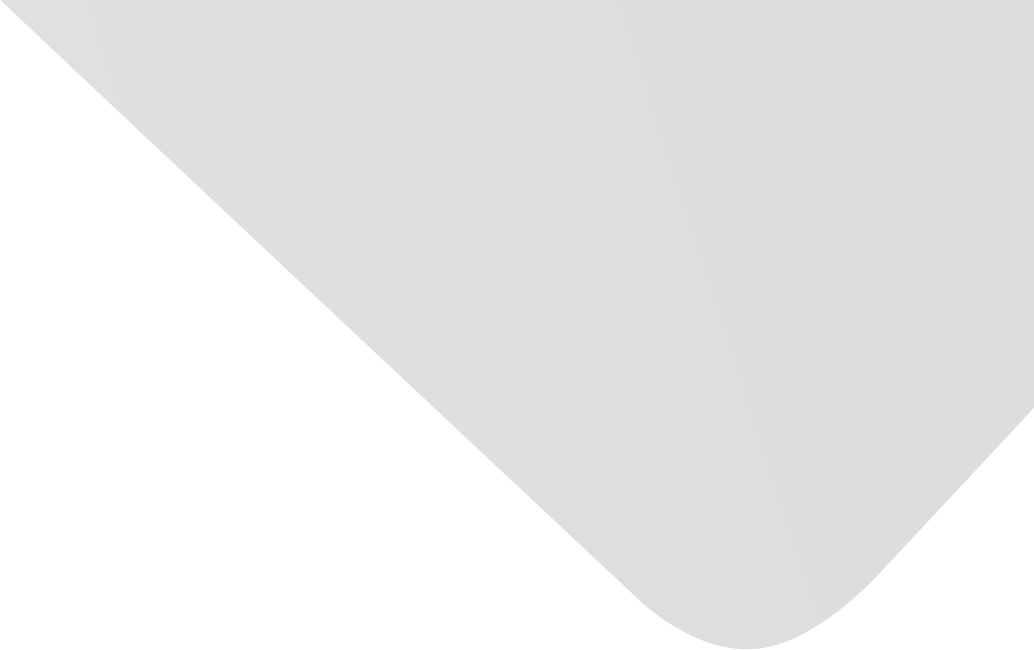
Univariate Time Series Analysis of Short-Term Forecasting Horizons Using Artificial Neural Networks: The Case of Public Ambulance Emergency Preparedness
المؤلفون المشاركون
Mapuwei, Tichaona W.
Bodhlyera, Oliver
Mwambi, Henry
المصدر
Journal of Applied Mathematics
العدد
المجلد 2020، العدد 2020 (31 ديسمبر/كانون الأول 2020)، ص ص. 1-11، 11ص.
الناشر
Hindawi Publishing Corporation
تاريخ النشر
2020-05-01
دولة النشر
مصر
عدد الصفحات
11
التخصصات الرئيسية
الملخص EN
This study examined the applicability of artificial neural network models in modelling univariate time series ambulance demand for short-term forecasting horizons in Zimbabwe.
Bulawayo City Councils’ ambulance services department was used as a case study.
Two models, feed-forward neural network (FFNN) and seasonal autoregressive integrated moving average, (SARIMA) were developed using monthly historical data from 2010 to 2017 and compared against observed data for 2018.
The mean absolute error (MAE), root mean square error (RMSE), and paired sample t-test were used as performance measures.
Calculated performance measures for FFNN were MAE (94.0), RMSE (137.19), and the test statistic value p=0.493(>0.05) whilst corresponding values for SARIMA were 105.71, 125.28, and p=0.005(<0.05), respectively.
Findings of this study suggest that the FFNN model is inclined to value estimation whilst the SARIMA model is directional with a linear pattern over time.
Based on the performance measures, the parsimonious FFNN model was selected to predict short-term annual ambulance demand.
Demand forecasts with FFNN for 2019 reflected the expected general trends in Bulawayo.
The forecasts indicate high demand during the months of January, March, September, and December.
Key ambulance logistic activities such as vehicle servicing, replenishment of essential equipment and drugs, staff training, leave days scheduling, and mock drills need to be planned for April, June, and July when low demand is anticipated.
This deliberate planning strategy would avoid a dire situation whereby ambulances are available but without adequate staff, essential drugs, and equipment to respond to public emergency calls.
نمط استشهاد جمعية علماء النفس الأمريكية (APA)
Mapuwei, Tichaona W.& Bodhlyera, Oliver& Mwambi, Henry. 2020. Univariate Time Series Analysis of Short-Term Forecasting Horizons Using Artificial Neural Networks: The Case of Public Ambulance Emergency Preparedness. Journal of Applied Mathematics،Vol. 2020, no. 2020, pp.1-11.
https://search.emarefa.net/detail/BIM-1174485
نمط استشهاد الجمعية الأمريكية للغات الحديثة (MLA)
Mapuwei, Tichaona W.…[et al.]. Univariate Time Series Analysis of Short-Term Forecasting Horizons Using Artificial Neural Networks: The Case of Public Ambulance Emergency Preparedness. Journal of Applied Mathematics No. 2020 (2020), pp.1-11.
https://search.emarefa.net/detail/BIM-1174485
نمط استشهاد الجمعية الطبية الأمريكية (AMA)
Mapuwei, Tichaona W.& Bodhlyera, Oliver& Mwambi, Henry. Univariate Time Series Analysis of Short-Term Forecasting Horizons Using Artificial Neural Networks: The Case of Public Ambulance Emergency Preparedness. Journal of Applied Mathematics. 2020. Vol. 2020, no. 2020, pp.1-11.
https://search.emarefa.net/detail/BIM-1174485
نوع البيانات
مقالات
لغة النص
الإنجليزية
الملاحظات
Includes bibliographical references
رقم السجل
BIM-1174485
قاعدة معامل التأثير والاستشهادات المرجعية العربي "ارسيف Arcif"
أضخم قاعدة بيانات عربية للاستشهادات المرجعية للمجلات العلمية المحكمة الصادرة في العالم العربي
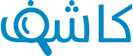
تقوم هذه الخدمة بالتحقق من التشابه أو الانتحال في الأبحاث والمقالات العلمية والأطروحات الجامعية والكتب والأبحاث باللغة العربية، وتحديد درجة التشابه أو أصالة الأعمال البحثية وحماية ملكيتها الفكرية. تعرف اكثر
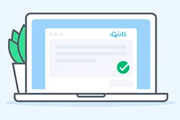