cancer patients, to identify the features from mRNAs and microRNAs, and evaluated the performance of the models with the new combined features of the multiscale genomic features.
The results showed that, compared with the models constructed with a single type of features, the models with the multiscale genomic features generated by our proposed method achieved better performance on stratifying the ER status of breast cancer patients.
Moreover, we found that the identified multiscale genomic features were closely related to the cancer by gene set enrichment analysis, indicating that our proposed strategy can well reflect the biological relevance of the genes to breast cancer.
In conclusion, modelling with multiscale genomic features closely related to the cancer not only can guarantee the prediction performance of the models but also can effectively provide candidate genes for interpreting the mechanisms of cancer.
نمط استشهاد جمعية علماء النفس الأمريكية (APA)
Hao, Yingyi& He, Li& Zhou, Yifan& Zhao, Yiru& Li, Menglong& Jing, Runyu…[et al.]. 2020. Improving Model Performance on the Stratification of Breast Cancer Patients by Integrating Multiscale Genomic Features. BioMed Research International،Vol. 2020, no. 2020, pp.1-12.
https://search.emarefa.net/detail/BIM-1131670
نمط استشهاد الجمعية الأمريكية للغات الحديثة (MLA)
Hao, Yingyi…[et al.]. Improving Model Performance on the Stratification of Breast Cancer Patients by Integrating Multiscale Genomic Features. BioMed Research International No. 2020 (2020), pp.1-12.
https://search.emarefa.net/detail/BIM-1131670
نمط استشهاد الجمعية الطبية الأمريكية (AMA)
Hao, Yingyi& He, Li& Zhou, Yifan& Zhao, Yiru& Li, Menglong& Jing, Runyu…[et al.]. Improving Model Performance on the Stratification of Breast Cancer Patients by Integrating Multiscale Genomic Features. BioMed Research International. 2020. Vol. 2020, no. 2020, pp.1-12.
https://search.emarefa.net/detail/BIM-1131670
نوع البيانات
مقالات
لغة النص
الإنجليزية
الملاحظات
Includes bibliographical references
رقم السجل
BIM-1131670
قاعدة معامل التأثير والاستشهادات المرجعية العربي "ارسيف Arcif"
أضخم قاعدة بيانات عربية للاستشهادات المرجعية للمجلات العلمية المحكمة الصادرة في العالم العربي
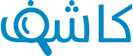
تقوم هذه الخدمة بالتحقق من التشابه أو الانتحال في الأبحاث والمقالات العلمية والأطروحات الجامعية والكتب والأبحاث باللغة العربية، وتحديد درجة التشابه أو أصالة الأعمال البحثية وحماية ملكيتها الفكرية. تعرف اكثر
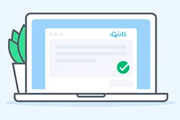