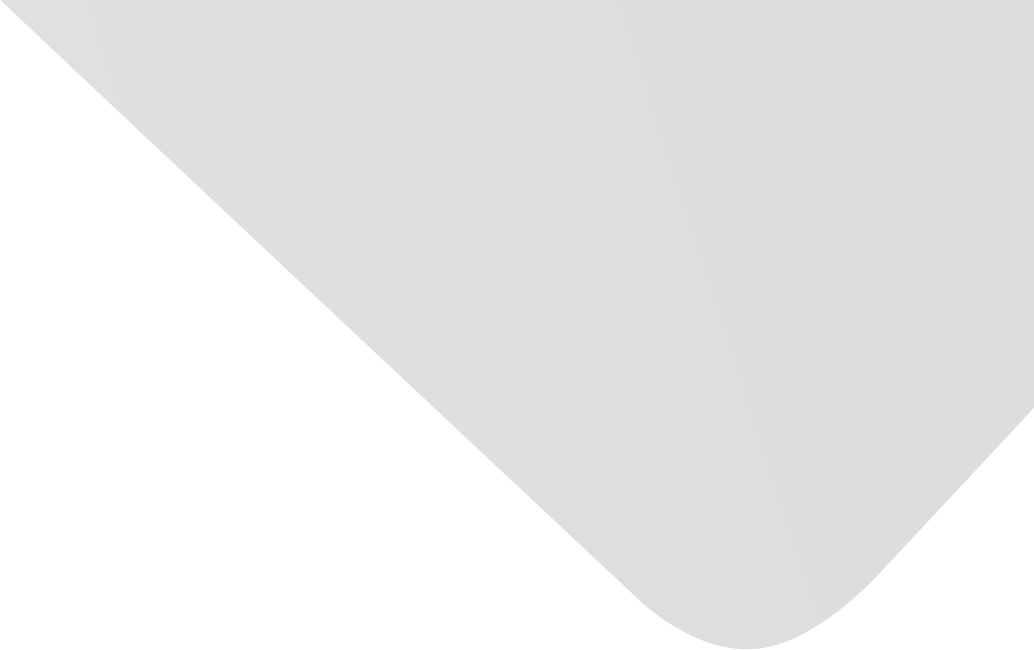
Semisupervised Community Preserving Network Embedding with Pairwise Constraints
المؤلفون المشاركون
Liu, Dong
Ru, Yan
Li, Qinpeng
Wang, Shibin
Niu, Jianwei
المصدر
العدد
المجلد 2020، العدد 2020 (31 ديسمبر/كانون الأول 2020)، ص ص. 1-14، 14ص.
الناشر
Hindawi Publishing Corporation
تاريخ النشر
2020-11-10
دولة النشر
مصر
عدد الصفحات
14
التخصصات الرئيسية
الملخص EN
Network embedding aims to learn the low-dimensional representations of nodes in networks.
It preserves the structure and internal attributes of the networks while representing nodes as low-dimensional dense real-valued vectors.
These vectors are used as inputs of machine learning algorithms for network analysis tasks such as node clustering, classification, link prediction, and network visualization.
The network embedding algorithms, which considered the community structure, impose a higher level of constraint on the similarity of nodes, and they make the learned node embedding results more discriminative.
However, the existing network representation learning algorithms are mostly unsupervised models; the pairwise constraint information, which represents community membership, is not effectively utilized to obtain node embedding results that are more consistent with prior knowledge.
This paper proposes a semisupervised modularized nonnegative matrix factorization model, SMNMF, while preserving the community structure for network embedding; the pairwise constraints (must-link and cannot-link) information are effectively fused with the adjacency matrix and node similarity matrix of the network so that the node representations learned by the model are more interpretable.
Experimental results on eight real network datasets show that, comparing with the representative network embedding methods, the node representations learned after incorporating the pairwise constraints can obtain higher accuracy in node clustering task and the results of link prediction, and network visualization tasks indicate that the semisupervised model SMNMF is more discriminative than unsupervised ones.
نمط استشهاد جمعية علماء النفس الأمريكية (APA)
Liu, Dong& Ru, Yan& Li, Qinpeng& Wang, Shibin& Niu, Jianwei. 2020. Semisupervised Community Preserving Network Embedding with Pairwise Constraints. Complexity،Vol. 2020, no. 2020, pp.1-14.
https://search.emarefa.net/detail/BIM-1143995
نمط استشهاد الجمعية الأمريكية للغات الحديثة (MLA)
Liu, Dong…[et al.]. Semisupervised Community Preserving Network Embedding with Pairwise Constraints. Complexity No. 2020 (2020), pp.1-14.
https://search.emarefa.net/detail/BIM-1143995
نمط استشهاد الجمعية الطبية الأمريكية (AMA)
Liu, Dong& Ru, Yan& Li, Qinpeng& Wang, Shibin& Niu, Jianwei. Semisupervised Community Preserving Network Embedding with Pairwise Constraints. Complexity. 2020. Vol. 2020, no. 2020, pp.1-14.
https://search.emarefa.net/detail/BIM-1143995
نوع البيانات
مقالات
لغة النص
الإنجليزية
الملاحظات
Includes bibliographical references
رقم السجل
BIM-1143995
قاعدة معامل التأثير والاستشهادات المرجعية العربي "ارسيف Arcif"
أضخم قاعدة بيانات عربية للاستشهادات المرجعية للمجلات العلمية المحكمة الصادرة في العالم العربي
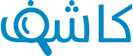
تقوم هذه الخدمة بالتحقق من التشابه أو الانتحال في الأبحاث والمقالات العلمية والأطروحات الجامعية والكتب والأبحاث باللغة العربية، وتحديد درجة التشابه أو أصالة الأعمال البحثية وحماية ملكيتها الفكرية. تعرف اكثر
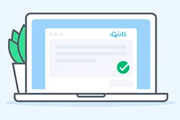