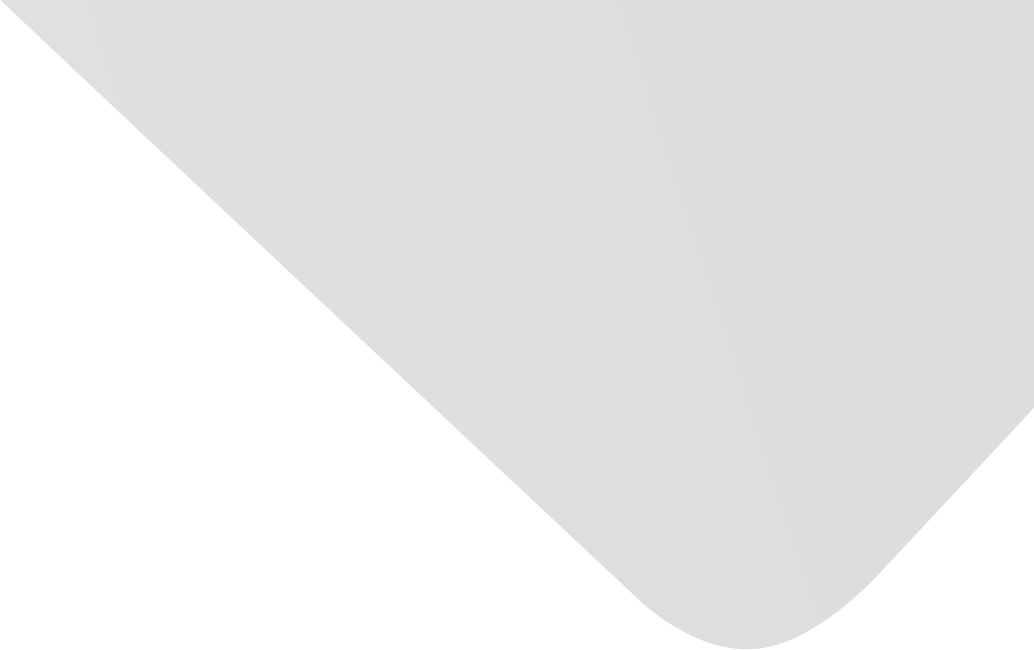
Applying Deep Neural Networks over Homomorphic Encrypted Medical Data
المؤلفون المشاركون
Itu, L. M.
Vizitiu, Anamaria
Niƫă, Cosmin Ioan
Puiu, Andrei
Suciu, Constantin
المصدر
Computational and Mathematical Methods in Medicine
العدد
المجلد 2020، العدد 2020 (31 ديسمبر/كانون الأول 2020)، ص ص. 1-26، 26ص.
الناشر
Hindawi Publishing Corporation
تاريخ النشر
2020-04-09
دولة النشر
مصر
عدد الصفحات
26
التخصصات الرئيسية
الملخص EN
In recent years, powered by state-of-the-art achievements in a broad range of areas, machine learning has received considerable attention from the healthcare sector.
Despite their ability to provide solutions within personalized medicine, strict regulations on the confidentiality of patient health information have in many cases hindered the adoption of deep learning-based solutions in clinical workflows.
To allow for the processing of sensitive health information without disclosing the underlying data, we propose a solution based on fully homomorphic encryption (FHE).
The considered encryption scheme, MORE (Matrix Operation for Randomization or Encryption), enables the computations within a neural network model to be directly performed on floating point data with a relatively small computational overhead.
We consider the well-known MNIST digit recognition problem to evaluate the feasibility of the proposed method and show that performance does not decrease when deep learning is applied on MORE homomorphic data.
To further evaluate the suitability of the method for healthcare applications, we first train a model on encrypted data to estimate the outputs of a whole-body circulation (WBC) hemodynamic model and then provide a solution for classifying encrypted X-ray coronary angiography medical images.
The findings highlight the potential of the proposed privacy-preserving deep learning methods to outperform existing approaches by providing, within a reasonable amount of time, results equivalent to those achieved by unencrypted models.
Lastly, we discuss the security implications of the encryption scheme and show that while the considered cryptosystem promotes efficiency and utility at a lower security level, it is still applicable in certain practical use cases.
نمط استشهاد جمعية علماء النفس الأمريكية (APA)
Vizitiu, Anamaria& Niƫă, Cosmin Ioan& Puiu, Andrei& Suciu, Constantin& Itu, L. M.. 2020. Applying Deep Neural Networks over Homomorphic Encrypted Medical Data. Computational and Mathematical Methods in Medicine،Vol. 2020, no. 2020, pp.1-26.
https://search.emarefa.net/detail/BIM-1139414
نمط استشهاد الجمعية الأمريكية للغات الحديثة (MLA)
Vizitiu, Anamaria…[et al.]. Applying Deep Neural Networks over Homomorphic Encrypted Medical Data. Computational and Mathematical Methods in Medicine No. 2020 (2020), pp.1-26.
https://search.emarefa.net/detail/BIM-1139414
نمط استشهاد الجمعية الطبية الأمريكية (AMA)
Vizitiu, Anamaria& Niƫă, Cosmin Ioan& Puiu, Andrei& Suciu, Constantin& Itu, L. M.. Applying Deep Neural Networks over Homomorphic Encrypted Medical Data. Computational and Mathematical Methods in Medicine. 2020. Vol. 2020, no. 2020, pp.1-26.
https://search.emarefa.net/detail/BIM-1139414
نوع البيانات
مقالات
لغة النص
الإنجليزية
الملاحظات
Includes bibliographical references
رقم السجل
BIM-1139414
قاعدة معامل التأثير والاستشهادات المرجعية العربي "ارسيف Arcif"
أضخم قاعدة بيانات عربية للاستشهادات المرجعية للمجلات العلمية المحكمة الصادرة في العالم العربي
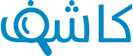
تقوم هذه الخدمة بالتحقق من التشابه أو الانتحال في الأبحاث والمقالات العلمية والأطروحات الجامعية والكتب والأبحاث باللغة العربية، وتحديد درجة التشابه أو أصالة الأعمال البحثية وحماية ملكيتها الفكرية. تعرف اكثر
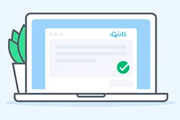