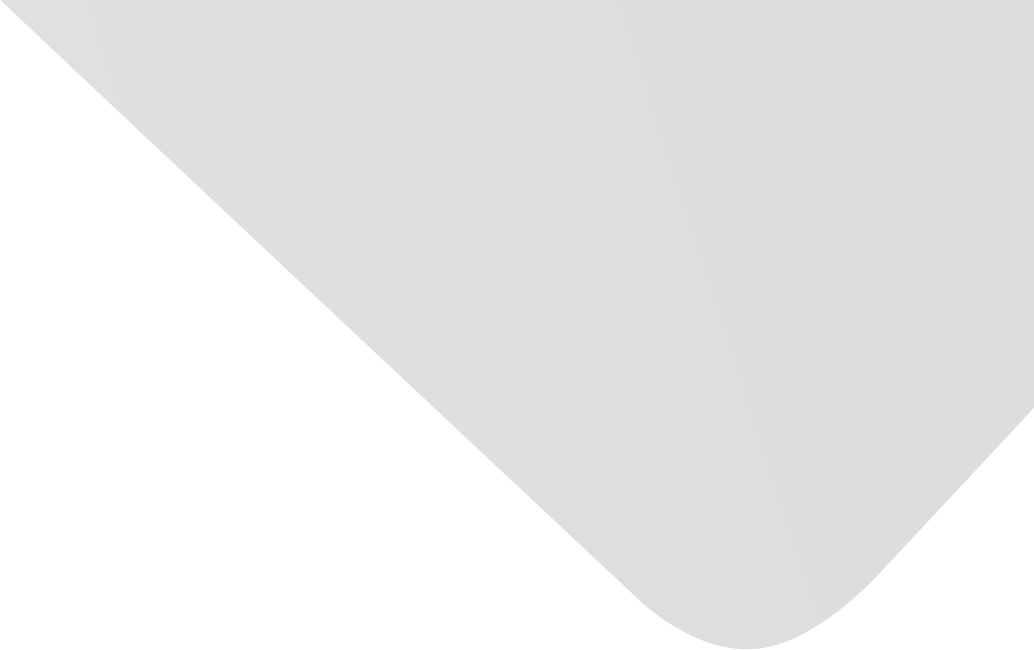
Predicting the Remaining Useful Life of an Aircraft Engine Using a Stacked Sparse Autoencoder with Multilayer Self-Learning
المؤلفون المشاركون
Ma, Jian
Su, Hua
Zhao, Wan-lin
Liu, Bin
المصدر
العدد
المجلد 2018، العدد 2018 (31 ديسمبر/كانون الأول 2018)، ص ص. 1-13، 13ص.
الناشر
Hindawi Publishing Corporation
تاريخ النشر
2018-07-30
دولة النشر
مصر
عدد الصفحات
13
التخصصات الرئيسية
الملخص EN
Because they are key components of aircraft, improving the safety, reliability and economy of engines is crucial.
To ensure flight safety and reduce the cost of maintenance during aircraft engine operation, a prognostics and health management system that focuses on fault diagnosis, health assessment, and life prediction is introduced to solve the problems.
Predicting the remaining useful life (RUL) is the most important information for making decisions about aircraft engine operation and maintenance, and it relies largely on the selection of performance degradation features.
The choice of such features is highly significant, but there are some weaknesses in the current algorithm for RUL prediction, notably, the inability to obtain tendencies from the data.
Especially with aircraft engines, extracting useful degradation features from multisensor data with complex correlations is a key technical problem that has hindered the implementation of degradation assessment.
To solve these problems, deep learning has been proposed in recent years to exploit multiple layers of nonlinear information processing for unsupervised self-learning of features.
This paper presents a deep learning approach to predict the RUL of an aircraft engine based on a stacked sparse autoencoder and logistic regression.
The stacked sparse autoencoder is used to automatically extract performance degradation features from multiple sensors on the aircraft engine and to fuse multiple features through multilayer self-learning.
Logistic regression is used to predict the remaining useful life.
However, the hyperparameters of the deep learning, which significantly impact the feature extraction and prediction performance, are determined based on expert experience in most cases.
The grid search method is introduced in this paper to optimize the hyperparameters of the proposed aircraft engine RUL prediction model.
An application of this method of predicting the RUL of an aircraft engine with a benchmark dataset is employed to demonstrate the effectiveness of the proposed approach.
نمط استشهاد جمعية علماء النفس الأمريكية (APA)
Ma, Jian& Su, Hua& Zhao, Wan-lin& Liu, Bin. 2018. Predicting the Remaining Useful Life of an Aircraft Engine Using a Stacked Sparse Autoencoder with Multilayer Self-Learning. Complexity،Vol. 2018, no. 2018, pp.1-13.
https://search.emarefa.net/detail/BIM-1133812
نمط استشهاد الجمعية الأمريكية للغات الحديثة (MLA)
Ma, Jian…[et al.]. Predicting the Remaining Useful Life of an Aircraft Engine Using a Stacked Sparse Autoencoder with Multilayer Self-Learning. Complexity No. 2018 (2018), pp.1-13.
https://search.emarefa.net/detail/BIM-1133812
نمط استشهاد الجمعية الطبية الأمريكية (AMA)
Ma, Jian& Su, Hua& Zhao, Wan-lin& Liu, Bin. Predicting the Remaining Useful Life of an Aircraft Engine Using a Stacked Sparse Autoencoder with Multilayer Self-Learning. Complexity. 2018. Vol. 2018, no. 2018, pp.1-13.
https://search.emarefa.net/detail/BIM-1133812
نوع البيانات
مقالات
لغة النص
الإنجليزية
الملاحظات
Includes bibliographical references
رقم السجل
BIM-1133812
قاعدة معامل التأثير والاستشهادات المرجعية العربي "ارسيف Arcif"
أضخم قاعدة بيانات عربية للاستشهادات المرجعية للمجلات العلمية المحكمة الصادرة في العالم العربي
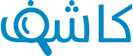
تقوم هذه الخدمة بالتحقق من التشابه أو الانتحال في الأبحاث والمقالات العلمية والأطروحات الجامعية والكتب والأبحاث باللغة العربية، وتحديد درجة التشابه أو أصالة الأعمال البحثية وحماية ملكيتها الفكرية. تعرف اكثر
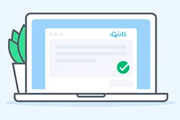