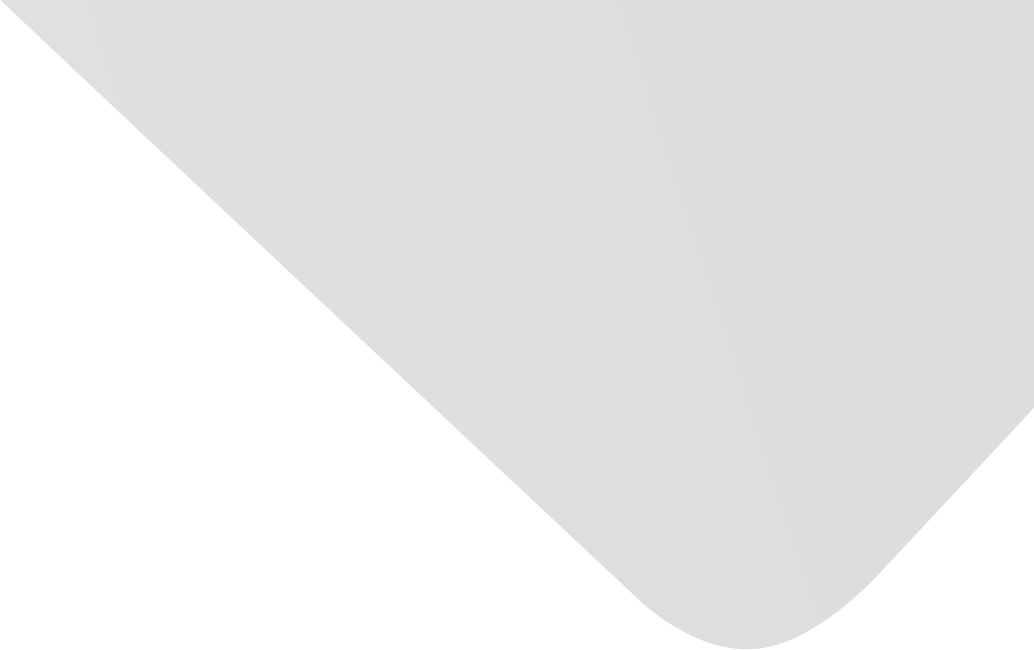
Deep Interest-Shifting Network with Meta-Embeddings for Fresh Item Recommendation
المؤلفون المشاركون
Zhang, Zhen
Zhang, Zhiqiang
Liu, Weiwei
Zhang, Ji
Li, Zhao
Wang, Haobo
Ding, Donghui
Hu, Shichang
Gao, Jianliang
المصدر
العدد
المجلد 2020، العدد 2020 (31 ديسمبر/كانون الأول 2020)، ص ص. 1-13، 13ص.
الناشر
Hindawi Publishing Corporation
تاريخ النشر
2020-10-28
دولة النشر
مصر
عدد الصفحات
13
التخصصات الرئيسية
الملخص EN
Nowadays, people have an increasing interest in fresh products such as new shoes and cosmetics.
To this end, an E-commerce platform Taobao launched a fresh-item hub page on the recommender system, with which customers can freely and exclusively explore and purchase fresh items, namely, the New Tendency page.
In this work, we make a first attempt to tackle the fresh-item recommendation task with two major challenges.
First, a fresh-item recommendation scenario usually faces the challenge that the training data are highly deficient due to low page views.
In this paper, we propose a deep interest-shifting network (DisNet), which transfers knowledge from a huge number of auxiliary data and then shifts user interests with contextual information.
Furthermore, three interpretable interest-shifting operators are introduced.
Second, since the items are fresh, many of them have never been exposed to users, leading to a severe cold-start problem.
Though this problem can be alleviated by knowledge transfer, we further babysit these fully cold-start items by a relational meta-Id-embedding generator (RM-IdEG).
Specifically, it trains the item id embeddings in a learning-to-learn manner and integrates relational information for better embedding performance.
We conducted comprehensive experiments on both synthetic datasets as well as a real-world dataset.
Both DisNet and RM-IdEG significantly outperform state-of-the-art approaches, respectively.
Empirical results clearly verify the effectiveness of the proposed techniques, which are arguably promising and scalable in real-world applications.
نمط استشهاد جمعية علماء النفس الأمريكية (APA)
Li, Zhao& Wang, Haobo& Ding, Donghui& Hu, Shichang& Zhang, Zhen& Liu, Weiwei…[et al.]. 2020. Deep Interest-Shifting Network with Meta-Embeddings for Fresh Item Recommendation. Complexity،Vol. 2020, no. 2020, pp.1-13.
https://search.emarefa.net/detail/BIM-1144720
نمط استشهاد الجمعية الأمريكية للغات الحديثة (MLA)
Li, Zhao…[et al.]. Deep Interest-Shifting Network with Meta-Embeddings for Fresh Item Recommendation. Complexity No. 2020 (2020), pp.1-13.
https://search.emarefa.net/detail/BIM-1144720
نمط استشهاد الجمعية الطبية الأمريكية (AMA)
Li, Zhao& Wang, Haobo& Ding, Donghui& Hu, Shichang& Zhang, Zhen& Liu, Weiwei…[et al.]. Deep Interest-Shifting Network with Meta-Embeddings for Fresh Item Recommendation. Complexity. 2020. Vol. 2020, no. 2020, pp.1-13.
https://search.emarefa.net/detail/BIM-1144720
نوع البيانات
مقالات
لغة النص
الإنجليزية
الملاحظات
Includes bibliographical references
رقم السجل
BIM-1144720
قاعدة معامل التأثير والاستشهادات المرجعية العربي "ارسيف Arcif"
أضخم قاعدة بيانات عربية للاستشهادات المرجعية للمجلات العلمية المحكمة الصادرة في العالم العربي

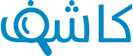
تقوم هذه الخدمة بالتحقق من التشابه أو الانتحال في الأبحاث والمقالات العلمية والأطروحات الجامعية والكتب والأبحاث باللغة العربية، وتحديد درجة التشابه أو أصالة الأعمال البحثية وحماية ملكيتها الفكرية. تعرف اكثر
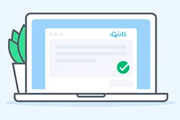