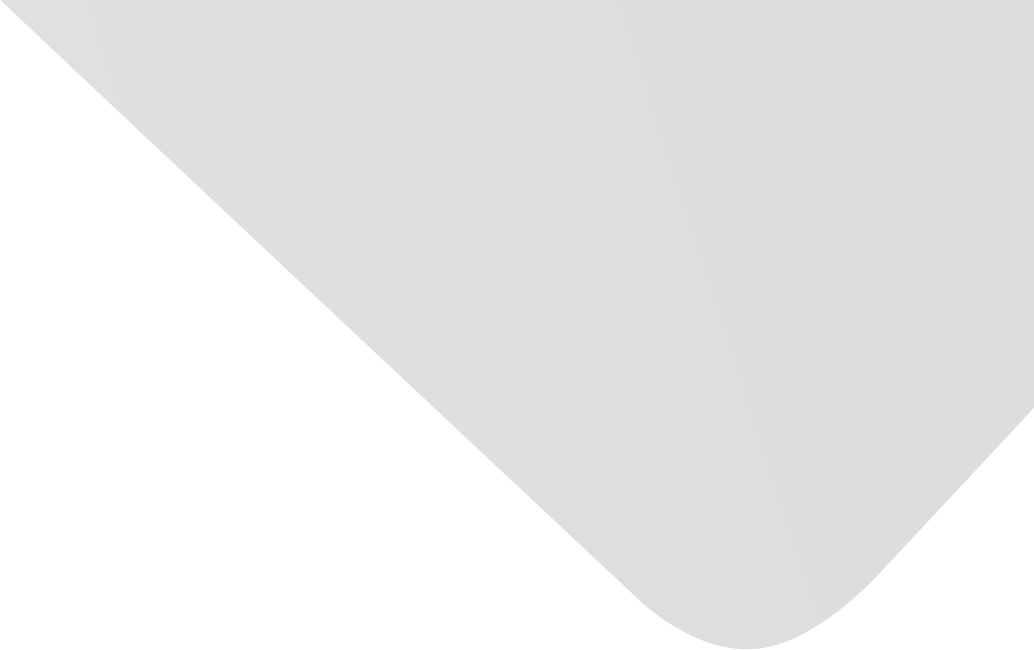
IPF-LASSO: Integrative L1-Penalized Regression with Penalty Factors for Prediction Based on Multi-Omics Data
المؤلفون المشاركون
Boulesteix, Anne-Laure
De Bin, Riccardo
Jiang, Xiaoyu
Fuchs, Mathias
المصدر
Computational and Mathematical Methods in Medicine
العدد
المجلد 2017، العدد 2017 (31 ديسمبر/كانون الأول 2017)، ص ص. 1-14، 14ص.
الناشر
Hindawi Publishing Corporation
تاريخ النشر
2017-05-04
دولة النشر
مصر
عدد الصفحات
14
التخصصات الرئيسية
الملخص EN
As modern biotechnologies advance, it has become increasingly frequent that different modalities of high-dimensional molecular data (termed “omics” data in this paper), such as gene expression, methylation, and copy number, are collected from the same patient cohort to predict the clinical outcome.
While prediction based on omics data has been widely studied in the last fifteen years, little has been done in the statistical literature on the integration of multiple omics modalities to select a subset of variables for prediction, which is a critical task in personalized medicine.
In this paper, we propose a simple penalized regression method to address this problem by assigning different penalty factors to different data modalities for feature selection and prediction.
The penalty factors can be chosen in a fully data-driven fashion by cross-validation or by taking practical considerations into account.
In simulation studies, we compare the prediction performance of our approach, called IPF-LASSO (Integrative LASSO with Penalty Factors) and implemented in the R package ipflasso, with the standard LASSO and sparse group LASSO.
The use of IPF-LASSO is also illustrated through applications to two real-life cancer datasets.
All data and codes are available on the companion website to ensure reproducibility.
نمط استشهاد جمعية علماء النفس الأمريكية (APA)
Boulesteix, Anne-Laure& De Bin, Riccardo& Jiang, Xiaoyu& Fuchs, Mathias. 2017. IPF-LASSO: Integrative L1-Penalized Regression with Penalty Factors for Prediction Based on Multi-Omics Data. Computational and Mathematical Methods in Medicine،Vol. 2017, no. 2017, pp.1-14.
https://search.emarefa.net/detail/BIM-1142316
نمط استشهاد الجمعية الأمريكية للغات الحديثة (MLA)
Boulesteix, Anne-Laure…[et al.]. IPF-LASSO: Integrative L1-Penalized Regression with Penalty Factors for Prediction Based on Multi-Omics Data. Computational and Mathematical Methods in Medicine No. 2017 (2017), pp.1-14.
https://search.emarefa.net/detail/BIM-1142316
نمط استشهاد الجمعية الطبية الأمريكية (AMA)
Boulesteix, Anne-Laure& De Bin, Riccardo& Jiang, Xiaoyu& Fuchs, Mathias. IPF-LASSO: Integrative L1-Penalized Regression with Penalty Factors for Prediction Based on Multi-Omics Data. Computational and Mathematical Methods in Medicine. 2017. Vol. 2017, no. 2017, pp.1-14.
https://search.emarefa.net/detail/BIM-1142316
نوع البيانات
مقالات
لغة النص
الإنجليزية
الملاحظات
Includes bibliographical references
رقم السجل
BIM-1142316
قاعدة معامل التأثير والاستشهادات المرجعية العربي "ارسيف Arcif"
أضخم قاعدة بيانات عربية للاستشهادات المرجعية للمجلات العلمية المحكمة الصادرة في العالم العربي
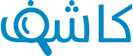
تقوم هذه الخدمة بالتحقق من التشابه أو الانتحال في الأبحاث والمقالات العلمية والأطروحات الجامعية والكتب والأبحاث باللغة العربية، وتحديد درجة التشابه أو أصالة الأعمال البحثية وحماية ملكيتها الفكرية. تعرف اكثر
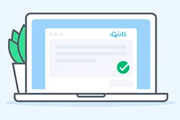