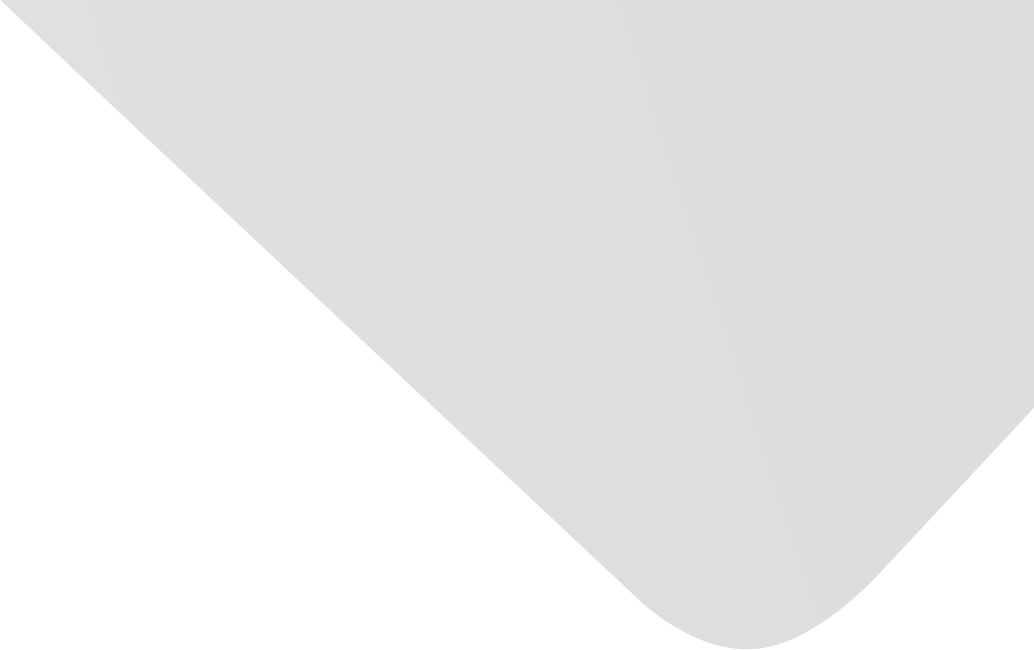
Learning to Make Document Context-Aware Recommendation with Joint Convolutional Matrix Factorization
المؤلفون المشاركون
Liu, Xiyu
Guo, Lei
Jiang, Haoran
Han, Yu
Yang, Xinxin
Wang, Xinhua
المصدر
العدد
المجلد 2020، العدد 2020 (31 ديسمبر/كانون الأول 2020)، ص ص. 1-15، 15ص.
الناشر
Hindawi Publishing Corporation
تاريخ النشر
2020-01-27
دولة النشر
مصر
عدد الصفحات
15
التخصصات الرئيسية
الملخص EN
Context-aware recommendation (CR) is the task of recommending relevant items by exploring the context information in online systems to alleviate the data sparsity issue of the user-item data.
Prior methods mainly studied CR by document-based modeling approaches, that is, making recommendations by additionally utilizing textual data such as reviews, abstracts, or synopses.
However, due to the inherent limitation of the bag-of-words model, they cannot effectively utilize contextual information of the documents, which results in a shallow understanding of the documents.
Recent works argued that the understanding of document context can be improved by the convolutional neural network (CNN) and proposed the convolutional matrix factorization (ConvMF) to leverage the contextual information of documents to enhance the rating prediction accuracy.
However, ConvMF only models the document content context from an item view and assumes users are independent and identically distributed (i.i.d).
But in reality, as we often turn to our friends for recommendations, the social relationship and social reviews are two important factors that can change our mind most.
Moreover, users are more inclined to interact (buy or click) with the items that they have bought (or clicked).
The relationships among items are also important factors that can impact the user’s final decision.
Based on the above observations, in this work, we target CR and propose a joint convolutional matrix factorization (JCMF) method to tackle the encountered challenges, which jointly considers the item’s reviews, item’s relationships, user’s social influence, and user’s reviews in a unified framework.
More specifically, to explore items’ relationships, we introduce a predefined item relation network into ConvMF by a shared item latent factor and propose a method called convolutional matrix factorization with item relations (CMF-I).
To consider user’s social influence, we further integrate the user’s social network into CMF-I by sharing the user latent factor between user’s social network and user-item rating matrix, which can be treated as a regularization term to constrain the recommendation process.
Finally, to model the document contextual information of user’s reviews, we exploit another CNN to learn user’s content representations and achieve our final model JCMF.
We conduct extensive experiments on the real-world dataset from Yelp.
The experimental results demonstrate the superiority of JCMF compared to several state-of-the-art methods in terms of root mean squared error (RMSE) and mean average error (MAE).
نمط استشهاد جمعية علماء النفس الأمريكية (APA)
Guo, Lei& Han, Yu& Jiang, Haoran& Yang, Xinxin& Wang, Xinhua& Liu, Xiyu. 2020. Learning to Make Document Context-Aware Recommendation with Joint Convolutional Matrix Factorization. Complexity،Vol. 2020, no. 2020, pp.1-15.
https://search.emarefa.net/detail/BIM-1139900
نمط استشهاد الجمعية الأمريكية للغات الحديثة (MLA)
Guo, Lei…[et al.]. Learning to Make Document Context-Aware Recommendation with Joint Convolutional Matrix Factorization. Complexity No. 2020 (2020), pp.1-15.
https://search.emarefa.net/detail/BIM-1139900
نمط استشهاد الجمعية الطبية الأمريكية (AMA)
Guo, Lei& Han, Yu& Jiang, Haoran& Yang, Xinxin& Wang, Xinhua& Liu, Xiyu. Learning to Make Document Context-Aware Recommendation with Joint Convolutional Matrix Factorization. Complexity. 2020. Vol. 2020, no. 2020, pp.1-15.
https://search.emarefa.net/detail/BIM-1139900
نوع البيانات
مقالات
لغة النص
الإنجليزية
الملاحظات
Includes bibliographical references
رقم السجل
BIM-1139900
قاعدة معامل التأثير والاستشهادات المرجعية العربي "ارسيف Arcif"
أضخم قاعدة بيانات عربية للاستشهادات المرجعية للمجلات العلمية المحكمة الصادرة في العالم العربي
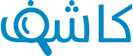
تقوم هذه الخدمة بالتحقق من التشابه أو الانتحال في الأبحاث والمقالات العلمية والأطروحات الجامعية والكتب والأبحاث باللغة العربية، وتحديد درجة التشابه أو أصالة الأعمال البحثية وحماية ملكيتها الفكرية. تعرف اكثر
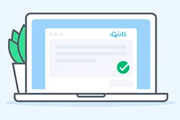