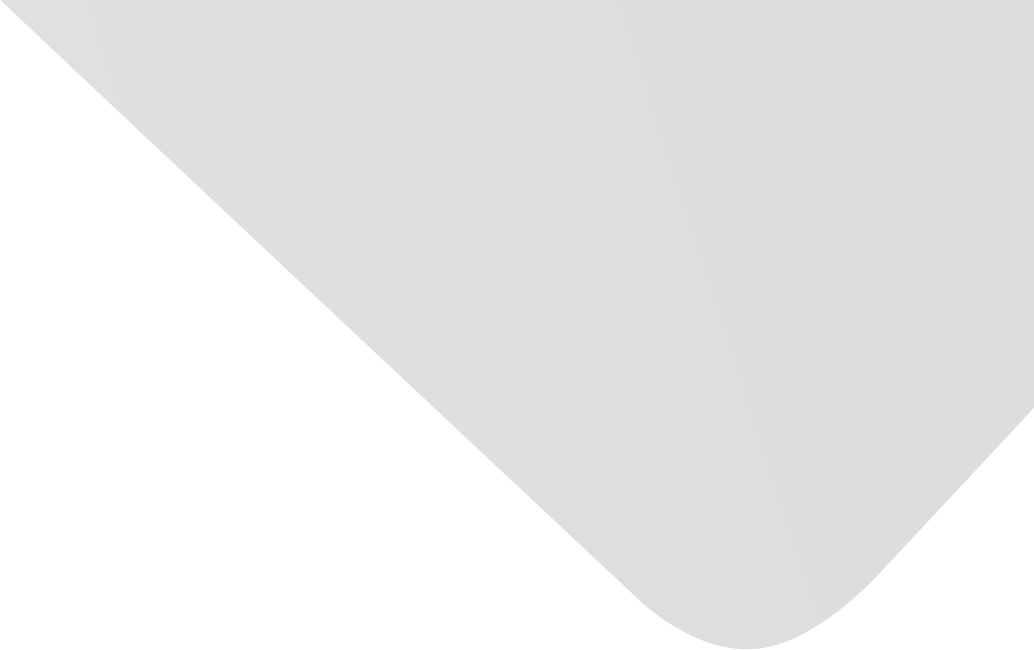
Pedestrian Re-Recognition Algorithm Based on Optimization Deep Learning-Sequence Memory Model
المؤلف
المصدر
العدد
المجلد 2019، العدد 2019 (31 ديسمبر/كانون الأول 2019)، ص ص. 1-16، 16ص.
الناشر
Hindawi Publishing Corporation
تاريخ النشر
2019-10-14
دولة النشر
مصر
عدد الصفحات
16
التخصصات الرئيسية
الملخص EN
Pedestrian re-recognition is an important research because it affects applications such as intelligent monitoring, content-based video retrieval, and human-computer interaction.
It can help relay tracking and criminal suspect detection in large-scale video surveillance systems.
Although the existing traditional pedestrian re-recognition methods have been widely applied to address practical problems, they have deficiencies such as low recognition accuracy, inefficient computation, and difficulty to adapt to specific applications.
In recent years, the pedestrian re-recognition algorithms based on deep learning have been widely used in the pedestrian re-recognition field because of their strong adaptive ability and high recognition accuracy.
The deep learning models provide a technical approach for pedestrian re-recognition tasks with their powerful learning ability.
However, the pedestrian re-recognition method based on deep learning also has the following problems: First, the existing deep learning pedestrian re-recognition methods lack memory and prediction mechanisms, and the deep learning methods offer only limited improvement to pedestrian re-recognition accuracy.
Second, they exhibit overfitting problems.
Finally, initializing the existing LSTM parameters is problematic.
In view of this, this paper introduces a revertive connection into the pedestrian re-recognition detector, making it more similar to the human cognitive process by converting a single image into an image sequence; then, the memory image sequence pattern reidentifies the pedestrian image.
This approach endows deep learning-based pedestrian re-recognition algorithms with the ability to memorize image sequence patterns and allows them to reidentify pedestrians in images.
At the same time, this paper proposes a selective dropout method for shallow learning.
Selective dropout uses the classifier obtained through shallow learning to modify the probability that a node weight in the hidden layer is set to 0, thereby eliminating the overfitting phenomenon of the deep learning model.
Therefore, this paper also proposes a greedy layer-by-layer pretraining algorithm for initializing LSTM and obtains better generalization performance.
Based on the above explanation, this paper proposes a pedestrian re-recognition algorithm based on an optimized LSTM deep learning-sequence memory learning model.
Experiments show that the pedestrian re-recognition method proposed in this paper not only has strong self-adaptive ability but also identifies the average accuracy.
The proposed method also demonstrates a significant improvement compared with other mainstream methods because it can better memorize and learn the continuous motion of pedestrians and effectively avoid overfitting and parameter initialization in the deep learning model.
This proposal provides a technical method and approach for adaptive pedestrian re-recognition algorithms.
نمط استشهاد جمعية علماء النفس الأمريكية (APA)
An, Feng-Ping. 2019. Pedestrian Re-Recognition Algorithm Based on Optimization Deep Learning-Sequence Memory Model. Complexity،Vol. 2019, no. 2019, pp.1-16.
https://search.emarefa.net/detail/BIM-1132008
نمط استشهاد الجمعية الأمريكية للغات الحديثة (MLA)
An, Feng-Ping. Pedestrian Re-Recognition Algorithm Based on Optimization Deep Learning-Sequence Memory Model. Complexity No. 2019 (2019), pp.1-16.
https://search.emarefa.net/detail/BIM-1132008
نمط استشهاد الجمعية الطبية الأمريكية (AMA)
An, Feng-Ping. Pedestrian Re-Recognition Algorithm Based on Optimization Deep Learning-Sequence Memory Model. Complexity. 2019. Vol. 2019, no. 2019, pp.1-16.
https://search.emarefa.net/detail/BIM-1132008
نوع البيانات
مقالات
لغة النص
الإنجليزية
الملاحظات
Includes bibliographical references
رقم السجل
BIM-1132008
قاعدة معامل التأثير والاستشهادات المرجعية العربي "ارسيف Arcif"
أضخم قاعدة بيانات عربية للاستشهادات المرجعية للمجلات العلمية المحكمة الصادرة في العالم العربي
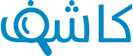
تقوم هذه الخدمة بالتحقق من التشابه أو الانتحال في الأبحاث والمقالات العلمية والأطروحات الجامعية والكتب والأبحاث باللغة العربية، وتحديد درجة التشابه أو أصالة الأعمال البحثية وحماية ملكيتها الفكرية. تعرف اكثر
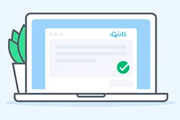