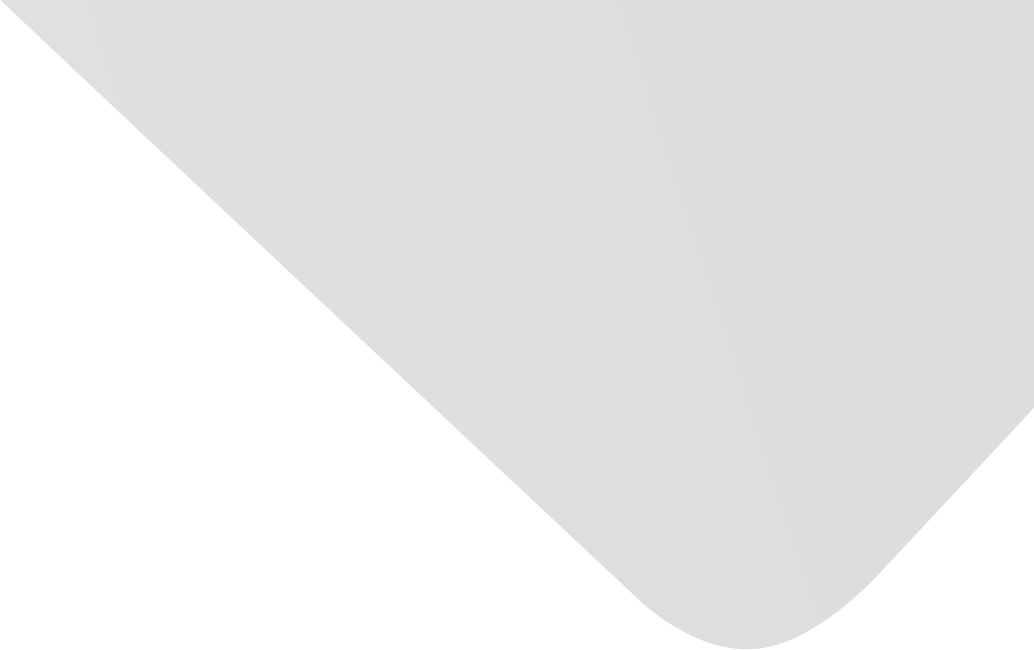
Multicenter Computer-Aided Diagnosis for Lymph Nodes Using Unsupervised Domain-Adaptation Networks Based on Cross-Domain Confounding Representations
المؤلفون المشاركون
Shi, Dapeng
Jiang, Lingyun
Chen, Jian
Qin, RuoXi
Zhang, Huike
Qiao, Kai
Hai, Jinjin
Xu, Junling
Yan, Bin
المصدر
Computational and Mathematical Methods in Medicine
العدد
المجلد 2020، العدد 2020 (31 ديسمبر/كانون الأول 2020)، ص ص. 1-10، 10ص.
الناشر
Hindawi Publishing Corporation
تاريخ النشر
2020-01-24
دولة النشر
مصر
عدد الصفحات
10
التخصصات الرئيسية
الملخص EN
To achieve the robust high-performance computer-aided diagnosis systems for lymph nodes, CT images may be typically collected from multicenter data, which cause the isolated performance of the model based on different data source centers.
The variability adaptation problem of lymph node data which is related to the problem of domain adaptation in deep learning differs from the general domain adaptation problem because of the typically larger CT image size and more complex data distributions.
Therefore, domain adaptation for this problem needs to consider the shared feature representation and even the conditioning information of each domain so that the adaptation network can capture significant discriminative representations in a domain-invariant space.
This paper extracts domain-invariant features based on a cross-domain confounding representation and proposes a cycle-consistency learning framework to encourage the network to preserve class-conditioning information through cross-domain image translations.
Compared with the performance of different domain adaptation methods, the accurate rate of our method achieves at least 4.4% points higher under multicenter lymph node data.
The pixel-level cross-domain image mapping and the semantic-level cycle consistency provided a stable confounding representation with class-conditioning information to achieve effective domain adaptation under complex feature distribution.
نمط استشهاد جمعية علماء النفس الأمريكية (APA)
Qin, RuoXi& Zhang, Huike& Jiang, Lingyun& Qiao, Kai& Hai, Jinjin& Chen, Jian…[et al.]. 2020. Multicenter Computer-Aided Diagnosis for Lymph Nodes Using Unsupervised Domain-Adaptation Networks Based on Cross-Domain Confounding Representations. Computational and Mathematical Methods in Medicine،Vol. 2020, no. 2020, pp.1-10.
https://search.emarefa.net/detail/BIM-1139410
نمط استشهاد الجمعية الأمريكية للغات الحديثة (MLA)
Qin, RuoXi…[et al.]. Multicenter Computer-Aided Diagnosis for Lymph Nodes Using Unsupervised Domain-Adaptation Networks Based on Cross-Domain Confounding Representations. Computational and Mathematical Methods in Medicine No. 2020 (2020), pp.1-10.
https://search.emarefa.net/detail/BIM-1139410
نمط استشهاد الجمعية الطبية الأمريكية (AMA)
Qin, RuoXi& Zhang, Huike& Jiang, Lingyun& Qiao, Kai& Hai, Jinjin& Chen, Jian…[et al.]. Multicenter Computer-Aided Diagnosis for Lymph Nodes Using Unsupervised Domain-Adaptation Networks Based on Cross-Domain Confounding Representations. Computational and Mathematical Methods in Medicine. 2020. Vol. 2020, no. 2020, pp.1-10.
https://search.emarefa.net/detail/BIM-1139410
نوع البيانات
مقالات
لغة النص
الإنجليزية
الملاحظات
Includes bibliographical references
رقم السجل
BIM-1139410
قاعدة معامل التأثير والاستشهادات المرجعية العربي "ارسيف Arcif"
أضخم قاعدة بيانات عربية للاستشهادات المرجعية للمجلات العلمية المحكمة الصادرة في العالم العربي
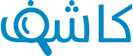
تقوم هذه الخدمة بالتحقق من التشابه أو الانتحال في الأبحاث والمقالات العلمية والأطروحات الجامعية والكتب والأبحاث باللغة العربية، وتحديد درجة التشابه أو أصالة الأعمال البحثية وحماية ملكيتها الفكرية. تعرف اكثر
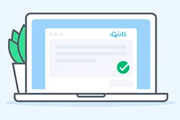