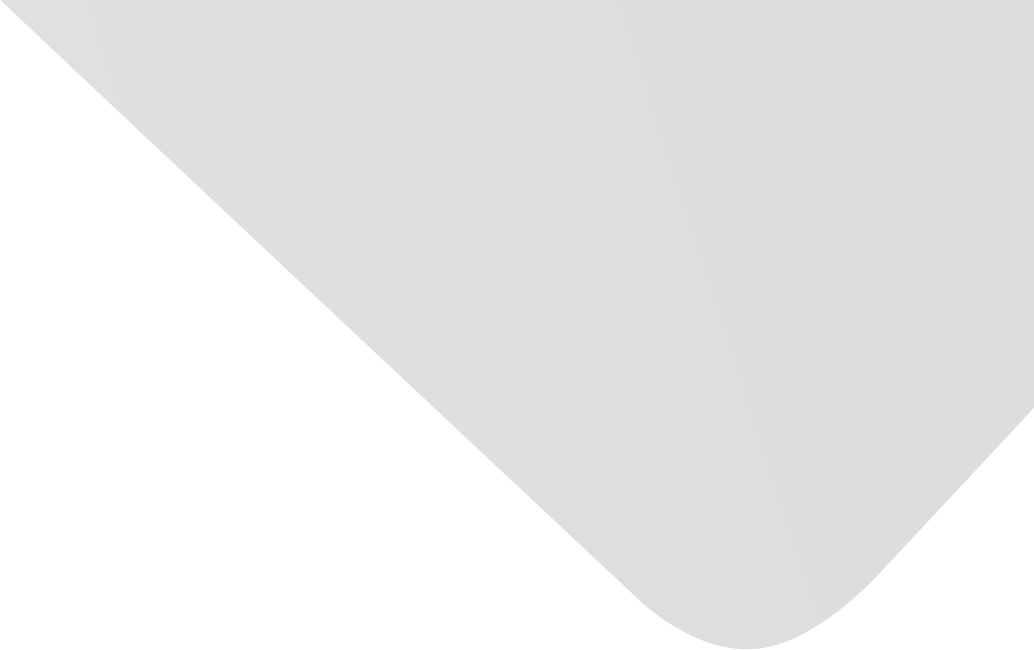
An Improved Multiobjective Quantum-Behaved Particle Swarm Optimization Based on Double Search Strategy and Circular Transposon Mechanism
المؤلفون المشاركون
Han, Fei
Sun, Yu-Wen-Tian
Ling, Qing-Hua
المصدر
العدد
المجلد 2018، العدد 2018 (31 ديسمبر/كانون الأول 2018)، ص ص. 1-22، 22ص.
الناشر
Hindawi Publishing Corporation
تاريخ النشر
2018-11-01
دولة النشر
مصر
عدد الصفحات
22
التخصصات الرئيسية
الملخص EN
Although multiobjective particle swarm optimization (MOPSO) has good performance in solving multiobjective optimization problems, how to obtain more accurate solutions as well as improve the distribution of the solutions set is still a challenge.
In this paper, to improve the convergence performance of MOPSO, an improved multiobjective quantum-behaved particle swarm optimization based on double search strategy and circular transposon mechanism (MOQPSO-DSCT) is proposed.
On one hand, to solve the problem of the dramatic diversity reduction of the solutions set in later iterations due to the single search pattern used in quantum-behaved particle swarm optimization (QPSO), the double search strategy is proposed in MOQPSO-DSCT.
The particles mainly learn from their personal best position in earlier iterations and then the particles mainly learn from the global best position in later iterations to balance the exploration and exploitation ability of the swarm.
Moreover, to alleviate the problem of the swarm converging to local minima during the local search, an improved attractor construction mechanism based on opposition-based learning is introduced to further search a better position locally as a new attractor for each particle.
On the other hand, to improve the accuracy of the solutions set, the circular transposon mechanism is introduced into the external archive to improve the communication ability of the particles, which could guide the population toward the true Pareto front (PF).
The proposed algorithm could generate a set of more accurate and well-distributed solutions compared to the traditional MOPSO.
Finally, the experiments on a set of benchmark test functions have verified that the proposed algorithm has better convergence performance than some state-of-the-art multiobjective optimization algorithms.
نمط استشهاد جمعية علماء النفس الأمريكية (APA)
Han, Fei& Sun, Yu-Wen-Tian& Ling, Qing-Hua. 2018. An Improved Multiobjective Quantum-Behaved Particle Swarm Optimization Based on Double Search Strategy and Circular Transposon Mechanism. Complexity،Vol. 2018, no. 2018, pp.1-22.
https://search.emarefa.net/detail/BIM-1136330
نمط استشهاد الجمعية الأمريكية للغات الحديثة (MLA)
Han, Fei…[et al.]. An Improved Multiobjective Quantum-Behaved Particle Swarm Optimization Based on Double Search Strategy and Circular Transposon Mechanism. Complexity No. 2018 (2018), pp.1-22.
https://search.emarefa.net/detail/BIM-1136330
نمط استشهاد الجمعية الطبية الأمريكية (AMA)
Han, Fei& Sun, Yu-Wen-Tian& Ling, Qing-Hua. An Improved Multiobjective Quantum-Behaved Particle Swarm Optimization Based on Double Search Strategy and Circular Transposon Mechanism. Complexity. 2018. Vol. 2018, no. 2018, pp.1-22.
https://search.emarefa.net/detail/BIM-1136330
نوع البيانات
مقالات
لغة النص
الإنجليزية
الملاحظات
Includes bibliographical references
رقم السجل
BIM-1136330
قاعدة معامل التأثير والاستشهادات المرجعية العربي "ارسيف Arcif"
أضخم قاعدة بيانات عربية للاستشهادات المرجعية للمجلات العلمية المحكمة الصادرة في العالم العربي
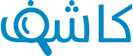
تقوم هذه الخدمة بالتحقق من التشابه أو الانتحال في الأبحاث والمقالات العلمية والأطروحات الجامعية والكتب والأبحاث باللغة العربية، وتحديد درجة التشابه أو أصالة الأعمال البحثية وحماية ملكيتها الفكرية. تعرف اكثر
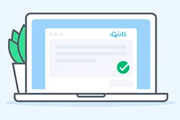