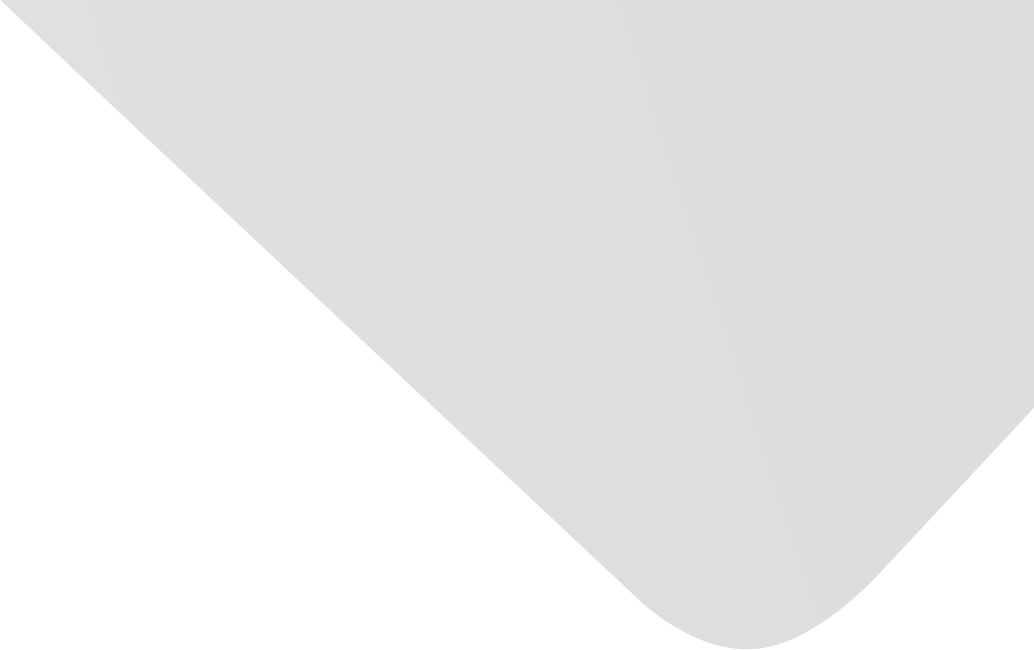
Ensemble Machine Learning Model for Classification of Spam Product Reviews
المؤلفون المشاركون
Uddin, M. Irfan
Khan, Atif
Fayaz, Muhammad
Rahman, Javid Ur
Alharbi, Abdullah
Alouffi, Bader
المصدر
العدد
المجلد 2020، العدد 2020 (31 ديسمبر/كانون الأول 2020)، ص ص. 1-10، 10ص.
الناشر
Hindawi Publishing Corporation
تاريخ النشر
2020-12-18
دولة النشر
مصر
عدد الصفحات
10
التخصصات الرئيسية
الملخص EN
Nowadays, online product reviews have been at the heart of the product assessment process for a company and its customers.
They give feedback to a company on improving product quality, planning, and monitoring its business schemes in order to increase sale and gain more profit.
They are also helpful for customers to select the right products in less effort and time.
Most companies make spam reviews of products in order to increase the products sales and gain more profit.
Detecting spam product reviews is a challenging issue in NLP (natural language processing).
Numerous machine learning approaches have attempted to detect and classify the product reviews as spam or nonspam.
However, in order to improve the classification accuracy, this study has introduced an ensemble machine learning model that combines predictions from multilayer perceptron (MLP), K-Nearest Neighbour (KNN), and Random Forest (RF) and predicts the outcome of the review as spam or real (nonspam), based on the majority vote of the contributing models.
In order to accomplish the task of spam review classification, the proposed ensemble and other benchmark boosting approaches are tested with 25 statistical features extracted from mobile application reviews of Yelp Dataset.
Then, three different selection techniques are exploited to diminish the feature space and filter out the top 10 optimal features.
The effectiveness of the proposed ensemble, the individual models, and other benchmark boosting approaches is again evaluated with 10 optimal features in terms of classification accuracy.
Experimental outcomes illustrate that the proposed ensemble model outperformed the individual classifiers (MLP, KNN, and RF) and state-of-the-art boosting approaches like Generalized Boost Regression Model (GBM), Extreme Gradient Boost (XGBoost), and AdaBoost Regression Model in terms of classification accuracy.
نمط استشهاد جمعية علماء النفس الأمريكية (APA)
Fayaz, Muhammad& Khan, Atif& Rahman, Javid Ur& Alharbi, Abdullah& Uddin, M. Irfan& Alouffi, Bader. 2020. Ensemble Machine Learning Model for Classification of Spam Product Reviews. Complexity،Vol. 2020, no. 2020, pp.1-10.
https://search.emarefa.net/detail/BIM-1144933
نمط استشهاد الجمعية الأمريكية للغات الحديثة (MLA)
Fayaz, Muhammad…[et al.]. Ensemble Machine Learning Model for Classification of Spam Product Reviews. Complexity No. 2020 (2020), pp.1-10.
https://search.emarefa.net/detail/BIM-1144933
نمط استشهاد الجمعية الطبية الأمريكية (AMA)
Fayaz, Muhammad& Khan, Atif& Rahman, Javid Ur& Alharbi, Abdullah& Uddin, M. Irfan& Alouffi, Bader. Ensemble Machine Learning Model for Classification of Spam Product Reviews. Complexity. 2020. Vol. 2020, no. 2020, pp.1-10.
https://search.emarefa.net/detail/BIM-1144933
نوع البيانات
مقالات
لغة النص
الإنجليزية
الملاحظات
Includes bibliographical references
رقم السجل
BIM-1144933
قاعدة معامل التأثير والاستشهادات المرجعية العربي "ارسيف Arcif"
أضخم قاعدة بيانات عربية للاستشهادات المرجعية للمجلات العلمية المحكمة الصادرة في العالم العربي

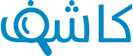
تقوم هذه الخدمة بالتحقق من التشابه أو الانتحال في الأبحاث والمقالات العلمية والأطروحات الجامعية والكتب والأبحاث باللغة العربية، وتحديد درجة التشابه أو أصالة الأعمال البحثية وحماية ملكيتها الفكرية. تعرف اكثر
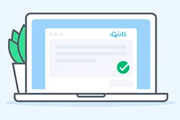