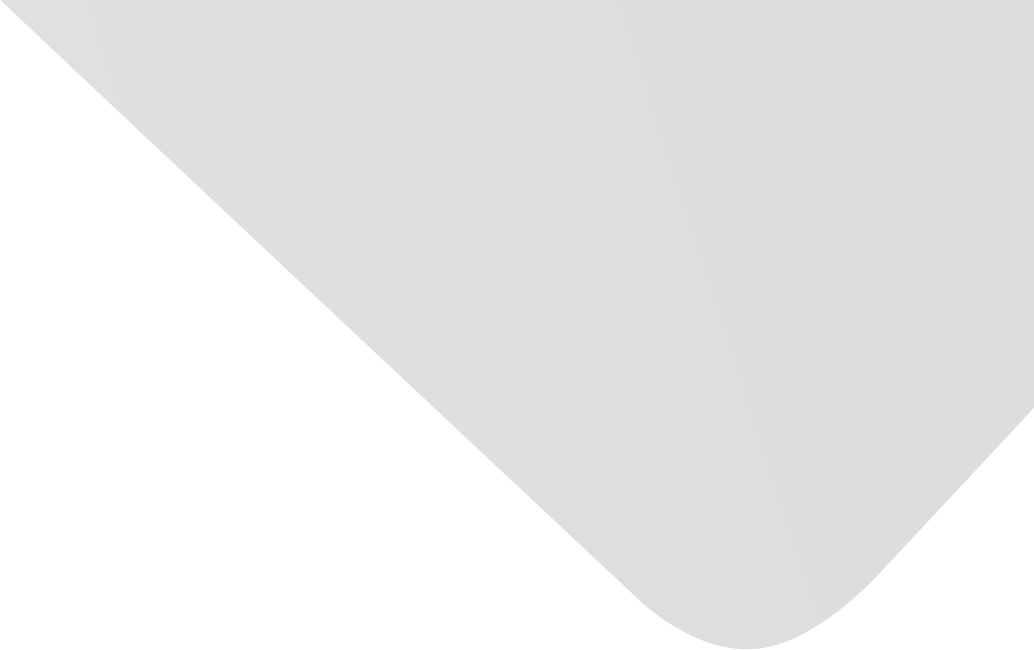
Risk Prediction for Ship Encounter Situation Awareness Using Long Short-Term Memory Based Deep Learning on Intership Behaviors
المؤلفون المشاركون
Li, Wenkai
Zhang, Chunwei
Jia, Chengfeng
Zhang, Yu
Ma, Jie
المصدر
Journal of Advanced Transportation
العدد
المجلد 2020، العدد 2020 (31 ديسمبر/كانون الأول 2020)، ص ص. 1-15، 15ص.
الناشر
Hindawi Publishing Corporation
تاريخ النشر
2020-11-29
دولة النشر
مصر
عدد الصفحات
15
التخصصات الرئيسية
الملخص EN
Encounter risk prediction is critical for safe ship navigation, especially in congested waters, where ships sail very near to each other during various encounter situations.
Prior studies on the risk of ship collisions were unable to address the uncertainty of the encounter process when ignoring the complex motions constituting the dynamic ship encounter behavior, which may seriously affect the risk prediction performance.
To fill this gap, a novel AIS data-driven approach is proposed for ship encounter risk prediction by modeling intership behavior patterns.
In particular, multidimensional features of intership behaviors are extracted from the AIS trace data to capture spatial dependencies between encountering ships.
Then, the challenging task of risk prediction is to discover the complex and uncertain relationship between intership behaviors and future collision risk.
To address this issue, we propose a deep learning framework.
To represent the temporal dynamics of the encounter process, we use the sliding window technique to generate the sequences of behavioral features.
The collision risk level at a future time is taken as the class label of the sequence.
Then, the long short-term memory network, which has a strong ability to model temporal dependency and complex patterns, is extended to establish the relationship.
The benefit of our approach is that it transforms the complex problem for risk prediction into a time series classification task, which makes collision risk prediction reliable and easier to implement.
Experiments were conducted on a set of naturalistic data from various encounter scenarios in the South Channel of the Yangtze River Estuary.
The results show that the proposed data-driven approach can predict future collision risk with high accuracy and efficiency.
The approach is expected to be applied for the early prediction of encountering ships and as decision support to improve navigation safety.
نمط استشهاد جمعية علماء النفس الأمريكية (APA)
Ma, Jie& Li, Wenkai& Jia, Chengfeng& Zhang, Chunwei& Zhang, Yu. 2020. Risk Prediction for Ship Encounter Situation Awareness Using Long Short-Term Memory Based Deep Learning on Intership Behaviors. Journal of Advanced Transportation،Vol. 2020, no. 2020, pp.1-15.
https://search.emarefa.net/detail/BIM-1180995
نمط استشهاد الجمعية الأمريكية للغات الحديثة (MLA)
Ma, Jie…[et al.]. Risk Prediction for Ship Encounter Situation Awareness Using Long Short-Term Memory Based Deep Learning on Intership Behaviors. Journal of Advanced Transportation No. 2020 (2020), pp.1-15.
https://search.emarefa.net/detail/BIM-1180995
نمط استشهاد الجمعية الطبية الأمريكية (AMA)
Ma, Jie& Li, Wenkai& Jia, Chengfeng& Zhang, Chunwei& Zhang, Yu. Risk Prediction for Ship Encounter Situation Awareness Using Long Short-Term Memory Based Deep Learning on Intership Behaviors. Journal of Advanced Transportation. 2020. Vol. 2020, no. 2020, pp.1-15.
https://search.emarefa.net/detail/BIM-1180995
نوع البيانات
مقالات
لغة النص
الإنجليزية
الملاحظات
Includes bibliographical references
رقم السجل
BIM-1180995
قاعدة معامل التأثير والاستشهادات المرجعية العربي "ارسيف Arcif"
أضخم قاعدة بيانات عربية للاستشهادات المرجعية للمجلات العلمية المحكمة الصادرة في العالم العربي
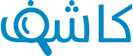
تقوم هذه الخدمة بالتحقق من التشابه أو الانتحال في الأبحاث والمقالات العلمية والأطروحات الجامعية والكتب والأبحاث باللغة العربية، وتحديد درجة التشابه أو أصالة الأعمال البحثية وحماية ملكيتها الفكرية. تعرف اكثر
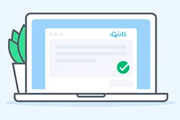