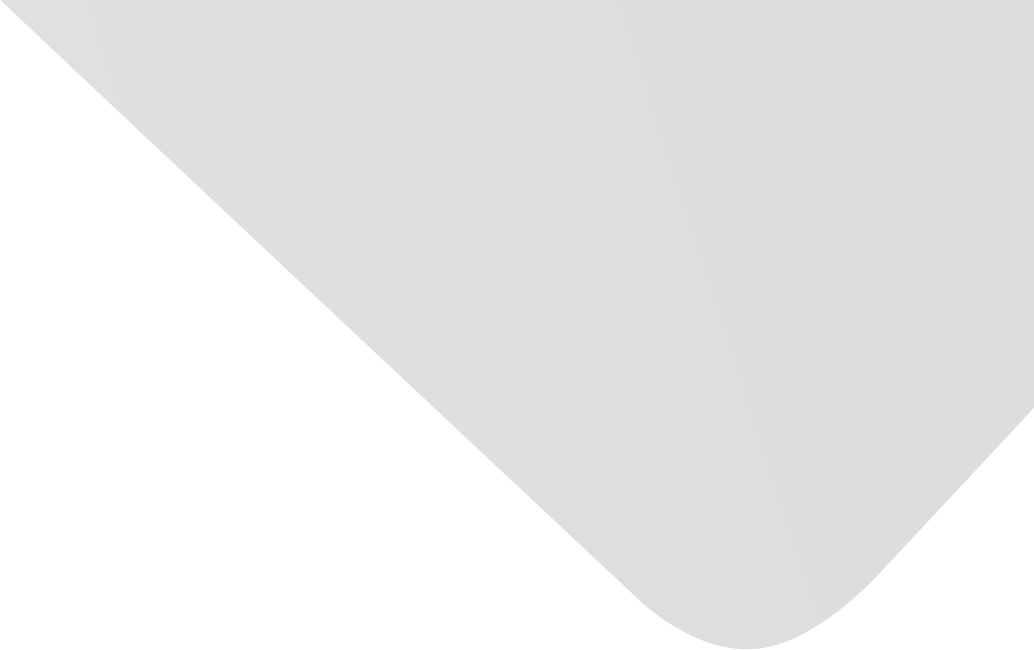
Deep Neural Network with Joint Distribution Matching for Cross-Subject Motor Imagery Brain-Computer Interfaces
المؤلفون المشاركون
Zhao, Xianghong
Zhao, Jieyu
Liu, Cong
Cai, W.
المصدر
العدد
المجلد 2020، العدد 2020 (31 ديسمبر/كانون الأول 2020)، ص ص. 1-15، 15ص.
الناشر
Hindawi Publishing Corporation
تاريخ النشر
2020-02-24
دولة النشر
مصر
عدد الصفحات
15
التخصصات الرئيسية
الملخص EN
Motor imagery brain-computer interfaces (BCIs) have demonstrated great potential and attract world-spread attentions.
Due to the nonstationary character of the motor imagery signals, costly and boring calibration sessions must be proceeded before use.
This prevents them from going into our realistic life.
In this paper, the source subject’s data are explored to perform calibration for target subjects.
Model trained on source subjects is transferred to work for target subjects, in which the critical problem to handle is the distribution shift.
It is found that the performance of classification would be bad when only the marginal distributions of source and target are made closer, since the discriminative directions of the source and target domains may still be much different.
In order to solve the problem, our idea comes that joint distribution adaptation is indispensable.
It makes the classifier trained in the source domain perform well in the target domain.
Specifically, a measure for joint distribution discrepancy (JDD) between the source and target is proposed.
Experiments demonstrate that it can align source and target data according to the class they belong to.
It has a direct relationship with classification accuracy and works well for transferring.
Secondly, a deep neural network with joint distribution matching for zero-training motor imagery BCI is proposed.
It explores both marginal and joint distribution adaptation to alleviate distribution discrepancy across subjects and obtain effective and generalized features in an aligned common space.
Visualizations of intermediate layers illustrate how and why the network works well.
Experiments on the two datasets prove the effectiveness and strength compared to outstanding counterparts.
نمط استشهاد جمعية علماء النفس الأمريكية (APA)
Zhao, Xianghong& Zhao, Jieyu& Liu, Cong& Cai, W.. 2020. Deep Neural Network with Joint Distribution Matching for Cross-Subject Motor Imagery Brain-Computer Interfaces. BioMed Research International،Vol. 2020, no. 2020, pp.1-15.
https://search.emarefa.net/detail/BIM-1136728
نمط استشهاد الجمعية الأمريكية للغات الحديثة (MLA)
Zhao, Xianghong…[et al.]. Deep Neural Network with Joint Distribution Matching for Cross-Subject Motor Imagery Brain-Computer Interfaces. BioMed Research International No. 2020 (2020), pp.1-15.
https://search.emarefa.net/detail/BIM-1136728
نمط استشهاد الجمعية الطبية الأمريكية (AMA)
Zhao, Xianghong& Zhao, Jieyu& Liu, Cong& Cai, W.. Deep Neural Network with Joint Distribution Matching for Cross-Subject Motor Imagery Brain-Computer Interfaces. BioMed Research International. 2020. Vol. 2020, no. 2020, pp.1-15.
https://search.emarefa.net/detail/BIM-1136728
نوع البيانات
مقالات
لغة النص
الإنجليزية
الملاحظات
Includes bibliographical references
رقم السجل
BIM-1136728
قاعدة معامل التأثير والاستشهادات المرجعية العربي "ارسيف Arcif"
أضخم قاعدة بيانات عربية للاستشهادات المرجعية للمجلات العلمية المحكمة الصادرة في العالم العربي
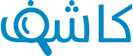
تقوم هذه الخدمة بالتحقق من التشابه أو الانتحال في الأبحاث والمقالات العلمية والأطروحات الجامعية والكتب والأبحاث باللغة العربية، وتحديد درجة التشابه أو أصالة الأعمال البحثية وحماية ملكيتها الفكرية. تعرف اكثر
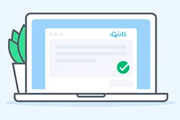