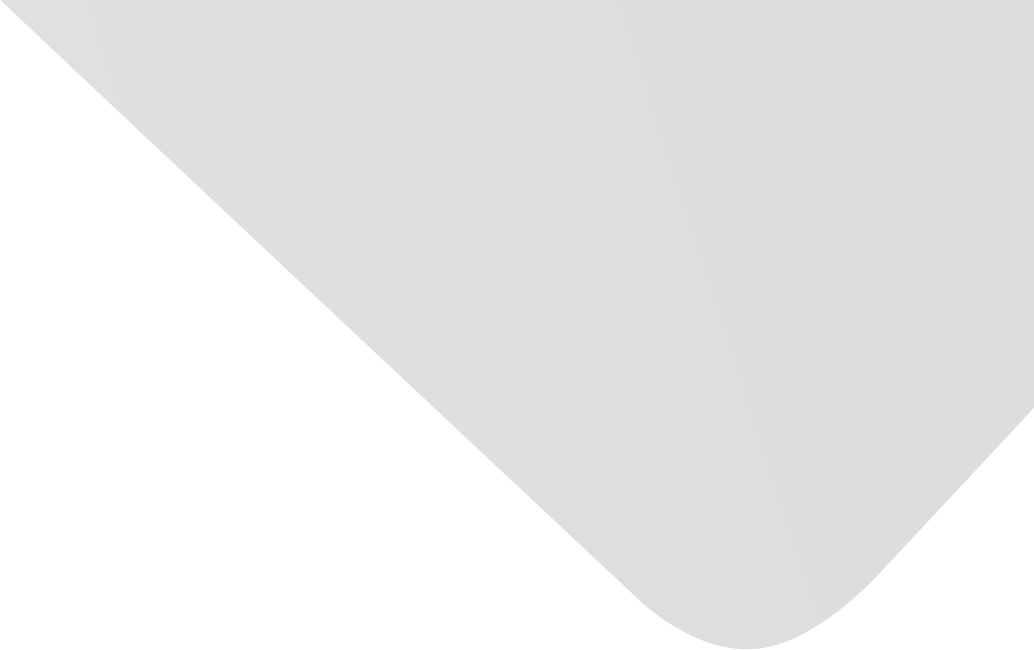
Multimodal MRI Brain Tumor Image Segmentation Using Sparse Subspace Clustering Algorithm
المؤلفون المشاركون
Liu, Li
Kuang, Liang
Ji, Yunfeng
المصدر
Computational and Mathematical Methods in Medicine
العدد
المجلد 2020، العدد 2020 (31 ديسمبر/كانون الأول 2020)، ص ص. 1-13، 13ص.
الناشر
Hindawi Publishing Corporation
تاريخ النشر
2020-07-04
دولة النشر
مصر
عدد الصفحات
13
التخصصات الرئيسية
الملخص EN
Brain tumors are one of the most deadly diseases with a high mortality rate.
The shape and size of the tumor are random during the growth process.
Brain tumor segmentation is a brain tumor assisted diagnosis technology that separates different brain tumor structures such as edema and active and tumor necrosis tissues from normal brain tissue.
Magnetic resonance imaging (MRI) technology has the advantages of no radiation impact on the human body, good imaging effect on structural tissues, and an ability to realize tomographic imaging of any orientation.
Therefore, doctors often use MRI brain tumor images to analyze and process brain tumors.
In these images, the tumor structure is only characterized by grayscale changes, and the developed images obtained by different equipment and different conditions may also be different.
This makes it difficult for traditional image segmentation methods to deal well with the segmentation of brain tumor images.
Considering that the traditional single-mode MRI brain tumor images contain incomplete brain tumor information, it is difficult to segment the single-mode brain tumor images to meet clinical needs.
In this paper, a sparse subspace clustering (SSC) algorithm is introduced to process the diagnosis of multimodal MRI brain tumor images.
In the absence of added noise, the proposed algorithm has better advantages than traditional methods.
Compared with the top 15 in the Brats 2015 competition, the accuracy is not much different, being basically stable between 10 and 15.
In order to verify the noise resistance of the proposed algorithm, this paper adds 5%, 10%, 15%, and 20% Gaussian noise to the test image.
Experimental results show that the proposed algorithm has better noise immunity than a comparable algorithm.
نمط استشهاد جمعية علماء النفس الأمريكية (APA)
Liu, Li& Kuang, Liang& Ji, Yunfeng. 2020. Multimodal MRI Brain Tumor Image Segmentation Using Sparse Subspace Clustering Algorithm. Computational and Mathematical Methods in Medicine،Vol. 2020, no. 2020, pp.1-13.
https://search.emarefa.net/detail/BIM-1139617
نمط استشهاد الجمعية الأمريكية للغات الحديثة (MLA)
Liu, Li…[et al.]. Multimodal MRI Brain Tumor Image Segmentation Using Sparse Subspace Clustering Algorithm. Computational and Mathematical Methods in Medicine No. 2020 (2020), pp.1-13.
https://search.emarefa.net/detail/BIM-1139617
نمط استشهاد الجمعية الطبية الأمريكية (AMA)
Liu, Li& Kuang, Liang& Ji, Yunfeng. Multimodal MRI Brain Tumor Image Segmentation Using Sparse Subspace Clustering Algorithm. Computational and Mathematical Methods in Medicine. 2020. Vol. 2020, no. 2020, pp.1-13.
https://search.emarefa.net/detail/BIM-1139617
نوع البيانات
مقالات
لغة النص
الإنجليزية
الملاحظات
Includes bibliographical references
رقم السجل
BIM-1139617
قاعدة معامل التأثير والاستشهادات المرجعية العربي "ارسيف Arcif"
أضخم قاعدة بيانات عربية للاستشهادات المرجعية للمجلات العلمية المحكمة الصادرة في العالم العربي
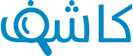
تقوم هذه الخدمة بالتحقق من التشابه أو الانتحال في الأبحاث والمقالات العلمية والأطروحات الجامعية والكتب والأبحاث باللغة العربية، وتحديد درجة التشابه أو أصالة الأعمال البحثية وحماية ملكيتها الفكرية. تعرف اكثر
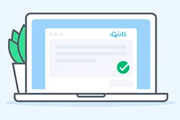