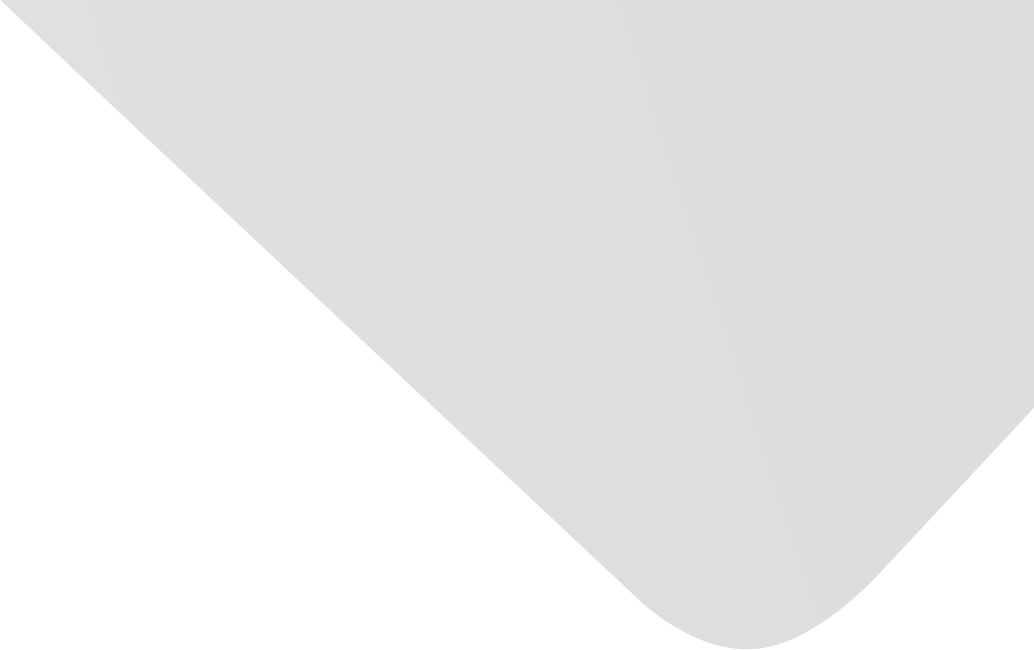
RNA-Seq-Based Breast Cancer Subtypes Classification Using Machine Learning Approaches
المؤلفون المشاركون
Zhang, Zhe
Yu, Zhezhou
Wang, Zhuo
Yu, Xiangchun
المصدر
Computational Intelligence and Neuroscience
العدد
المجلد 2020، العدد 2020 (31 ديسمبر/كانون الأول 2020)، ص ص. 1-13، 13ص.
الناشر
Hindawi Publishing Corporation
تاريخ النشر
2020-10-29
دولة النشر
مصر
عدد الصفحات
13
التخصصات الرئيسية
الملخص EN
Background.
Breast invasive carcinoma (BRCA) is not a single disease as each subtype has a distinct morphology structure.
Although several computational methods have been proposed to conduct breast cancer subtype identification, the specific interaction mechanisms of genes involved in the subtypes are still incomplete.
To identify and explore the corresponding interaction mechanisms of genes for each subtype of breast cancer can impose an important impact on the personalized treatment for different patients.
Methods.
We integrate the biological importance of genes from the gene regulatory networks to the differential expression analysis and then obtain the weighted differentially expressed genes (weighted DEGs).
A gene with a high weight means it regulates more target genes and thus holds more biological importance.
Besides, we constructed gene coexpression networks for control and experiment groups, and the significantly differentially interacting structures encouraged us to design the corresponding Gene Ontology (GO) enrichment based on gene coexpression networks (GOEGCN).
The GOEGCN considers the two-side distinction analysis between gene coexpression networks for control and experiment groups.
The method allows us to study how the modulated coexpressed gene couples impact biological functions at a GO level.
Results.
We modeled the binary classification with weighted DEGs for each subtype.
The binary classifier could make a good prediction for an unseen sample, and the experimental results validated the effectiveness of our proposed approaches.
The novel enriched GO terms based on GOEGCN for control and experiment groups of each subtype explain the specific biological function changes according to the two-side distinction of coexpression network structures to some extent.
Conclusion.
The weighted DEGs contain biological importance derived from the gene regulatory network.
Based on the weighted DEGs, five binary classifiers were learned and showed good performance concerning the “Sensitivity,” “Specificity,” “Accuracy,” “F1,” and “AUC” metrics.
The GOEGCN with weighted DEGs for control and experiment groups presented a novel GO enrichment analysis results and the novel enriched GO terms would further unveil the changes of specific biological functions among all the BRCA subtypes to some extent.
The R code in this research is available at https://github.com/yxchspring/GOEGCN_BRCA_Subtypes.
نمط استشهاد جمعية علماء النفس الأمريكية (APA)
Yu, Zhezhou& Wang, Zhuo& Yu, Xiangchun& Zhang, Zhe. 2020. RNA-Seq-Based Breast Cancer Subtypes Classification Using Machine Learning Approaches. Computational Intelligence and Neuroscience،Vol. 2020, no. 2020, pp.1-13.
https://search.emarefa.net/detail/BIM-1138761
نمط استشهاد الجمعية الأمريكية للغات الحديثة (MLA)
Yu, Zhezhou…[et al.]. RNA-Seq-Based Breast Cancer Subtypes Classification Using Machine Learning Approaches. Computational Intelligence and Neuroscience No. 2020 (2020), pp.1-13.
https://search.emarefa.net/detail/BIM-1138761
نمط استشهاد الجمعية الطبية الأمريكية (AMA)
Yu, Zhezhou& Wang, Zhuo& Yu, Xiangchun& Zhang, Zhe. RNA-Seq-Based Breast Cancer Subtypes Classification Using Machine Learning Approaches. Computational Intelligence and Neuroscience. 2020. Vol. 2020, no. 2020, pp.1-13.
https://search.emarefa.net/detail/BIM-1138761
نوع البيانات
مقالات
لغة النص
الإنجليزية
الملاحظات
Includes bibliographical references
رقم السجل
BIM-1138761
قاعدة معامل التأثير والاستشهادات المرجعية العربي "ارسيف Arcif"
أضخم قاعدة بيانات عربية للاستشهادات المرجعية للمجلات العلمية المحكمة الصادرة في العالم العربي
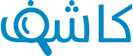
تقوم هذه الخدمة بالتحقق من التشابه أو الانتحال في الأبحاث والمقالات العلمية والأطروحات الجامعية والكتب والأبحاث باللغة العربية، وتحديد درجة التشابه أو أصالة الأعمال البحثية وحماية ملكيتها الفكرية. تعرف اكثر
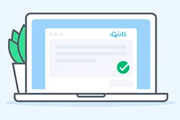