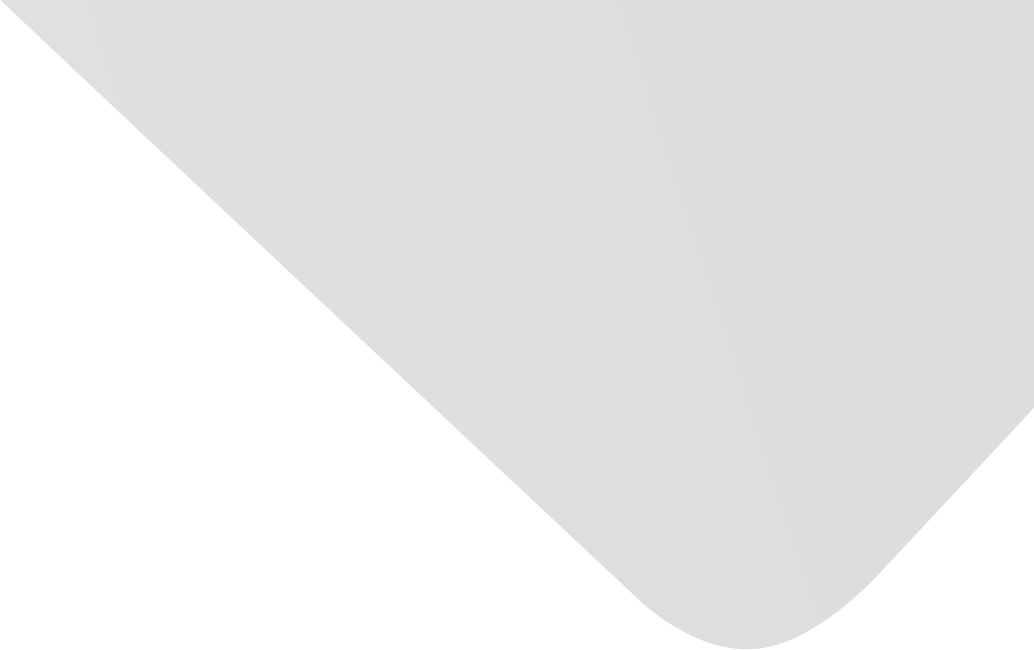
Graph Neural Network and Context-Aware Based User Behavior Prediction and Recommendation System Research
المؤلفون المشاركون
المصدر
Computational Intelligence and Neuroscience
العدد
المجلد 2020، العدد 2020 (31 ديسمبر/كانون الأول 2020)، ص ص. 1-14، 14ص.
الناشر
Hindawi Publishing Corporation
تاريخ النشر
2020-11-30
دولة النشر
مصر
عدد الصفحات
14
التخصصات الرئيسية
الملخص EN
Due to the influence of context information on user behavior, context-aware recommendation system (CARS) has attracted extensive attention in recent years.
The most advanced context-aware recommendation system maps the original multi-field features into a shared hidden space and then simply connects it to a deep neural network (DNN) or other specially designed networks.
However, for different areas, the ability of modeling complex interactions in a sufficiently flexible and explicit way is limited by the simple unstructured combination of feature fields.
Therefore, it is hard to get the accurate results of the user behavior prediction.
In this paper, a graph structure is used to establish the interaction between context and users/items.
Through modeling user behavior, we can explore user preferences in different context environments, so as to make personalized recommendations for users.
In particular, we construct a context-user and context-item interactions graph separately.
In the interactions graph, each node is composed of a user feature field, an item feature field, and a feature field of different contexts.
Different feature fields can interact through edges.
Therefore, the task of modeling feature interaction can be transformed into modeling the node interaction on the corresponding graph.
To this end, an innovative model called context-aware graph neural network (CA-GNN) model is designed.
Furthermore, in order to obtain more accurate and efficient recommendation results, first, we innovatively use the attention mechanism to improve the interpretability of CA-GNN; second, we innovatively use the degree of physical fatigue features which has never been used in traditional CARS as critical contextual feature information into our CA-GNN.
We simulated the Food and Yelp datasets.
The experimental results show that CA-GNN is better than other methods in terms of root mean square error (RMSE) and mean absolute error (MAE).
نمط استشهاد جمعية علماء النفس الأمريكية (APA)
Gao, Qian& Ma, Pengcheng. 2020. Graph Neural Network and Context-Aware Based User Behavior Prediction and Recommendation System Research. Computational Intelligence and Neuroscience،Vol. 2020, no. 2020, pp.1-14.
https://search.emarefa.net/detail/BIM-1138847
نمط استشهاد الجمعية الأمريكية للغات الحديثة (MLA)
Gao, Qian& Ma, Pengcheng. Graph Neural Network and Context-Aware Based User Behavior Prediction and Recommendation System Research. Computational Intelligence and Neuroscience No. 2020 (2020), pp.1-14.
https://search.emarefa.net/detail/BIM-1138847
نمط استشهاد الجمعية الطبية الأمريكية (AMA)
Gao, Qian& Ma, Pengcheng. Graph Neural Network and Context-Aware Based User Behavior Prediction and Recommendation System Research. Computational Intelligence and Neuroscience. 2020. Vol. 2020, no. 2020, pp.1-14.
https://search.emarefa.net/detail/BIM-1138847
نوع البيانات
مقالات
لغة النص
الإنجليزية
الملاحظات
Includes bibliographical references
رقم السجل
BIM-1138847
قاعدة معامل التأثير والاستشهادات المرجعية العربي "ارسيف Arcif"
أضخم قاعدة بيانات عربية للاستشهادات المرجعية للمجلات العلمية المحكمة الصادرة في العالم العربي
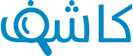
تقوم هذه الخدمة بالتحقق من التشابه أو الانتحال في الأبحاث والمقالات العلمية والأطروحات الجامعية والكتب والأبحاث باللغة العربية، وتحديد درجة التشابه أو أصالة الأعمال البحثية وحماية ملكيتها الفكرية. تعرف اكثر
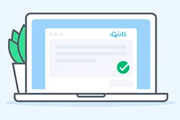