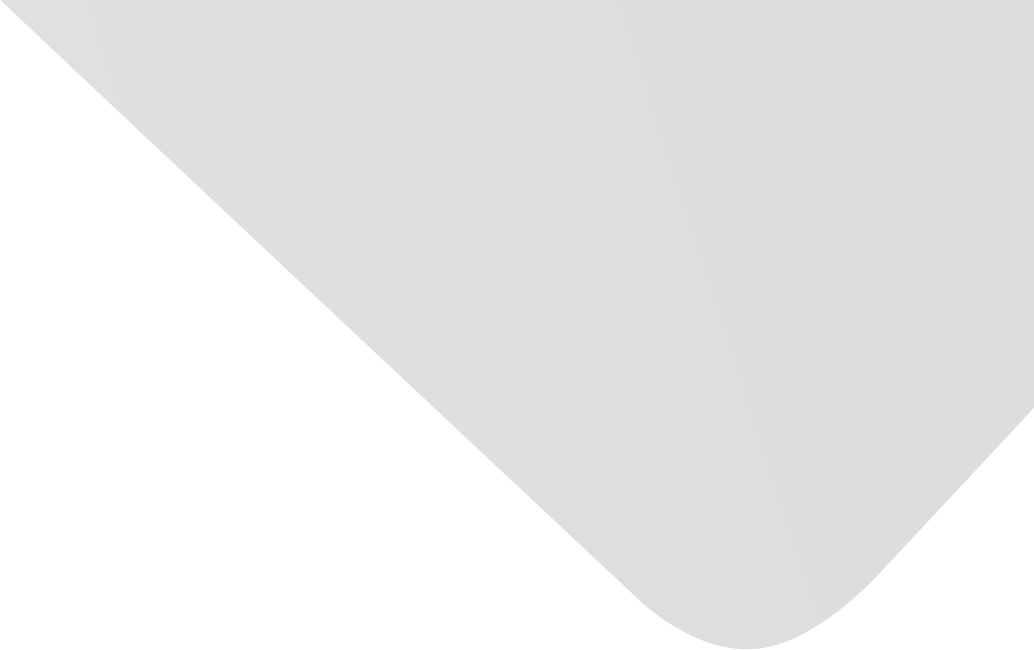
A Cross-Reference Line Method Based Multiobjective Evolutionary Algorithm to Enhance Population Diversity
المؤلفون المشاركون
Chen, Zhi-Yuan
Fu, Ting
Feng, Ya-Nan
Wang, Zhao-Hui
Fan, Jia-Rong
المصدر
Computational Intelligence and Neuroscience
العدد
المجلد 2020، العدد 2020 (31 ديسمبر/كانون الأول 2020)، ص ص. 1-23، 23ص.
الناشر
Hindawi Publishing Corporation
تاريخ النشر
2020-07-20
دولة النشر
مصر
عدد الصفحات
23
التخصصات الرئيسية
الملخص EN
Multiobjective evolutionary algorithms (MOEAs) with higher population diversity have been extensively presented in literature studies and shown great potential in the approximate Pareto front (PF).
Especially, in the recent development of MOEAs, the reference line method is increasingly favored due to its diversity enhancement nature and auxiliary selection mechanism based on the uniformly distributed reference line.
However, the existing reference line method ignores the nadir point and consequently causes the Pareto incompatibility problem, which makes the algorithm convergence worse.
To address this issue, a multiobjective evolutionary algorithm based on the adaptive cross-reference line method, called MOEA-CRL, is proposed under the framework of the indicator-based MOEAs.
Based on the dominant penalty distance (DPD) indicator, the cross-reference line method can not only solve the Pareto incompatibility problem but also enhance the population diversity on the convex PF and improve the performances of MOEA-CRL for irregular PF.
In addition, the MOEA-CRL adjusts the distribution of the cross-reference lines directly defined by the DPD indicator according to the contributing solutions.
Therefore, the adaptation of cross-reference lines will not be affected by the population size and the uniform distribution of cross-reference lines can be maintained.
The MOEA-CRL is examined and compared with other MOEAs on several benchmark problems.
The experimental results show that the MOEA-CRL is superior to several advanced MOEAs, especially on the convex PF.
The MOEA-CRL exhibits the flexibility in population size setting and the great versatility in various multiobjective optimization problems (MOPs) and many-objective optimization problems (MaOPs).
نمط استشهاد جمعية علماء النفس الأمريكية (APA)
Feng, Ya-Nan& Wang, Zhao-Hui& Fan, Jia-Rong& Fu, Ting& Chen, Zhi-Yuan. 2020. A Cross-Reference Line Method Based Multiobjective Evolutionary Algorithm to Enhance Population Diversity. Computational Intelligence and Neuroscience،Vol. 2020, no. 2020, pp.1-23.
https://search.emarefa.net/detail/BIM-1138804
نمط استشهاد الجمعية الأمريكية للغات الحديثة (MLA)
Feng, Ya-Nan…[et al.]. A Cross-Reference Line Method Based Multiobjective Evolutionary Algorithm to Enhance Population Diversity. Computational Intelligence and Neuroscience No. 2020 (2020), pp.1-23.
https://search.emarefa.net/detail/BIM-1138804
نمط استشهاد الجمعية الطبية الأمريكية (AMA)
Feng, Ya-Nan& Wang, Zhao-Hui& Fan, Jia-Rong& Fu, Ting& Chen, Zhi-Yuan. A Cross-Reference Line Method Based Multiobjective Evolutionary Algorithm to Enhance Population Diversity. Computational Intelligence and Neuroscience. 2020. Vol. 2020, no. 2020, pp.1-23.
https://search.emarefa.net/detail/BIM-1138804
نوع البيانات
مقالات
لغة النص
الإنجليزية
الملاحظات
Includes bibliographical references
رقم السجل
BIM-1138804
قاعدة معامل التأثير والاستشهادات المرجعية العربي "ارسيف Arcif"
أضخم قاعدة بيانات عربية للاستشهادات المرجعية للمجلات العلمية المحكمة الصادرة في العالم العربي
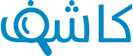
تقوم هذه الخدمة بالتحقق من التشابه أو الانتحال في الأبحاث والمقالات العلمية والأطروحات الجامعية والكتب والأبحاث باللغة العربية، وتحديد درجة التشابه أو أصالة الأعمال البحثية وحماية ملكيتها الفكرية. تعرف اكثر
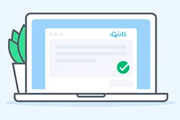