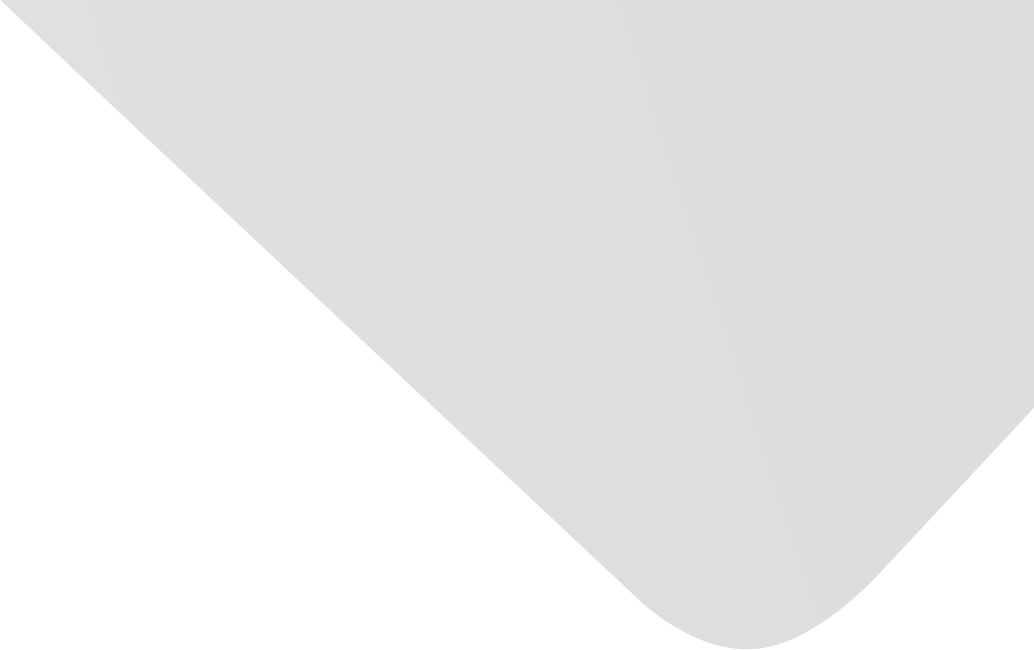
Adoption of Machine Learning in Intelligent Terrain Classification of Hyperspectral Remote Sensing Images
المؤلفون المشاركون
Gao, Tong
Zhang, Liguo
Li, Yanyi
Wang, Jian
Sun, Qiwen
Tang, Mingxiu
المصدر
Computational Intelligence and Neuroscience
العدد
المجلد 2020، العدد 2020 (31 ديسمبر/كانون الأول 2020)، ص ص. 1-13، 13ص.
الناشر
Hindawi Publishing Corporation
تاريخ النشر
2020-09-01
دولة النشر
مصر
عدد الصفحات
13
التخصصات الرئيسية
الملخص EN
To overcome the difficulty of automating and intelligently classifying the ground features in remote-sensing hyperspectral images, machine learning methods are gradually introduced into the process of remote-sensing imaging.
First, the PaviaU, Botswana, and Cuprite hyperspectral datasets are selected as research subjects in this study, and the objective is to process remote-sensing hyperspectral images via machine learning to realize the automatic and intelligent classification of features.
Then, the basic principles of the support vector machine (SVM) and extreme learning machine (ELM) classification algorithms are introduced, and they are applied to the datasets.
Next, by adjusting the parameter estimates using a restricted Boltzmann machine (RBM), a new terrain classification model of hyperspectral images that is based on a deep belief network (DBN) is constructed.
Next, the SVM, ELM, and DBN classification algorithms for hyperspectral image terrain classification are analysed and compared in terms of accuracy and consistency.
The results demonstrate that the average detection accuracies of ELM on the three datasets are 89.54%, 96.14%, and 96.28%, and the Kappa coefficient values are 0.832, 0.963, and 0.924; the average detection accuracies of SVM are 88.90%, 92.11%, and 91.68%, and the Kappa coefficient values are 0.768, 0.913, and 0.944; the average detection accuracies of the DBN classification model are 92.36%, 97.31%, and 98.84%, and the Kappa coefficient values are 0.883, 0.944, and 0.972.
The results also demonstrate that the classification accuracy of the DBN algorithm exceeds those of the previous two methods because it fully utilizes the spatial and spectral information of hyperspectral remote-sensing images.
In summary, the DBN algorithm that is proposed in this study has high application value in object classification for remote-sensing hyperspectral images.
نمط استشهاد جمعية علماء النفس الأمريكية (APA)
Li, Yanyi& Wang, Jian& Gao, Tong& Sun, Qiwen& Zhang, Liguo& Tang, Mingxiu. 2020. Adoption of Machine Learning in Intelligent Terrain Classification of Hyperspectral Remote Sensing Images. Computational Intelligence and Neuroscience،Vol. 2020, no. 2020, pp.1-13.
https://search.emarefa.net/detail/BIM-1138948
نمط استشهاد الجمعية الأمريكية للغات الحديثة (MLA)
Li, Yanyi…[et al.]. Adoption of Machine Learning in Intelligent Terrain Classification of Hyperspectral Remote Sensing Images. Computational Intelligence and Neuroscience No. 2020 (2020), pp.1-13.
https://search.emarefa.net/detail/BIM-1138948
نمط استشهاد الجمعية الطبية الأمريكية (AMA)
Li, Yanyi& Wang, Jian& Gao, Tong& Sun, Qiwen& Zhang, Liguo& Tang, Mingxiu. Adoption of Machine Learning in Intelligent Terrain Classification of Hyperspectral Remote Sensing Images. Computational Intelligence and Neuroscience. 2020. Vol. 2020, no. 2020, pp.1-13.
https://search.emarefa.net/detail/BIM-1138948
نوع البيانات
مقالات
لغة النص
الإنجليزية
الملاحظات
Includes bibliographical references
رقم السجل
BIM-1138948
قاعدة معامل التأثير والاستشهادات المرجعية العربي "ارسيف Arcif"
أضخم قاعدة بيانات عربية للاستشهادات المرجعية للمجلات العلمية المحكمة الصادرة في العالم العربي
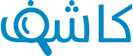
تقوم هذه الخدمة بالتحقق من التشابه أو الانتحال في الأبحاث والمقالات العلمية والأطروحات الجامعية والكتب والأبحاث باللغة العربية، وتحديد درجة التشابه أو أصالة الأعمال البحثية وحماية ملكيتها الفكرية. تعرف اكثر
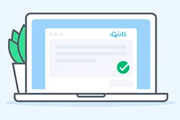