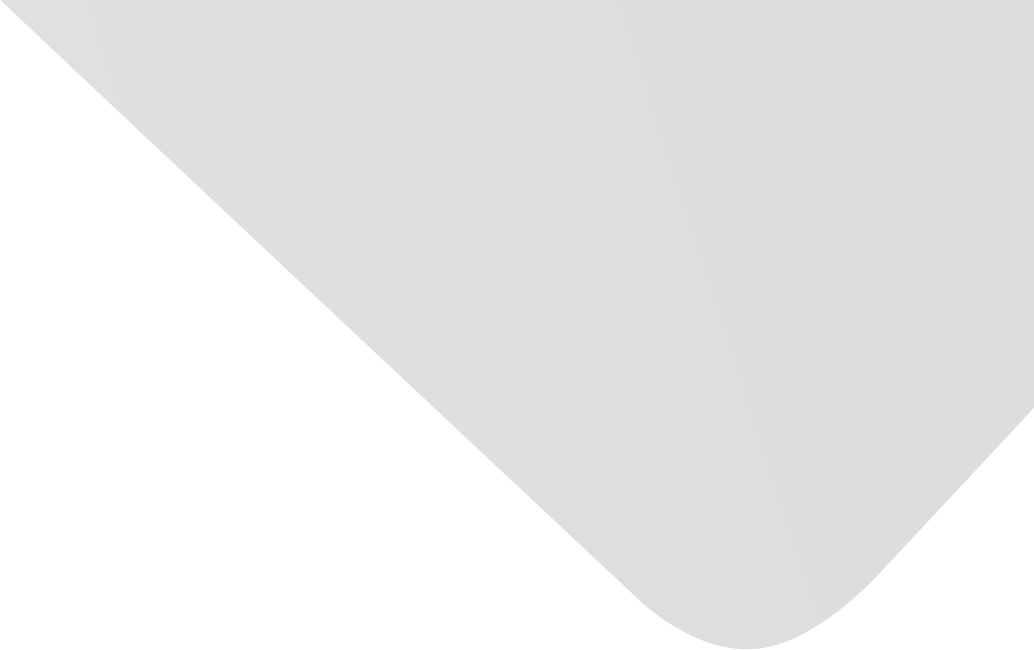
Interactive Dual Attention Network for Text Sentiment Classification
المؤلفون المشاركون
Zhu, Yinglin
Zheng, Wenbin
Tang, Hong
المصدر
Computational Intelligence and Neuroscience
العدد
المجلد 2020، العدد 2020 (31 ديسمبر/كانون الأول 2020)، ص ص. 1-11، 11ص.
الناشر
Hindawi Publishing Corporation
تاريخ النشر
2020-11-04
دولة النشر
مصر
عدد الصفحات
11
التخصصات الرئيسية
الملخص EN
Text sentiment classification is an essential research field of natural language processing.
Recently, numerous deep learning-based methods for sentiment classification have been proposed and achieved better performances compared with conventional machine learning methods.
However, most of the proposed methods ignore the interactive relationship between contextual semantics and sentimental tendency while modeling their text representation.
In this paper, we propose a novel Interactive Dual Attention Network (IDAN) model that aims to interactively learn the representation between contextual semantics and sentimental tendency information.
Firstly, we design an algorithm that utilizes linguistic resources to obtain sentimental tendency information from text and then extract word embeddings from the BERT (Bidirectional Encoder Representations from Transformers) pretraining model as the embedding layer of IDAN.
Next, we use two Bidirectional LSTM (BiLSTM) networks to learn the long-range dependencies of contextual semantics and sentimental tendency information, respectively.
Finally, two types of attention mechanisms are implemented in IDAN.
One is multihead attention, which is the next layer of BiLSTM and is used to learn the interactive relationship between contextual semantics and sentimental tendency information.
The other is global attention that aims to make the model focus on the important parts of the sequence and generate the final representation for classification.
These two attention mechanisms enable IDAN to interactively learn the relationship between semantics and sentimental tendency information and improve the classification performance.
A large number of experiments on four benchmark datasets show that our IDAN model is superior to competitive methods.
Moreover, both the result analysis and the attention weight visualization further demonstrate the effectiveness of our proposed method.
نمط استشهاد جمعية علماء النفس الأمريكية (APA)
Zhu, Yinglin& Zheng, Wenbin& Tang, Hong. 2020. Interactive Dual Attention Network for Text Sentiment Classification. Computational Intelligence and Neuroscience،Vol. 2020, no. 2020, pp.1-11.
https://search.emarefa.net/detail/BIM-1138912
نمط استشهاد الجمعية الأمريكية للغات الحديثة (MLA)
Zhu, Yinglin…[et al.]. Interactive Dual Attention Network for Text Sentiment Classification. Computational Intelligence and Neuroscience No. 2020 (2020), pp.1-11.
https://search.emarefa.net/detail/BIM-1138912
نمط استشهاد الجمعية الطبية الأمريكية (AMA)
Zhu, Yinglin& Zheng, Wenbin& Tang, Hong. Interactive Dual Attention Network for Text Sentiment Classification. Computational Intelligence and Neuroscience. 2020. Vol. 2020, no. 2020, pp.1-11.
https://search.emarefa.net/detail/BIM-1138912
نوع البيانات
مقالات
لغة النص
الإنجليزية
الملاحظات
Includes bibliographical references
رقم السجل
BIM-1138912
قاعدة معامل التأثير والاستشهادات المرجعية العربي "ارسيف Arcif"
أضخم قاعدة بيانات عربية للاستشهادات المرجعية للمجلات العلمية المحكمة الصادرة في العالم العربي

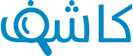
تقوم هذه الخدمة بالتحقق من التشابه أو الانتحال في الأبحاث والمقالات العلمية والأطروحات الجامعية والكتب والأبحاث باللغة العربية، وتحديد درجة التشابه أو أصالة الأعمال البحثية وحماية ملكيتها الفكرية. تعرف اكثر
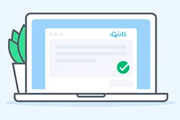