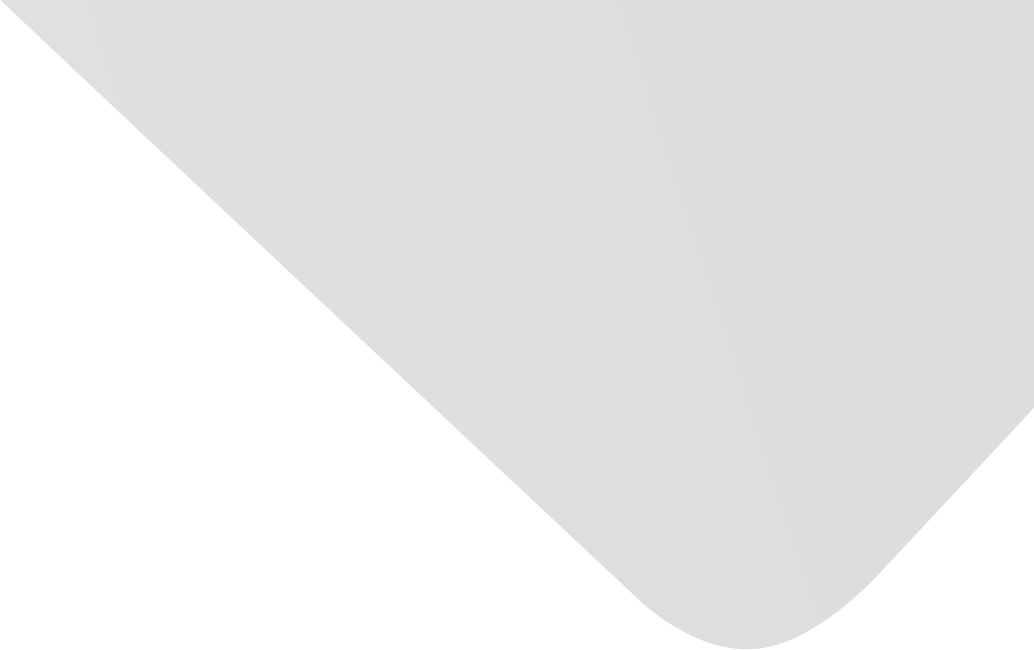
A Semisupervised Learning Scheme with Self-Paced Learning for Classifying Breast Cancer Histopathological Images
المؤلفون المشاركون
Asare, Sarpong Kwadwo
You, Fei
Nartey, Obed Tettey
المصدر
Computational Intelligence and Neuroscience
العدد
المجلد 2020، العدد 2020 (31 ديسمبر/كانون الأول 2020)، ص ص. 1-16، 16ص.
الناشر
Hindawi Publishing Corporation
تاريخ النشر
2020-12-08
دولة النشر
مصر
عدد الصفحات
16
التخصصات الرئيسية
الملخص EN
The unavailability of large amounts of well-labeled data poses a significant challenge in many medical imaging tasks.
Even in the likelihood of having access to sufficient data, the process of accurately labeling the data is an arduous and time-consuming one, requiring expertise skills.
Again, the issue of unbalanced data further compounds the abovementioned problems and presents a considerable challenge for many machine learning algorithms.
In lieu of this, the ability to develop algorithms that can exploit large amounts of unlabeled data together with a small amount of labeled data, while demonstrating robustness to data imbalance, can offer promising prospects in building highly efficient classifiers.
This work proposes a semisupervised learning method that integrates self-training and self-paced learning to generate and select pseudolabeled samples for classifying breast cancer histopathological images.
A novel pseudolabel generation and selection algorithm is introduced in the learning scheme to generate and select highly confident pseudolabeled samples from both well-represented classes to less-represented classes.
Such a learning approach improves the performance by jointly learning a model and optimizing the generation of pseudolabels on unlabeled-target data to augment the training data and retraining the model with the generated labels.
A class balancing framework that normalizes the class-wise confidence scores is also proposed to prevent the model from ignoring samples from less represented classes (hard-to-learn samples), hence effectively handling the issue of data imbalance.
Extensive experimental evaluation of the proposed method on the BreakHis dataset demonstrates the effectiveness of the proposed method.
نمط استشهاد جمعية علماء النفس الأمريكية (APA)
Asare, Sarpong Kwadwo& You, Fei& Nartey, Obed Tettey. 2020. A Semisupervised Learning Scheme with Self-Paced Learning for Classifying Breast Cancer Histopathological Images. Computational Intelligence and Neuroscience،Vol. 2020, no. 2020, pp.1-16.
https://search.emarefa.net/detail/BIM-1138865
نمط استشهاد الجمعية الأمريكية للغات الحديثة (MLA)
Asare, Sarpong Kwadwo…[et al.]. A Semisupervised Learning Scheme with Self-Paced Learning for Classifying Breast Cancer Histopathological Images. Computational Intelligence and Neuroscience No. 2020 (2020), pp.1-16.
https://search.emarefa.net/detail/BIM-1138865
نمط استشهاد الجمعية الطبية الأمريكية (AMA)
Asare, Sarpong Kwadwo& You, Fei& Nartey, Obed Tettey. A Semisupervised Learning Scheme with Self-Paced Learning for Classifying Breast Cancer Histopathological Images. Computational Intelligence and Neuroscience. 2020. Vol. 2020, no. 2020, pp.1-16.
https://search.emarefa.net/detail/BIM-1138865
نوع البيانات
مقالات
لغة النص
الإنجليزية
الملاحظات
Includes bibliographical references
رقم السجل
BIM-1138865
قاعدة معامل التأثير والاستشهادات المرجعية العربي "ارسيف Arcif"
أضخم قاعدة بيانات عربية للاستشهادات المرجعية للمجلات العلمية المحكمة الصادرة في العالم العربي

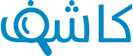
تقوم هذه الخدمة بالتحقق من التشابه أو الانتحال في الأبحاث والمقالات العلمية والأطروحات الجامعية والكتب والأبحاث باللغة العربية، وتحديد درجة التشابه أو أصالة الأعمال البحثية وحماية ملكيتها الفكرية. تعرف اكثر
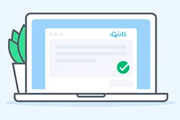