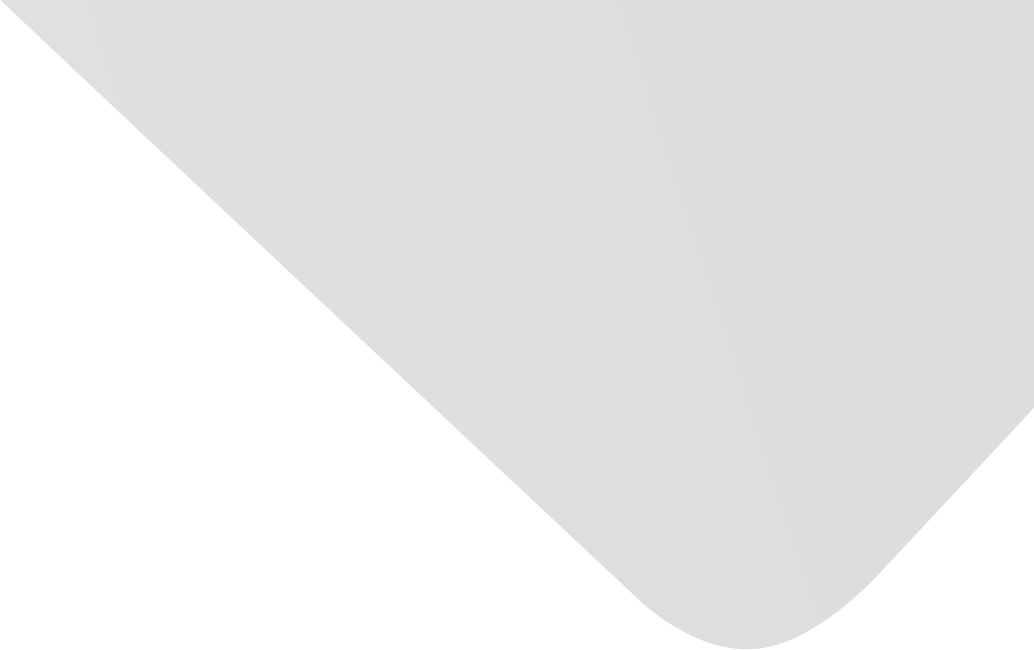
Automated Segmentation of Colorectal Tumor in 3D MRI Using 3D Multiscale Densely Connected Convolutional Neural Network
المؤلفون المشاركون
Schmid, Maurizio
Conforto, Silvia
Rengo, Marco
Soomro, Mumtaz Hussain
Giunta, Gaetano
Caruso, Damiano
Coppotelli, Matteo
Del Secco, Lorenzo
Neri, Emanuele
Laghi, Andrea
المصدر
Journal of Healthcare Engineering
العدد
المجلد 2019، العدد 2019 (31 ديسمبر/كانون الأول 2019)، ص ص. 1-11، 11ص.
الناشر
Hindawi Publishing Corporation
تاريخ النشر
2019-01-31
دولة النشر
مصر
عدد الصفحات
11
التخصصات الرئيسية
الملخص EN
The main goal of this work is to automatically segment colorectal tumors in 3D T2-weighted (T2w) MRI with reasonable accuracy.
For such a purpose, a novel deep learning-based algorithm suited for volumetric colorectal tumor segmentation is proposed.
The proposed CNN architecture, based on densely connected neural network, contains multiscale dense interconnectivity between layers of fine and coarse scales, thus leveraging multiscale contextual information in the network to get better flow of information throughout the network.
Additionally, the 3D level-set algorithm was incorporated as a postprocessing task to refine contours of the network predicted segmentation.
The method was assessed on T2-weighted 3D MRI of 43 patients diagnosed with locally advanced colorectal tumor (cT3/T4).
Cross validation was performed in 100 rounds by partitioning the dataset into 30 volumes for training and 13 for testing.
Three performance metrics were computed to assess the similarity between predicted segmentation and the ground truth (i.e., manual segmentation by an expert radiologist/oncologist), including Dice similarity coefficient (DSC), recall rate (RR), and average surface distance (ASD).
The above performance metrics were computed in terms of mean and standard deviation (mean ± standard deviation).
The DSC, RR, and ASD were 0.8406 ± 0.0191, 0.8513 ± 0.0201, and 2.6407 ± 2.7975 before postprocessing, and these performance metrics became 0.8585 ± 0.0184, 0.8719 ± 0.0195, and 2.5401 ± 2.402 after postprocessing, respectively.
We compared our proposed method to other existing volumetric medical image segmentation baseline methods (particularly 3D U-net and DenseVoxNet) in our segmentation tasks.
The experimental results reveal that the proposed method has achieved better performance in colorectal tumor segmentation in volumetric MRI than the other baseline techniques.
نمط استشهاد جمعية علماء النفس الأمريكية (APA)
Soomro, Mumtaz Hussain& Coppotelli, Matteo& Conforto, Silvia& Schmid, Maurizio& Giunta, Gaetano& Del Secco, Lorenzo…[et al.]. 2019. Automated Segmentation of Colorectal Tumor in 3D MRI Using 3D Multiscale Densely Connected Convolutional Neural Network. Journal of Healthcare Engineering،Vol. 2019, no. 2019, pp.1-11.
https://search.emarefa.net/detail/BIM-1175048
نمط استشهاد الجمعية الأمريكية للغات الحديثة (MLA)
Soomro, Mumtaz Hussain…[et al.]. Automated Segmentation of Colorectal Tumor in 3D MRI Using 3D Multiscale Densely Connected Convolutional Neural Network. Journal of Healthcare Engineering No. 2019 (2019), pp.1-11.
https://search.emarefa.net/detail/BIM-1175048
نمط استشهاد الجمعية الطبية الأمريكية (AMA)
Soomro, Mumtaz Hussain& Coppotelli, Matteo& Conforto, Silvia& Schmid, Maurizio& Giunta, Gaetano& Del Secco, Lorenzo…[et al.]. Automated Segmentation of Colorectal Tumor in 3D MRI Using 3D Multiscale Densely Connected Convolutional Neural Network. Journal of Healthcare Engineering. 2019. Vol. 2019, no. 2019, pp.1-11.
https://search.emarefa.net/detail/BIM-1175048
نوع البيانات
مقالات
لغة النص
الإنجليزية
الملاحظات
Includes bibliographical references
رقم السجل
BIM-1175048
قاعدة معامل التأثير والاستشهادات المرجعية العربي "ارسيف Arcif"
أضخم قاعدة بيانات عربية للاستشهادات المرجعية للمجلات العلمية المحكمة الصادرة في العالم العربي

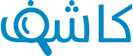
تقوم هذه الخدمة بالتحقق من التشابه أو الانتحال في الأبحاث والمقالات العلمية والأطروحات الجامعية والكتب والأبحاث باللغة العربية، وتحديد درجة التشابه أو أصالة الأعمال البحثية وحماية ملكيتها الفكرية. تعرف اكثر
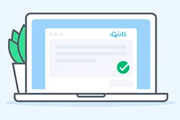