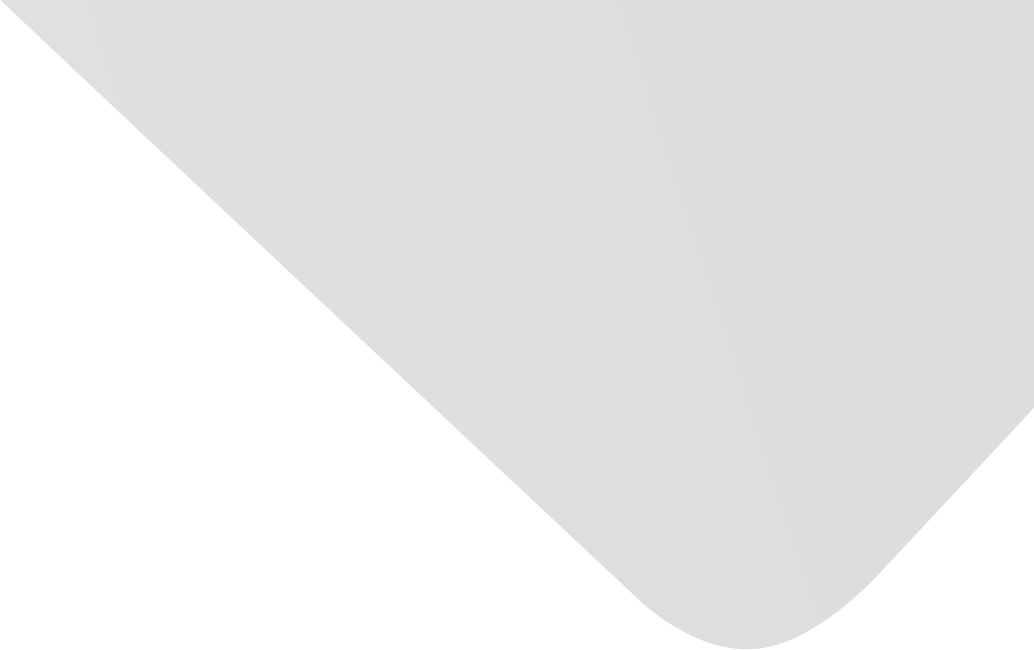
Adaptive Hybrid Soft-Sensor Model of Grinding Process Based on Regularized Extreme Learning Machine and Least Squares Support Vector Machine Optimized by Golden Sine Harris Hawk Optimization Algorithm
المؤلفون المشاركون
Xie, Wei
Xing, Cheng
Guo, Sha-Sha
Guo, Meng-wei
Zhu, Ling-feng
Wang, Jie-sheng
المصدر
العدد
المجلد 2020، العدد 2020 (31 ديسمبر/كانون الأول 2020)، ص ص. 1-26، 26ص.
الناشر
Hindawi Publishing Corporation
تاريخ النشر
2020-05-28
دولة النشر
مصر
عدد الصفحات
26
التخصصات الرئيسية
الملخص EN
Soft-sensor technology plays a vital role in tracking and monitoring the key production indicators of the grinding and classifying process.
Least squares support vector machine (LSSVM), as a soft-sensor model with strong generalization ability, can be used to predict key production indicators in complex grinding processes.
The traditional crossvalidation method cannot obtain the ideal structure parameters of LSSVM.
In order to improve the prediction accuracy of LSSVM, a golden sine Harris Hawk optimization (GSHHO) algorithm was proposed to optimize the structure parameters of LSSVM models with linear kernel, sigmoid kernel, polynomial kernel, and radial basis kernel, and the influences of GSHHO algorithm on the prediction accuracy under these LSSVM models were studied.
In order to deal with the problem that the prediction accuracy of the model decreases due to changes of industrial status, this paper adopts moving window (MW) strategy to adaptively revise the LSSVM (MW-LSSVM), which greatly improves the prediction accuracy of the LSSVM.
The prediction accuracy of the regularized extreme learning machine with MW strategy (MW-RELM) is higher than that of MW-LSSVM at some moments.
Based on the training errors of LSSVM and RELM within the window, this paper proposes an adaptive hybrid soft-sensing model that switches between LSSVM and RELM.
Compared with the previous MW-LSSVM, MW-neural network trained with extended Kalman filter(MW-KNN), and MW-RELM, the prediction accuracy of the hybrid model is further improved.
Simulation results show that the proposed hybrid adaptive soft-sensor model has good generalization ability and prediction accuracy.
نمط استشهاد جمعية علماء النفس الأمريكية (APA)
Xie, Wei& Wang, Jie-sheng& Xing, Cheng& Guo, Sha-Sha& Guo, Meng-wei& Zhu, Ling-feng. 2020. Adaptive Hybrid Soft-Sensor Model of Grinding Process Based on Regularized Extreme Learning Machine and Least Squares Support Vector Machine Optimized by Golden Sine Harris Hawk Optimization Algorithm. Complexity،Vol. 2020, no. 2020, pp.1-26.
https://search.emarefa.net/detail/BIM-1142910
نمط استشهاد الجمعية الأمريكية للغات الحديثة (MLA)
Xie, Wei…[et al.]. Adaptive Hybrid Soft-Sensor Model of Grinding Process Based on Regularized Extreme Learning Machine and Least Squares Support Vector Machine Optimized by Golden Sine Harris Hawk Optimization Algorithm. Complexity No. 2020 (2020), pp.1-26.
https://search.emarefa.net/detail/BIM-1142910
نمط استشهاد الجمعية الطبية الأمريكية (AMA)
Xie, Wei& Wang, Jie-sheng& Xing, Cheng& Guo, Sha-Sha& Guo, Meng-wei& Zhu, Ling-feng. Adaptive Hybrid Soft-Sensor Model of Grinding Process Based on Regularized Extreme Learning Machine and Least Squares Support Vector Machine Optimized by Golden Sine Harris Hawk Optimization Algorithm. Complexity. 2020. Vol. 2020, no. 2020, pp.1-26.
https://search.emarefa.net/detail/BIM-1142910
نوع البيانات
مقالات
لغة النص
الإنجليزية
الملاحظات
Includes bibliographical references
رقم السجل
BIM-1142910
قاعدة معامل التأثير والاستشهادات المرجعية العربي "ارسيف Arcif"
أضخم قاعدة بيانات عربية للاستشهادات المرجعية للمجلات العلمية المحكمة الصادرة في العالم العربي
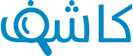
تقوم هذه الخدمة بالتحقق من التشابه أو الانتحال في الأبحاث والمقالات العلمية والأطروحات الجامعية والكتب والأبحاث باللغة العربية، وتحديد درجة التشابه أو أصالة الأعمال البحثية وحماية ملكيتها الفكرية. تعرف اكثر
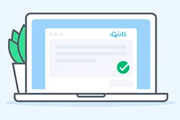