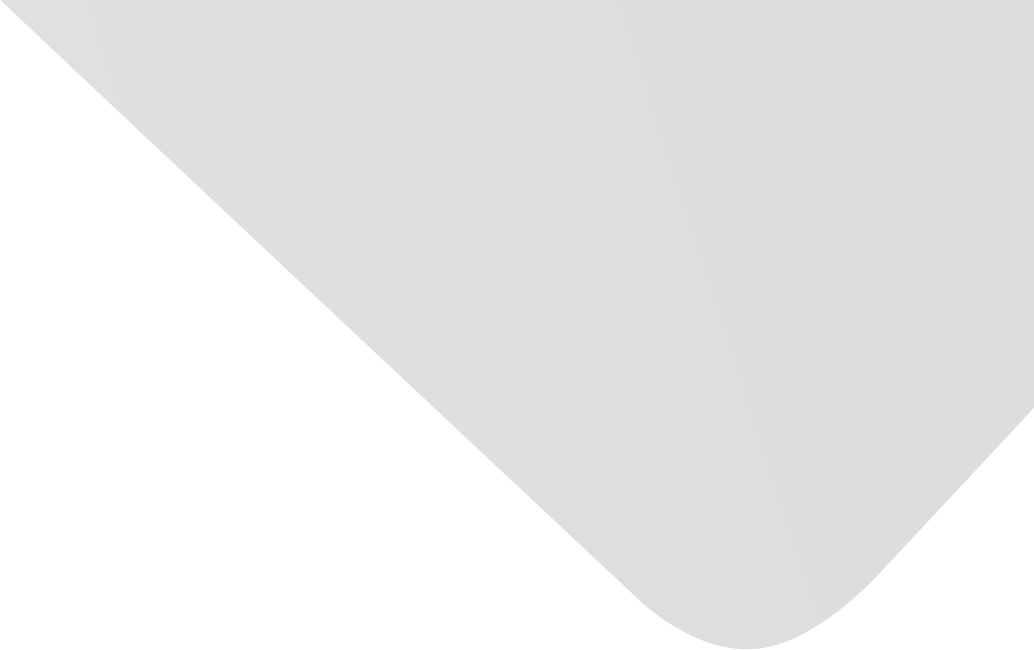
Diagnostic Accuracy of Machine Learning-Based Radiomics in Grading Gliomas: Systematic Review and Meta-Analysis
المؤلفون المشاركون
Sohn, Curtis K.
Bisdas, Sotirios
المصدر
Contrast Media & Molecular Imaging
العدد
المجلد 2020، العدد 2020 (31 ديسمبر/كانون الأول 2020)، ص ص. 1-12، 12ص.
الناشر
Hindawi Publishing Corporation
تاريخ النشر
2020-12-18
دولة النشر
مصر
عدد الصفحات
12
التخصصات الرئيسية
الملخص EN
Purpose.
This study aimed to estimate the diagnostic accuracy of machine learning- (ML-) based radiomics in differentiating high-grade gliomas (HGG) from low-grade gliomas (LGG) and to identify potential covariates that could affect the diagnostic accuracy of ML-based radiomic analysis in classifying gliomas.
Method.
A primary literature search of the PubMed database was conducted to find all related literatures in English between January 1, 2009, and May 1, 2020, with combining synonyms for “machine learning,” “glioma,” and “radiomics.” Five retrospective designed original articles including LGG and HGG subjects were chosen.
Pooled sensitivity, specificity, their 95% confidence interval, area under curve (AUC), and hierarchical summary receiver-operating characteristic (HSROC) models were obtained.
Result.
The pooled sensitivity when diagnosing HGG was higher (96% (95% CI: 0.93, 0.98)) than the specificity when diagnosing LGG (90% (95% CI 0.85, 0.93)).
Heterogeneity was observed in both sensitivity and specificity.
Metaregression confirmed the heterogeneity in sample sizes (p=0.05), imaging sequence types (p=0.02), and data sources (p=0.01), but not for the inclusion of the testing set (p=0.19), feature extraction number (p=0.36), and selection of feature number (p=0.18).
The results of subgroup analysis indicate that sample sizes of more than 100 and feature selection numbers less than the total sample size positively affected the diagnostic performance in differentiating HGG from LGG.
Conclusion.
This study demonstrates the excellent diagnostic performance of ML-based radiomics in differentiating HGG from LGG.
نمط استشهاد جمعية علماء النفس الأمريكية (APA)
Sohn, Curtis K.& Bisdas, Sotirios. 2020. Diagnostic Accuracy of Machine Learning-Based Radiomics in Grading Gliomas: Systematic Review and Meta-Analysis. Contrast Media & Molecular Imaging،Vol. 2020, no. 2020, pp.1-12.
https://search.emarefa.net/detail/BIM-1139229
نمط استشهاد الجمعية الأمريكية للغات الحديثة (MLA)
Sohn, Curtis K.& Bisdas, Sotirios. Diagnostic Accuracy of Machine Learning-Based Radiomics in Grading Gliomas: Systematic Review and Meta-Analysis. Contrast Media & Molecular Imaging No. 2020 (2020), pp.1-12.
https://search.emarefa.net/detail/BIM-1139229
نمط استشهاد الجمعية الطبية الأمريكية (AMA)
Sohn, Curtis K.& Bisdas, Sotirios. Diagnostic Accuracy of Machine Learning-Based Radiomics in Grading Gliomas: Systematic Review and Meta-Analysis. Contrast Media & Molecular Imaging. 2020. Vol. 2020, no. 2020, pp.1-12.
https://search.emarefa.net/detail/BIM-1139229
نوع البيانات
مقالات
لغة النص
الإنجليزية
الملاحظات
Includes bibliographical references
رقم السجل
BIM-1139229
قاعدة معامل التأثير والاستشهادات المرجعية العربي "ارسيف Arcif"
أضخم قاعدة بيانات عربية للاستشهادات المرجعية للمجلات العلمية المحكمة الصادرة في العالم العربي

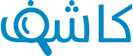
تقوم هذه الخدمة بالتحقق من التشابه أو الانتحال في الأبحاث والمقالات العلمية والأطروحات الجامعية والكتب والأبحاث باللغة العربية، وتحديد درجة التشابه أو أصالة الأعمال البحثية وحماية ملكيتها الفكرية. تعرف اكثر
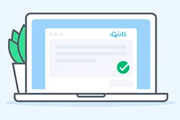